Texture Recognition on Metal Surface using Order-Less Scale Invariant GLAC
2019 IEEE 31st International Conference on Tools with Artificial Intelligence (ICTAI)(2019)
摘要
Inspection of metal surface textures using computer vision and machine learning techniques plays an important role in Automated Visual Inspection (AVI) systems. Texture recognition on metal surface is challenging because the characteristics of each texture type are dependent on the properties of the metal surface when captured under different lighting conditions. Since these textures have no obvious repetitive patterns like general textures, this results in high intra-class diversities. Prior knowledge has shown that surface properties such as surface curvature and depth are discriminant to different texture types on metal surface. Since scale, shapes and location of textures within the same type are not fixed, scale property and spatial ordering information are less important for differentiating between texture types. There-fore, surface property, scale invariance and order-less property should be considered when exploring a suitable image feature for metal surface texture recognition. This paper proposes Order-less Scale Invariant Gradient Local Auto-Correlation (OS-GLAC) which meets all three requirements for robust texture recognition. The experiment results show that OS-GLAC is robust to separate different metal surface texture types. In addition, we observed that OS-GLAC is not only useful for texture recognition on metal surface but also for general texture recognition when combined with pre-trained deep learning features as these two features capture complimentary information. The experiment results show that such a combination of OS-GLAC achieves competitive results on three well-established general texture datasets i.e., KTH-TIP-2a, KTH-TIPS-2b and FMD.
更多查看译文
关键词
Metal surface texture classification, Gradient Local Auto-Correlation (GLAC), Order-less Encoding method, Scale invariant, Convolutional Neural Network (CNN), KTH-TIPS-2a, KTH TIPS-2b FMD
AI 理解论文
溯源树
样例
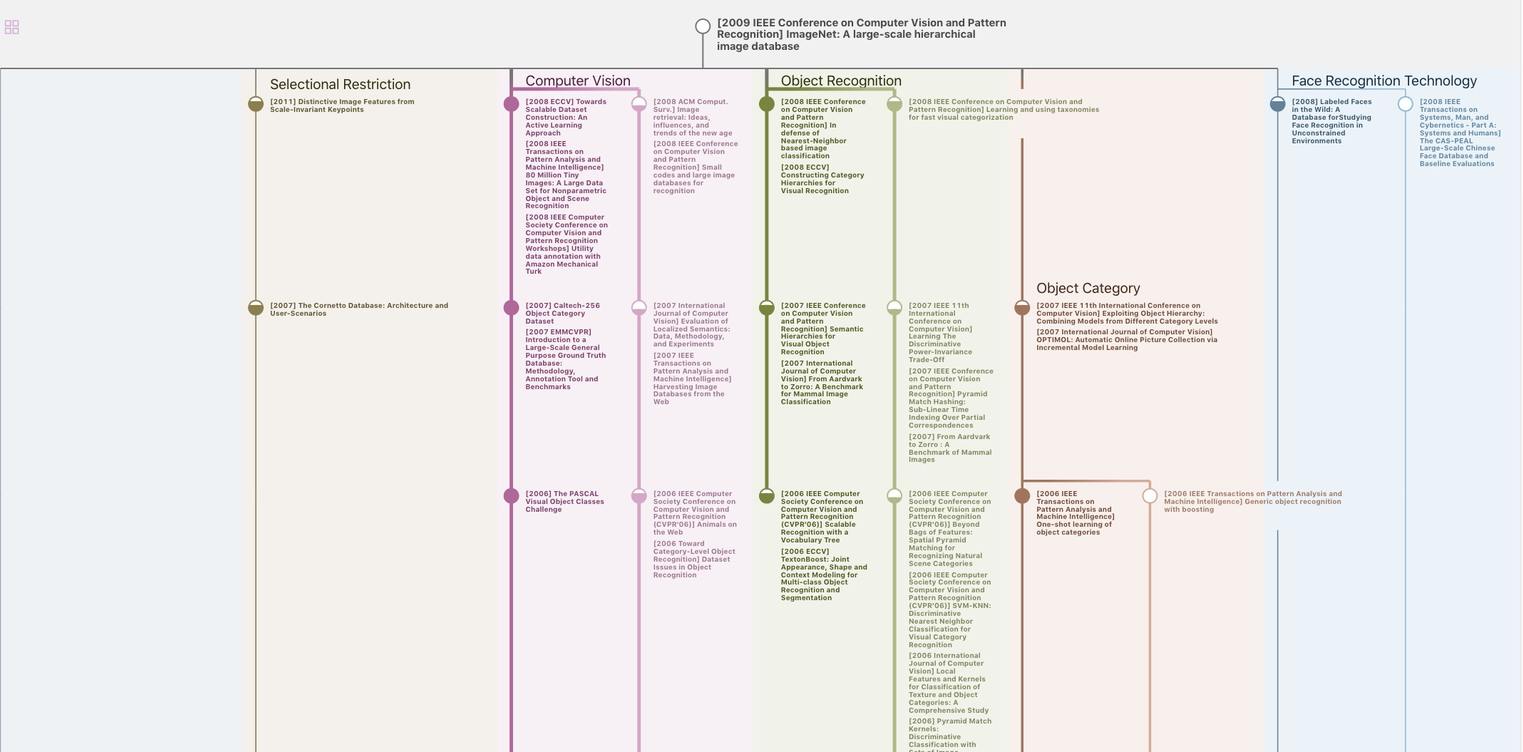
生成溯源树,研究论文发展脉络
Chat Paper
正在生成论文摘要