An Efficient Supervised Learning Method to Predict Power Supply Noise During At-speed Test
2019 IEEE International Test Conference (ITC)(2019)
摘要
The Power Distribution Network (PDN) is designed for worst-case power-hungry functional use-cases. Most often Design for Test (DFT) scenarios are not accounted for, while optimizing the PDN design. Automatic Test Pattern Generation (ATPG) tools typically follow a greedy algorithm to achieve maximum fault coverage with short test times. This causes Power Supply Noise (PSN) during scan testing to be much higher than functional mode since switching activity is higher by an order of magnitude. Understanding the noise characteristics through exhaustive pattern simulation is extremely machine and memory intensive and requires unsustainably long runtimes. Hence, we aggressively limit switching factors to conservative estimates and rely on post-silicon noise characterization to optimize test vectors. In this work, we propose a novel method to predict simultaneous switching noise using fast Deep Neural Networks (DNNs) such as Fully Connected Network, Convolutional Neural Network, and Natural Language Processing. Our approach, that is based on pre-silicon ATPG vectors, is significantly faster than conventional estimation methods and can potentially reduce the test time.
更多查看译文
关键词
Deep Learning,At-speed Capture,Simultaneous Switching Noise,Artificial Intelligence,IR Drop Prediction
AI 理解论文
溯源树
样例
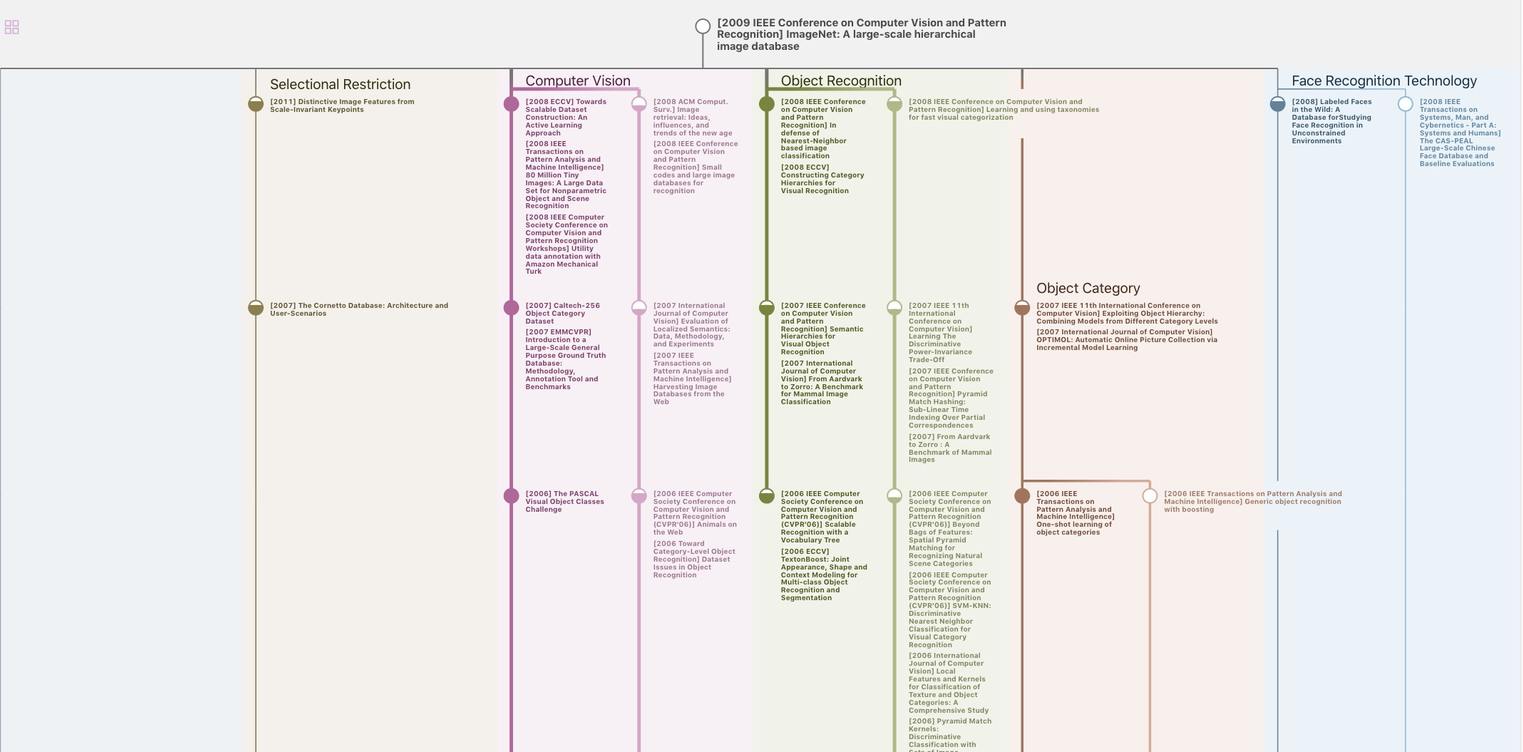
生成溯源树,研究论文发展脉络
Chat Paper
正在生成论文摘要