Semi-Supervised end-to-end Speech Recognition via Local Prior Matching
arxiv(2021)
摘要
For sequence transduction tasks like speech recognition, a strong structured prior model encodes rich information about the target space, implicitly ruling out invalid sequences by assigning them low probability. In this work, we propose local prior matching (LPM), a semi-supervised objective that distills knowledge from a strong prior (e.g. a language model) to provide learning signal to an end-to-end model trained on unlabeled speech. We demonstrate that LPM is simple to implement and superior to existing knowledge distillation techniques under comparable settings. Starting from a baseline trained on 100 hours of labeled speech, with an additional 360 hours of unlabeled data, LPM recovers 54%/82% and 73%/91% of the word error rate on clean and noisy test sets with/without language model rescoring relative to a fully supervised model on the same data.
更多查看译文
关键词
Semi-supervised ASR,knowledge distillation
AI 理解论文
溯源树
样例
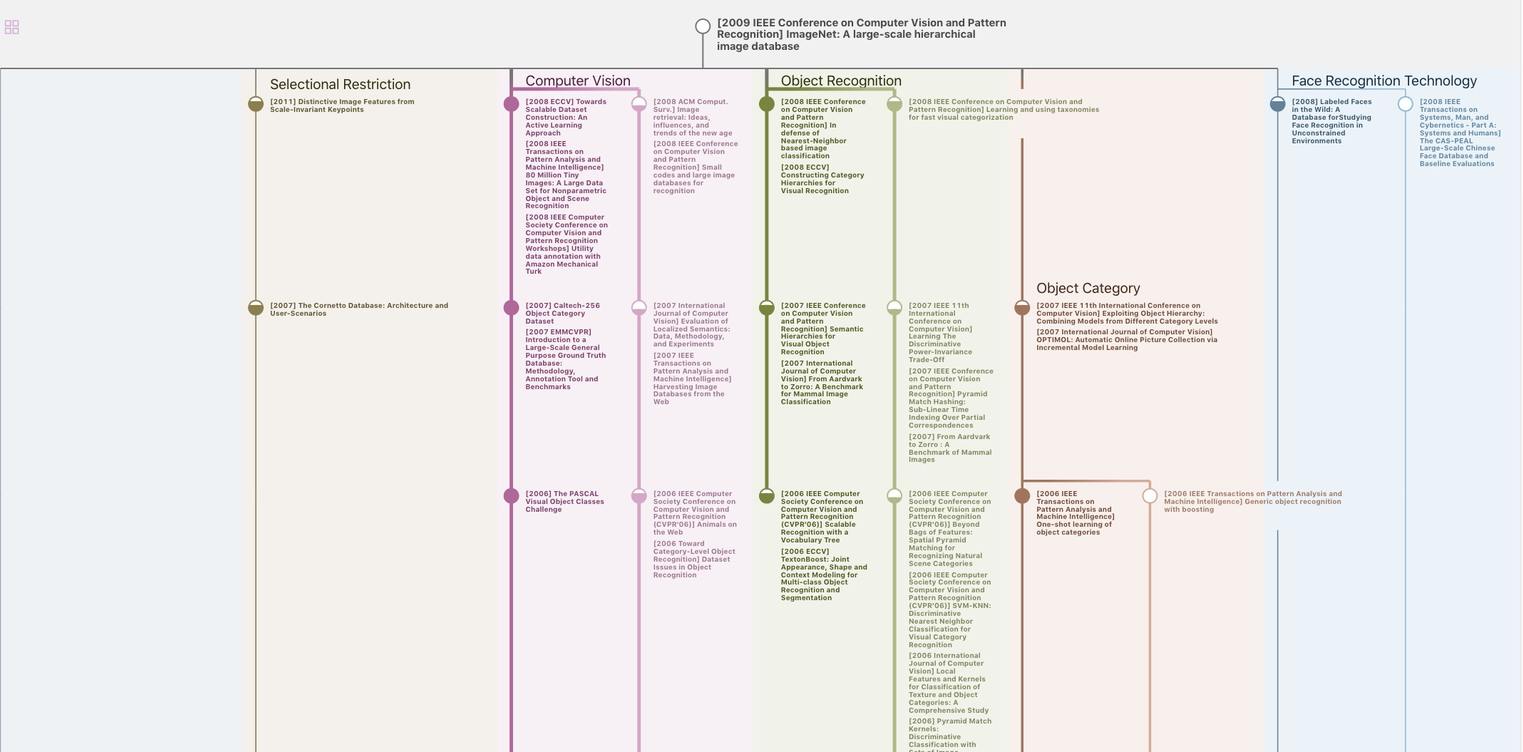
生成溯源树,研究论文发展脉络
Chat Paper
正在生成论文摘要