Emotion Classification Based on Biophysical Signals and Machine Learning Techniques.
SYMMETRY-BASEL(2020)
摘要
Emotions constitute an indispensable component of our everyday life. They consist of conscious mental reactions towards objects or situations and are associated with various physiological, behavioral, and cognitive changes. In this paper, we propose a comparative analysis between different machine learning and deep learning techniques, with and without feature selection, for binarily classifying the six basic emotions, namely anger, disgust, fear, joy, sadness, and surprise, into two symmetrical categorical classes (emotion and no emotion), using the physiological recordings and subjective ratings of valence, arousal, and dominance from the DEAP (Dataset for Emotion Analysis using EEG, Physiological and Video Signals) database. The results showed that the maximum classification accuracies for each emotion were: anger: 98.02%, joy:100%, surprise: 96%, disgust: 95%, fear: 90.75%, and sadness: 90.08%. In the case of four emotions (anger, disgust, fear, and sadness), the classification accuracies were higher without feature selection. Our approach to emotion classification has future applicability in the field of affective computing, which includes all the methods used for the automatic assessment of emotions and their applications in healthcare, education, marketing, website personalization, recommender systems, video games, and social media.
更多查看译文
关键词
emotion classification,machine learning,feature selection,affective computing,biophysical sensors
AI 理解论文
溯源树
样例
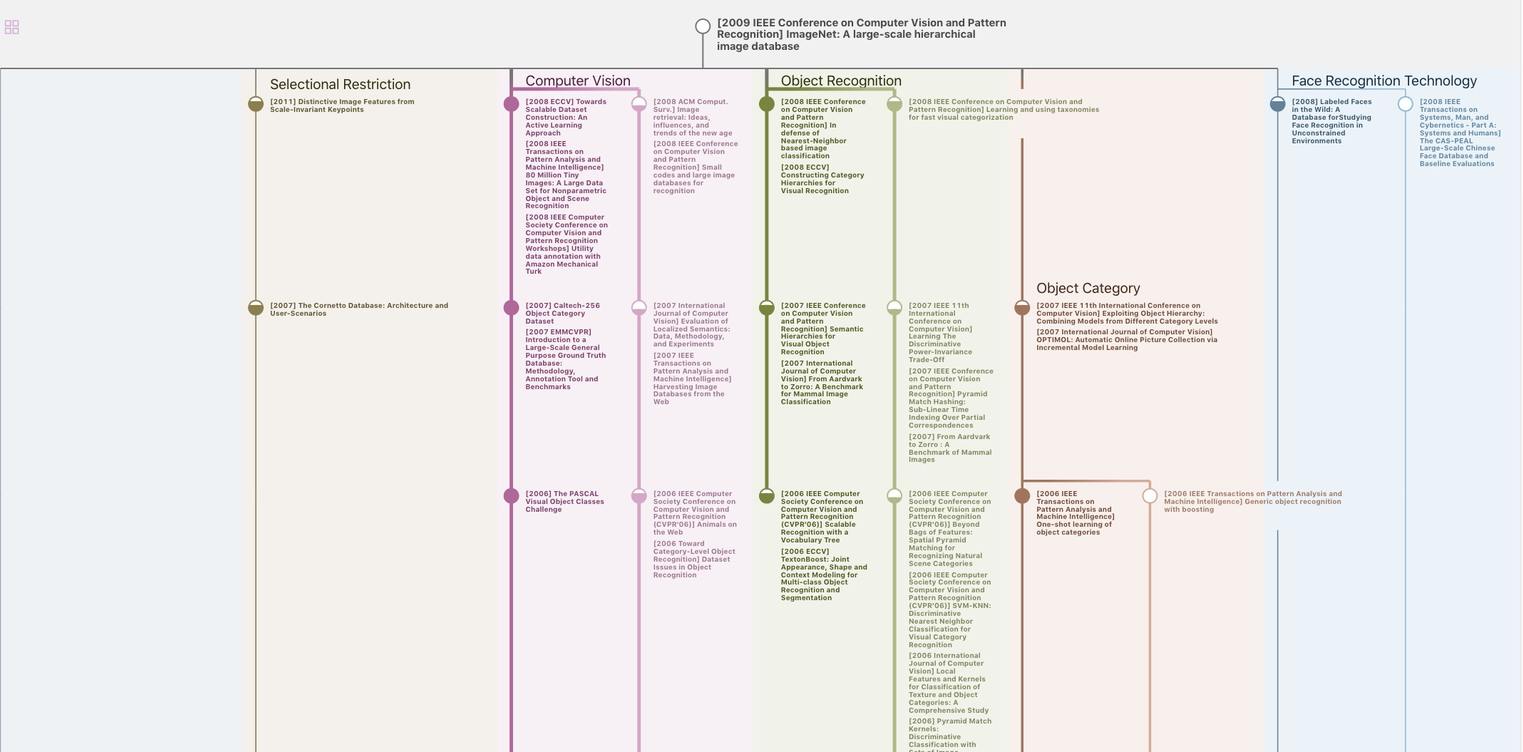
生成溯源树,研究论文发展脉络
Chat Paper
正在生成论文摘要