Anomaly Detection, Consider Your Dataset First An Illustration On Fraud Detection
2019 IEEE 31ST INTERNATIONAL CONFERENCE ON TOOLS WITH ARTIFICIAL INTELLIGENCE (ICTAI 2019)(2019)
摘要
Anomaly detection is a research area where machine learning can have a significant impact. Many efforts have been made in this area for years and conference proceedings are full of outstanding work presenting algorithms that are all more efficient than each other. Nevertheless, this paper states that it is just as important to consider in depth the datasets on which we will work later as it is to develop high-performance algorithms. Thus, in this paper, we present the context of a real case of anomaly detection and what work needs to be done on the dataset well before considering the development of new algorithms to process it. We expect that this work and various general references provided can be useful for many other researchers who will have to work on real datasets and not only on datasets from famous repositories that have already been pre-processed and for which we know all the characteristics.
更多查看译文
关键词
Anomaly detection, fraud detection, imbalanced dataset, class overlapping, concept drift, feature engineering
AI 理解论文
溯源树
样例
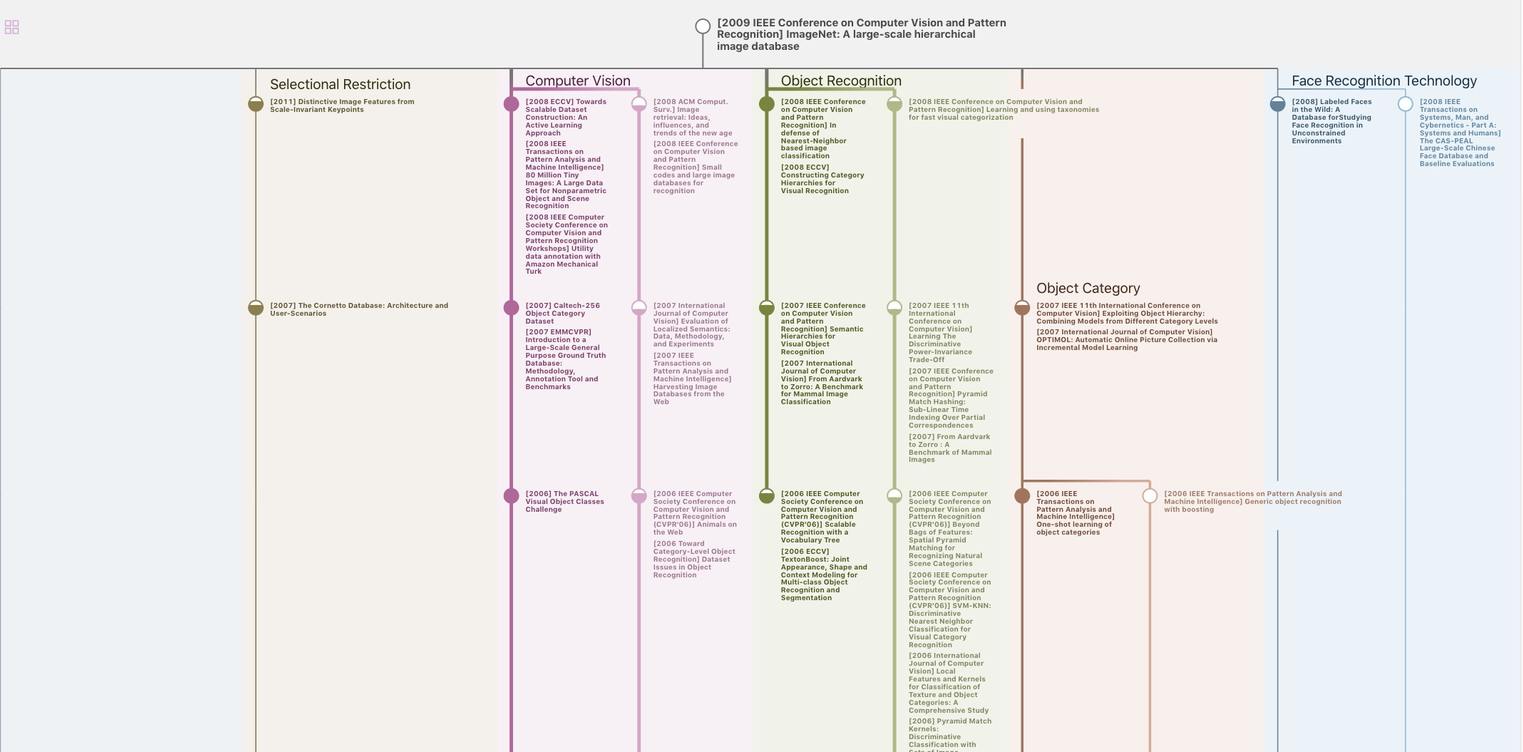
生成溯源树,研究论文发展脉络
Chat Paper
正在生成论文摘要