Attribute Reduction For Partially Labeled Data Based On Hypergraph Models
2019 IEEE 31ST INTERNATIONAL CONFERENCE ON TOOLS WITH ARTIFICIAL INTELLIGENCE (ICTAI 2019)(2019)
摘要
Attribute reduction is an important process in many fields, such as knowledge discovery, data mining, machine learning, pattern recognition and so on. However, totally labeled data are quite hard to obtain in real life. Thus, we often have to face the situation that not all the data have been associated with labels in advance. Due to the coexistence of labeled and unlabeled data, attribute reduction problem for partially labeled data becomes more complex and challenging. Many scholars have devoted themselves to solve this problem in the past few years. But current algorithms for partially labeled data are not efficient enough in terms of time complexity. To address this issue, we propose a hypergraph model, where two types of induced hypergraphs are designed from partially labeled decision systems. Then, a fast algorithm based on low-complexity heuristics is raised to compute the minimum vertex cover of a hypergraph. Finally, we propose two types of hypergraph models-based attribute reduction algorithms for partially labeled decision systems. Experimental results on broadly used data sets show that the feasibility and efficiency of our proposed algorithms.
更多查看译文
关键词
attribute reduction, hypergraph, partially labeled decision system, minimum vertex cover
AI 理解论文
溯源树
样例
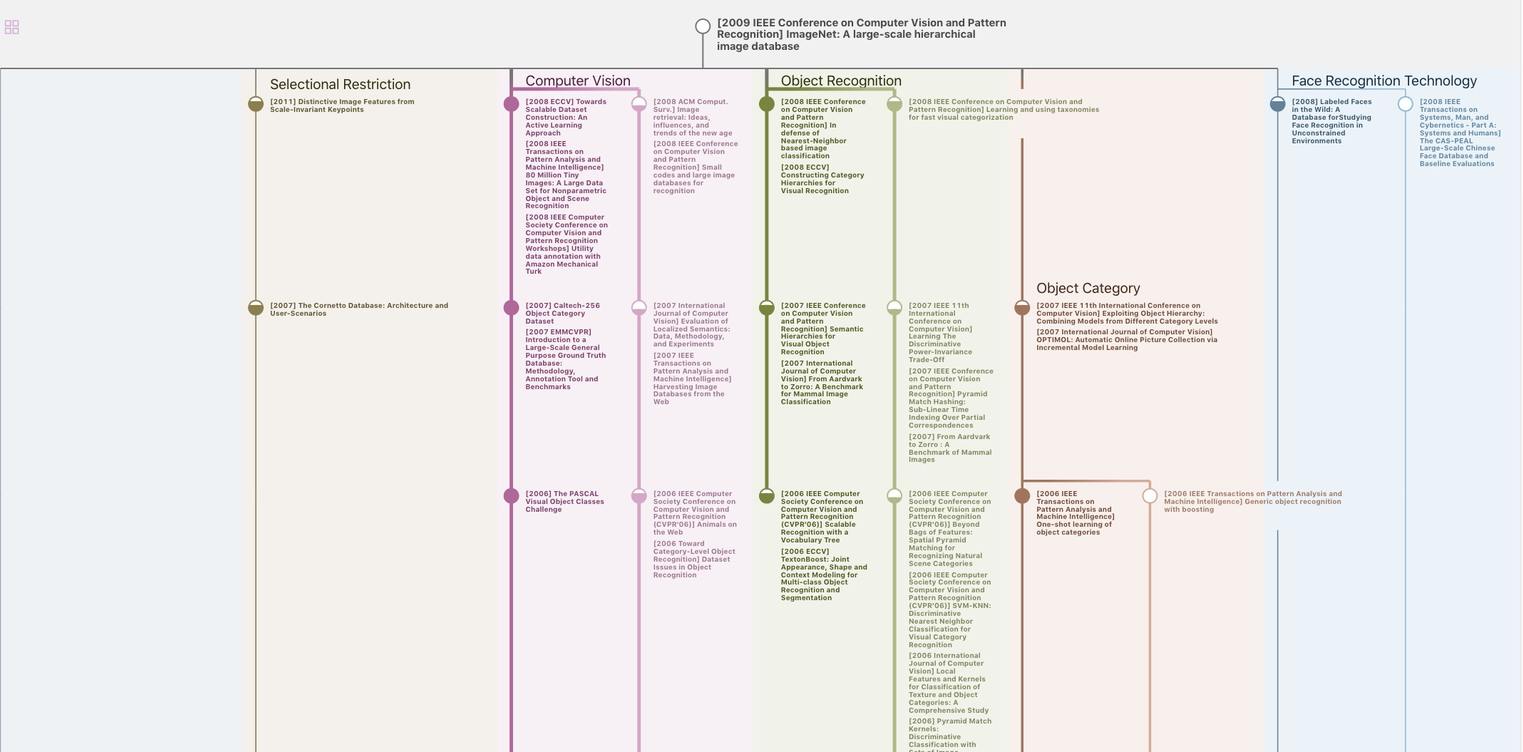
生成溯源树,研究论文发展脉络
Chat Paper
正在生成论文摘要