Deep Learning-Based Feature Extraction in Iris Recognition: Use Existing Models, Fine-tune or Train From Scratch?
2019 IEEE 10th International Conference on Biometrics Theory, Applications and Systems (BTAS)(2019)
摘要
Modern deep learning techniques can be employed to generate effective feature extractors for the task of iris recognition. The question arises: should we train such structures from scratch on a relatively large iris image dataset, or it is better to fine-tune the existing models to adapt them to a new domain? In this work we explore five different sets of weights for the popular ResNet-50 architecture to find out whether iris-specific feature extractors perform better than models trained for non-iris tasks. Features are extracted from each convolutional layer and the classification accuracy achieved by a Support Vector Machine is measured on a dataset that is disjoint from the samples used in training of the ResNet-50 model. We show that the optimal training strategy is to fine-tune an off-the-shelf set of weights to the iris recognition domain. This approach results in greater accuracy than both off-the-shelf weights and a model trained from scratch. The winning, fine-tuned approach also shows an increase in performance when compared to previous work, in which only off-the-shelf (not fine-tuned) models were used in iris feature extraction. We make the best-performing ResNet-50 model, fine-tuned with more than 360,000 iris images, publicly available along with this paper.
更多查看译文
关键词
fine-tuned approach,off-the-shelf models,not fine-tuned,iris feature extraction,ResNet-50 model,use existing models,modern deep learning techniques,effective feature extractors,relatively large iris image dataset,popular ResNet-50 architecture,iris-specific feature extractors,noniris tasks,classification accuracy,Support Vector Machine,optimal training strategy,iris recognition domain,off-the-shelf weights,winning approach
AI 理解论文
溯源树
样例
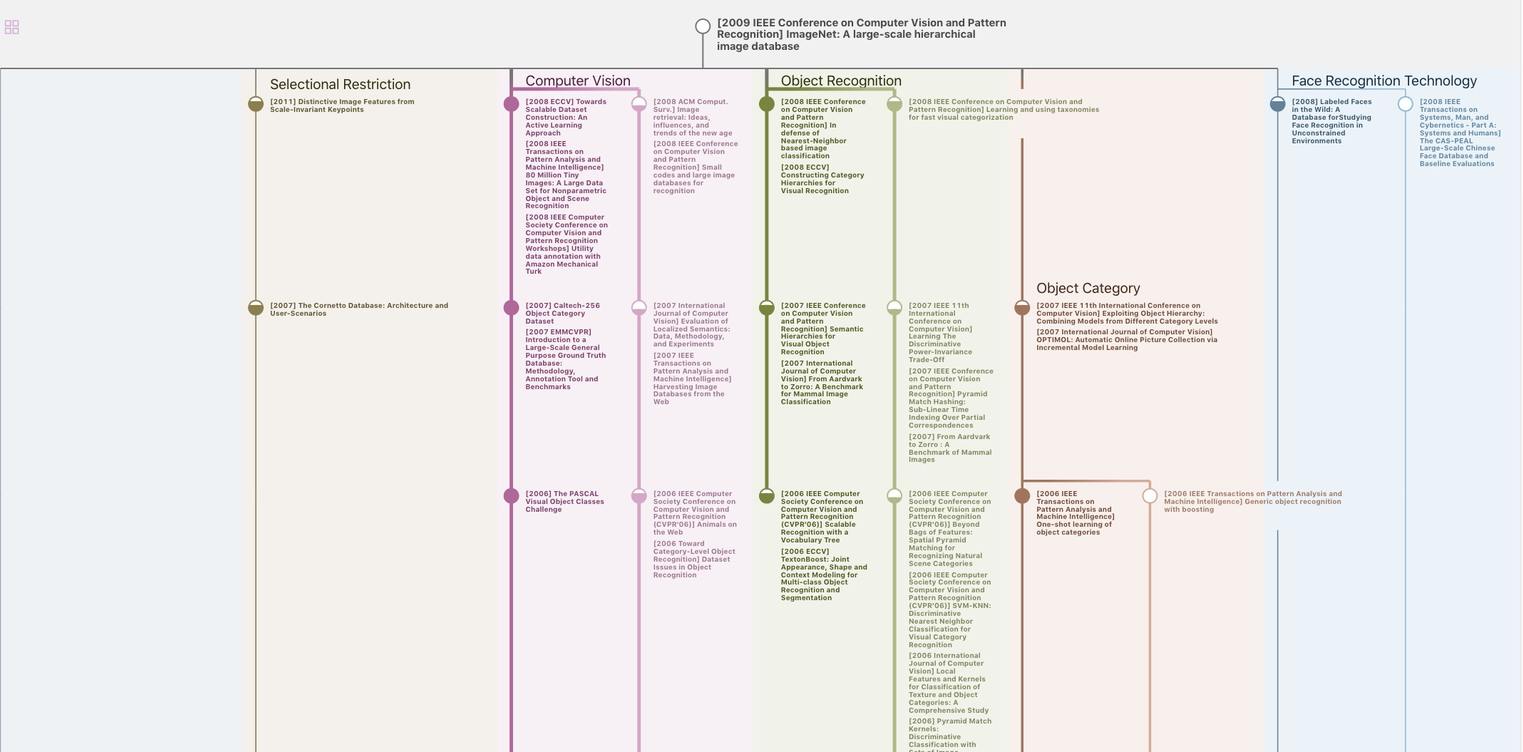
生成溯源树,研究论文发展脉络
Chat Paper
正在生成论文摘要