Asynchronous Multitask Reinforcement Learning with Dropout for Continuous Control
2019 18th IEEE International Conference On Machine Learning And Applications (ICMLA)(2019)
摘要
Deep reinforcement learning is sample inefficient for solving complex tasks. Recently, multitask reinforcement learning has received increased attention because of its ability to learn general policies with improved sample efficiency. In multitask reinforcement learning, a single agent must learn multiple related tasks, either sequentially or simultaneously. Based on the DDPG algorithm, this paper presents Asyn-DDPG, which asynchronously learns a multitask policy for continuous control with simultaneous worker agents. We empirically found that sparse policy gradients can significantly reduce interference among conflicting tasks and make multitask learning more stable and sample efficient. To ensure the sparsity of gradients evaluated for each task, Asyn-DDPG represents both actor and critic functions as deep neural networks and regularizes them using Dropout. During training, worker agents share the actor and the critic functions, and asynchronously optimize them using task-specific gradients. For evaluating Asyn-DDPG, we proposed robotic navigation tasks based on realistically simulated robots and physics-enabled maze-like environments. Although the number of tasks used in our experiment is small, each task is conducted based on a real-world setting and posts a challenging environment. Through extensive evaluation, we demonstrate that Dropout regularization can effectively stabilize asynchronous learning and enable Asyn-DDPG to outperform DDPG significantly. Also, Asyn-DDPG was able to learn a multitask policy that can be well generalized for handling environments unseen during training.
更多查看译文
关键词
Deep reinforcement learning, Multitask reinforcement learning, Asynchronous method, Continuous control, Partial observability
AI 理解论文
溯源树
样例
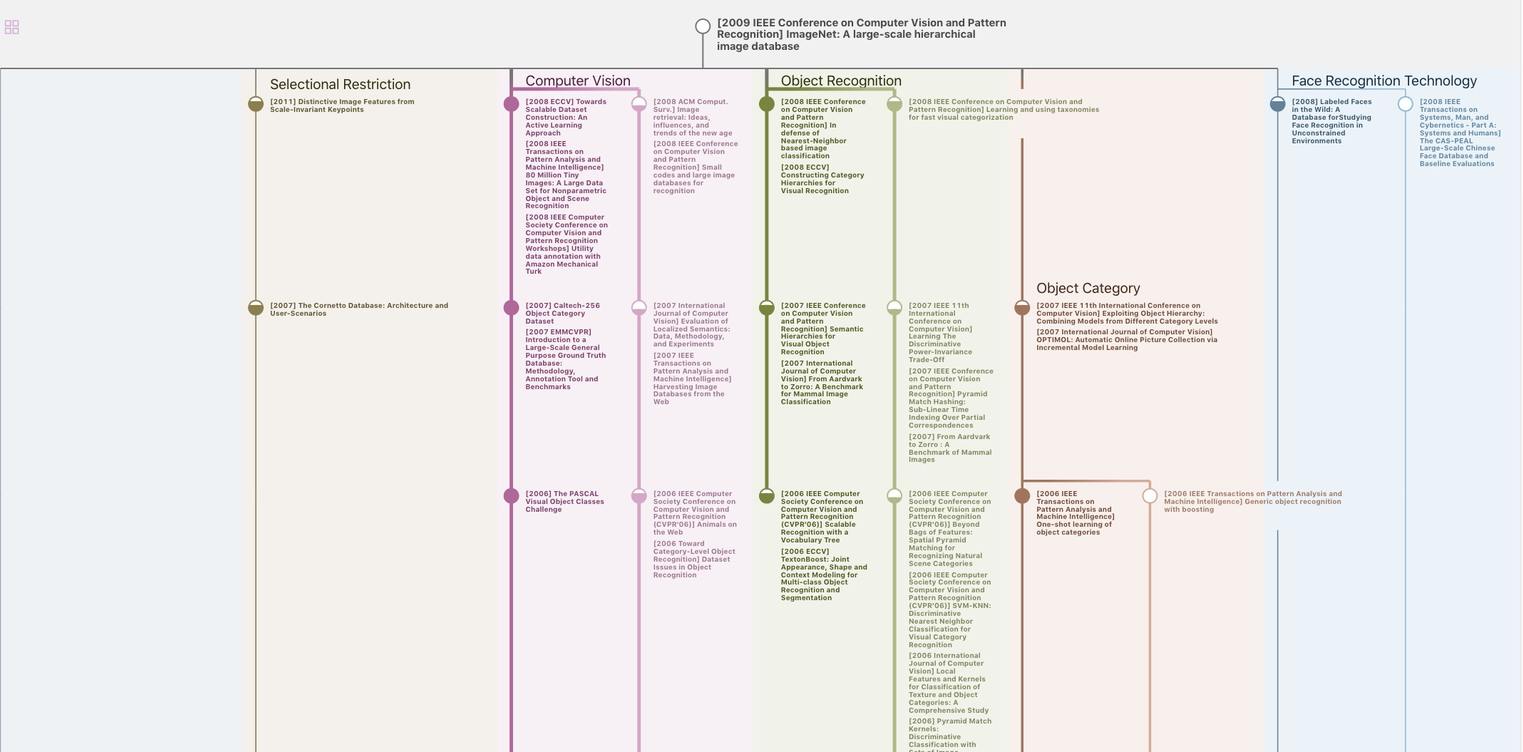
生成溯源树,研究论文发展脉络
Chat Paper
正在生成论文摘要