Causal Structure Learning from Time Series: Large Regression Coefficients May Predict Causal Links Better in Practice Than Small P-Values.
Neural Information Processing Systems(2020)
Abstract
In this article, we describe the algorithms for causal structure learning from time series data that won the Causality 4 Climate competition at the Conference on Neural Information Processing Systems 2019 (NeurIPS). We examine how our combination of established ideas achieves competitive performance on semi-realistic and realistic time series data exhibiting common challenges in real-world Earth sciences data. In particular, we discuss a) a rationale for leveraging linear methods to identify causal links in non-linear systems, b) a simulation-backed explanation as to why large regression coefficients may predict causal links better in practice than small p-values and thus why normalising the data may sometimes hinder causal structure learning. For benchmark usage, we detail the algorithms here and provide implementations at this https URL . We propose the presented competition-proven methods for baseline benchmark comparisons to guide the development of novel algorithms for structure learning from time series.
MoreTranslated text
Key words
Structure Learning,Causal Inference,Relational Data Modeling,Causal Discovery,Probabilistic Learning
AI Read Science
Must-Reading Tree
Example
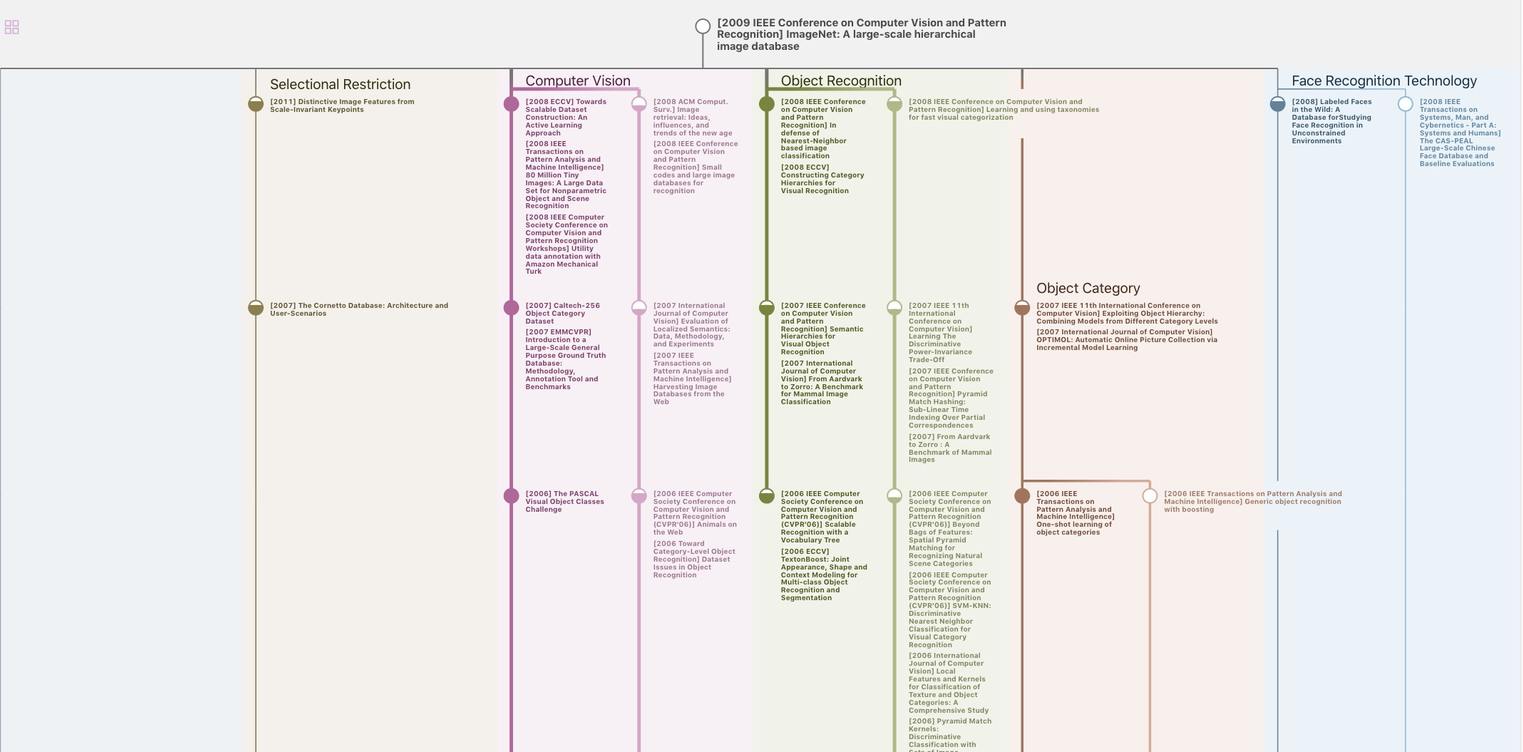
Generate MRT to find the research sequence of this paper
Chat Paper
Summary is being generated by the instructions you defined