Learning Hierarchical Representations for Expressive Speaking Style in End-to-End Speech Synthesis
2019 IEEE Automatic Speech Recognition and Understanding Workshop (ASRU)(2019)
摘要
Although Global Style Tokens (GSTs) are a recently-proposed method to uncover expressive factors of variation in speaking style, they are a mixture of style attributes without explicitly considering the factorization of multiple-level speaking styles. In this work, we introduce a hierarchical GST architecture with residuals to Tacotron, which learns multiple-level disentangled representations to model and control different style granularities in synthesized speech. We make hierarchical evaluations conditioned on individual tokens from different GST layers. As the number of layers increases, we tend to observe a coarse to fine style decomposition. For example, the first GST layer learns a good representation of speaker IDs while finer speaking style or emotion variations can be found in higher-level layers. Meanwhile, the proposed model shows good performance of style transfer.
更多查看译文
关键词
Speaking style,disentangled representations,hierarchical GST,style transfer
AI 理解论文
溯源树
样例
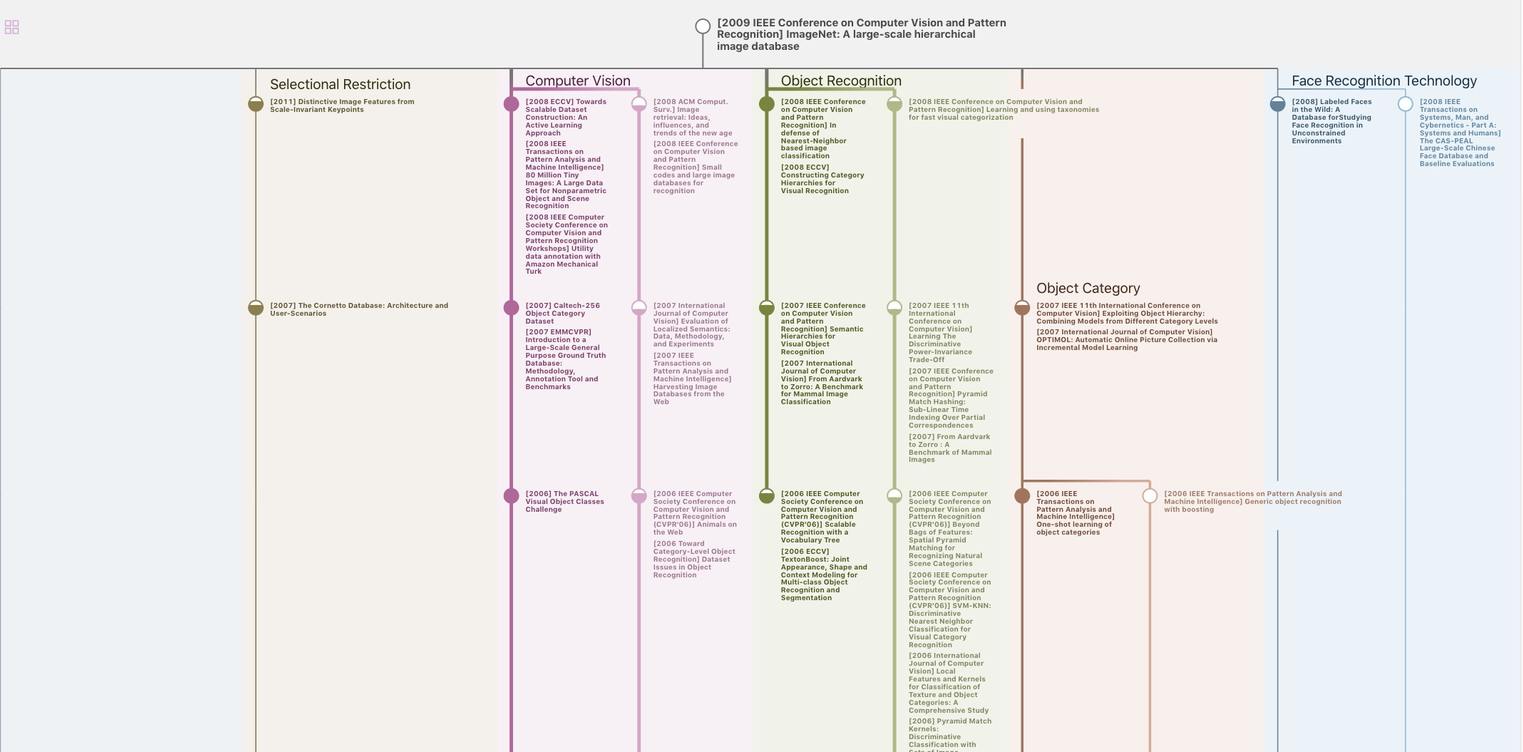
生成溯源树,研究论文发展脉络
Chat Paper
正在生成论文摘要