Stochasticity in Neural ODEs: An Empirical Study
international conference on learning representations
摘要
Stochastic regularization of neural networks (e.g. dropout) is a wide-spread technique in deep learning that allows for better generalization. Despite its success, continuous-time models, such as neural ordinary differential equation (ODE), usually rely on a completely deterministic feed-forward operation. This work provides an empirical study of stochastically regularized neural ODE on several image-classification tasks (CIFAR-10, CIFAR-100, TinyImageNet). Building upon the formalism of stochastic differential equations (SDEs), we demonstrate that neural SDE is able to outperform its deterministic counterpart. Further, we show that data augmentation during the training improves the performance of both deterministic and stochastic versions of the same model. However, the improvements obtained by the data augmentation completely eliminate the empirical gains of the stochastic regularization, making the difference in the performance of neural ODE and neural SDE negligible.
更多查看译文
关键词
neural odes,stochasticity,empirical study
AI 理解论文
溯源树
样例
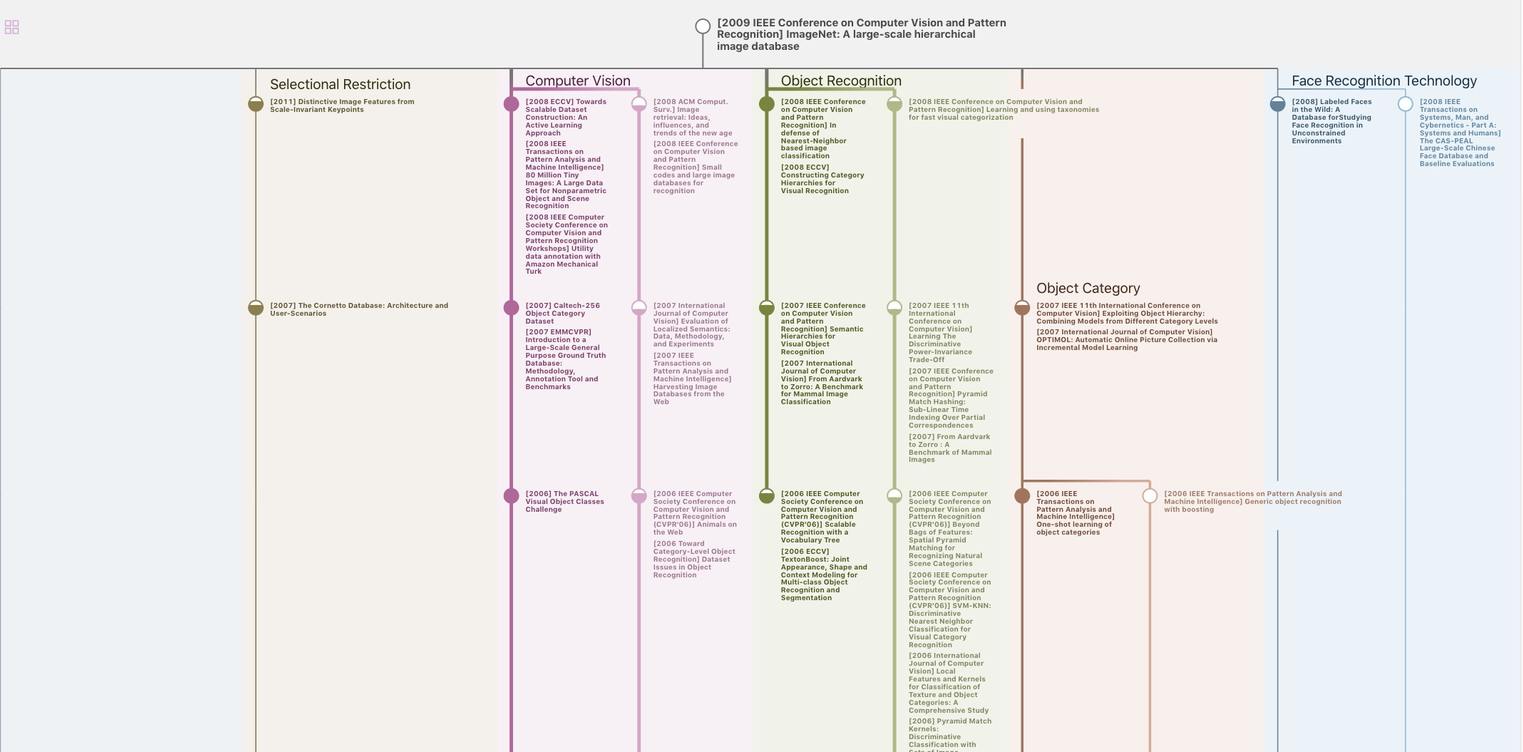
生成溯源树,研究论文发展脉络
Chat Paper
正在生成论文摘要