Driving with Data in the Motor City: Mining and Modeling Vehicle Fleet Maintenance Data
arxiv(2020)
摘要
The City of Detroit maintains an active fleet of over 2500 vehicles, spending an annual average of over \$5 million on purchases and over \$7.7 million on maintenance. Modeling patterns and trends in this data is of particular importance to a variety of stakeholders, particularly as Detroit emerges from Chapter 9 bankruptcy, but the structure in such data is complex, and the city lacks dedicated resources for in-depth analysis. The City of Detroit's Operations and Infrastructure Group and the University of Michigan initiated a collaboration which seeks to address this unmet need by analyzing data from the City of Detroit's vehicle fleet. This work presents a case study and provides the first data-driven benchmark, demonstrating a suite of methods to aid in data understanding and prediction for large vehicle maintenance datasets. We present analyses to address three key questions raised by the stakeholders, related to discovering multivariate maintenance patterns over time; predicting maintenance; and predicting vehicle- and fleet-level costs. We present a novel algorithm, PRISM, for automating multivariate sequential data analyses using tensor decomposition. This work is a first of its kind that presents both methodologies and insights to guide future civic data research.
更多查看译文
关键词
modeling vehicle fleet,motor city,maintenance,data
AI 理解论文
溯源树
样例
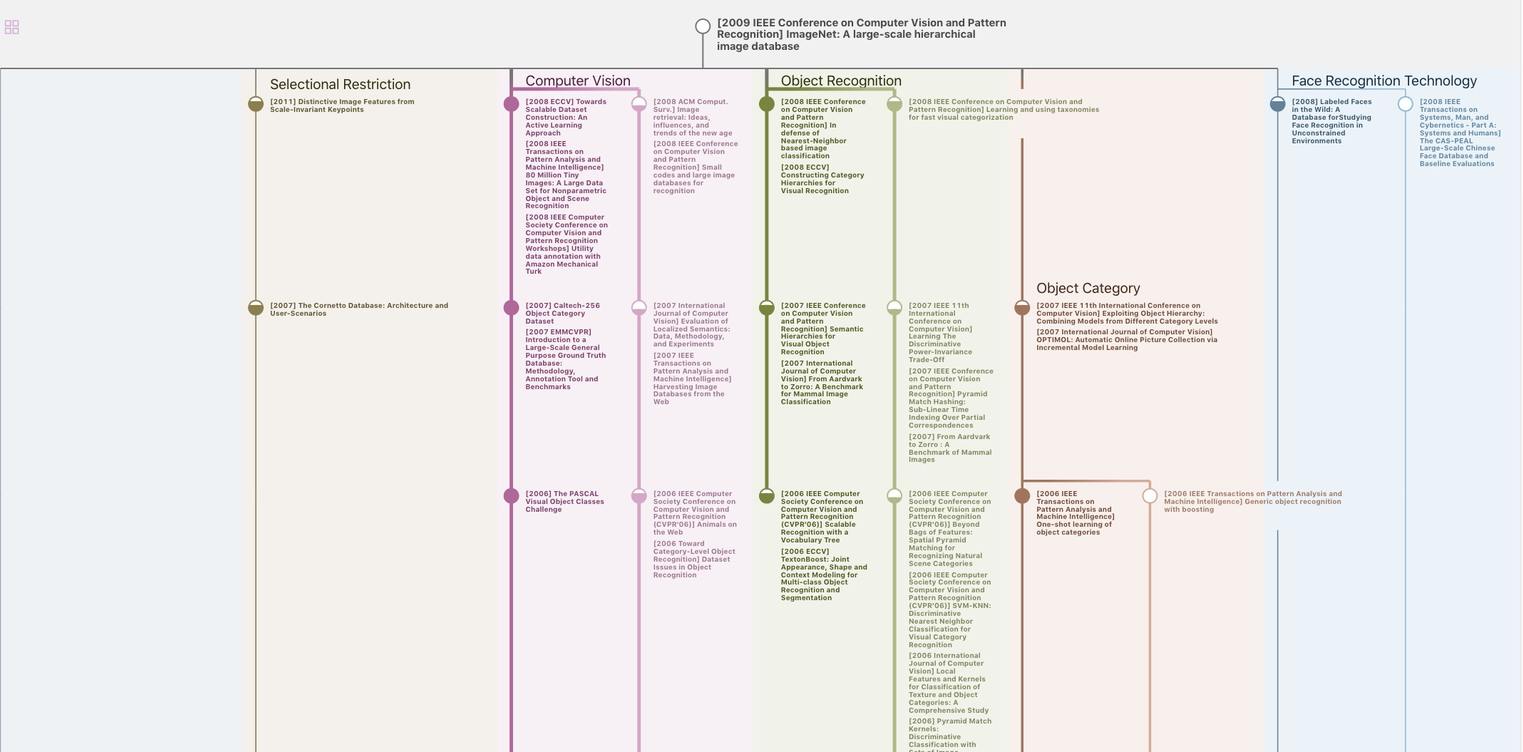
生成溯源树,研究论文发展脉络
Chat Paper
正在生成论文摘要