The Concept of Criticality in Reinforcement Learning
2019 IEEE 31st International Conference on Tools with Artificial Intelligence (ICTAI)(2019)
摘要
This paper introduces a novel idea in human-aided reinforcement learning - the concept of criticality. The criticality of a state indicates how much the choice of action in that particular state influences the expected return. In order to develop an intuition for the concept, we present examples of plausible criticality functions in multiple environments. Furthermore, we formulate a practical application of criticality in reinforcement learning: the criticality-based varying stepnumber algorithm (CVS) - a flexible stepnumber algorithm that utilizes the criticality function, provided by a human, in order to avoid the problem of choosing an appropriate stepnumber in n-step algorithms such as n-step SARSA and n-step Tree Backup. We present experiments in the Atari Pong environment demonstrating that CVS is able to outperform popular learning algorithms such as Deep Q-Learning and Monte Carlo.
更多查看译文
关键词
Human aided reinforcement learning Human agent interaction
AI 理解论文
溯源树
样例
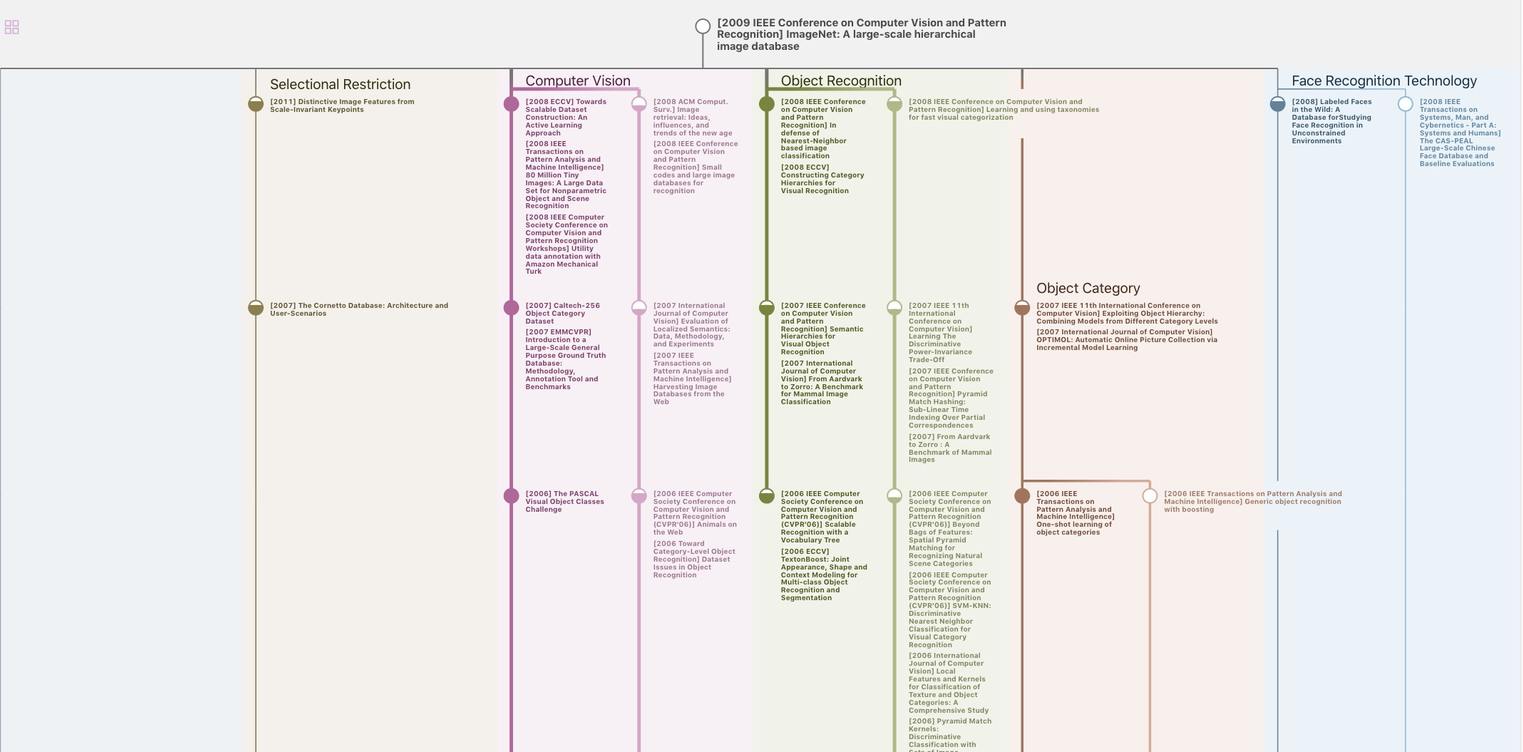
生成溯源树,研究论文发展脉络
Chat Paper
正在生成论文摘要