Regression Models For Performance Ranking Of Configurable Systems: A Comparative Study
STRUCTURED OBJECT-ORIENTED FORMAL LANGUAGE AND METHOD (SOFL+MSVL 2019)(2020)
摘要
Finding the best configurations for a highly configurable system is challenging. Existing studies learned regression models to predict the performance of potential configurations. Such learning suffers from the low accuracy and the high effort of examining the actual performance for data labeling. A recent approach uses an iterative strategy to sample a small number of configurations from the training pool to reduce the number of sampled ones. In this paper, we conducted a comparative study on the rank-based approach of configurable systems with four regression methods. These methods are compared on 21 evaluation scenarios of 16 real-world configurable systems. We designed three research questions to check the impacts of different methods on the rank-based approach. We find out that the decision tree method of Classification And Regression Tree (CART) and the ensemble learning method of Gradient Boosted Regression Trees (GBRT) can achieve better ranks among four regression methods under evaluation; the sampling strategy in the rank-based approach is useful to save the cost of sampling configurations; the measurement, i.e., rank difference correlates with the relative error in several evaluation scenarios.
更多查看译文
关键词
Regression methods, Performance prediction, Sampling, Software configurations
AI 理解论文
溯源树
样例
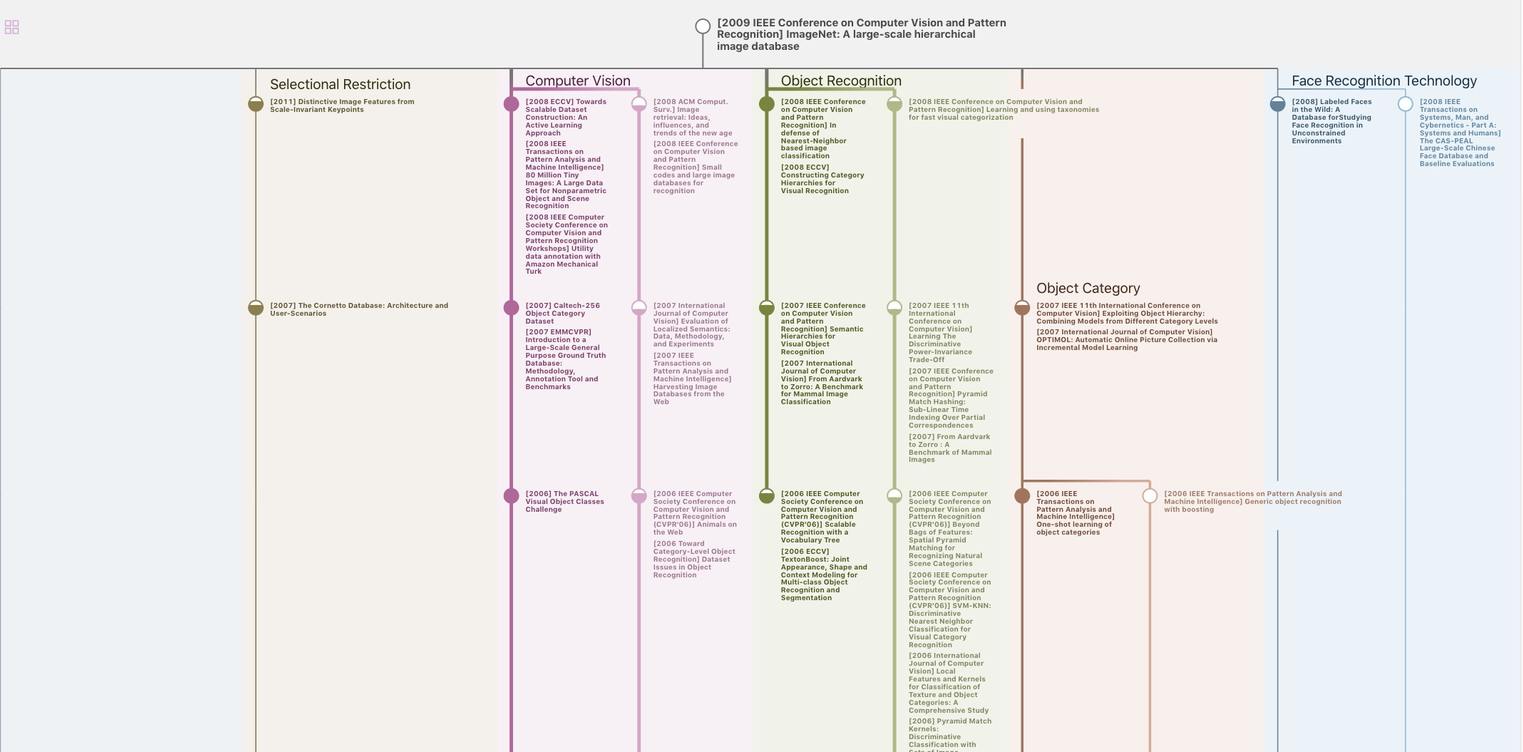
生成溯源树,研究论文发展脉络
Chat Paper
正在生成论文摘要