In-The-Wild End-To-End Detection Of Speech Affecting Diseases
2019 IEEE AUTOMATIC SPEECH RECOGNITION AND UNDERSTANDING WORKSHOP (ASRU 2019)(2019)
摘要
Speech is a complex bio-signal that has the potential to provide a rich bio-marker for health. It enables the development of non-invasive routes to early diagnosis and monitoring of speech affecting diseases, such as the ones studied in this work: Depression, and Parkinson's Disease.However, the major limitation of current speech based diagnosis and monitoring tools is the lack of large and diverse datasets. Existing datasets are small, and collected under very controlled conditions. As such, there is an upper bound in the complexity of the models that can be trained using these datasets. There is also limited applicability in real life scenarios where the channel and noise conditions, among others, are impossible to control.In this work, we show that datasets collected from in-thewild sources, such as collections of vlogs, can contribute to improve the performance of diagnosis tools both in controlled and in-the-wild conditions, even though the data are noisier.Moreover, we show that it is possible to successfully move away from hand-crafted features (i.e. features that are computed based on predefined algorithms, that based on human expertise) and adopt end-to-end modeling paradigms, such as CNN-LSTMs, that extract data driven features from the raw spectrograms of the speech signal, and capture temporal information from the speech signals.
更多查看译文
关键词
e-health, end-to-end machine learning, speech affecting diseases, in-the-wild data
AI 理解论文
溯源树
样例
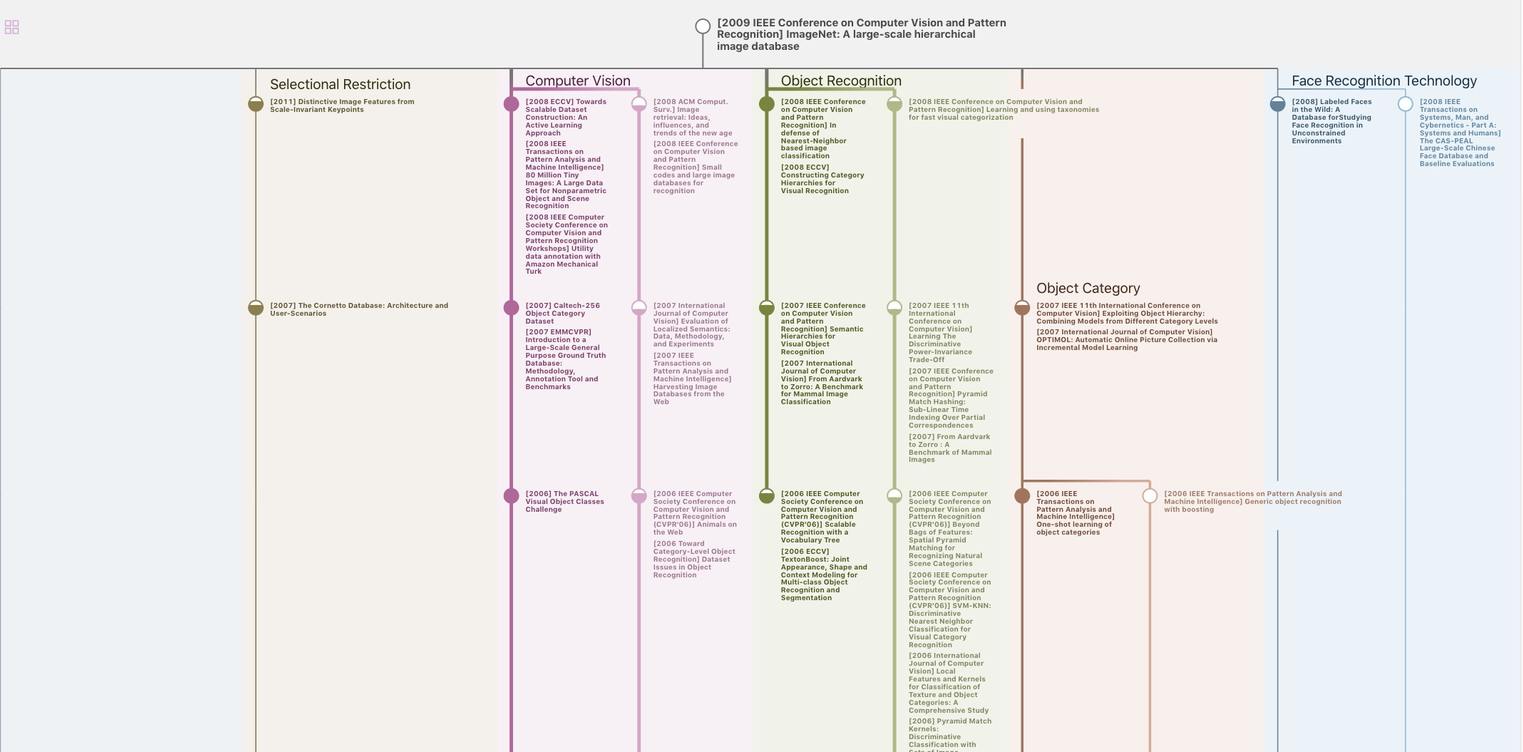
生成溯源树,研究论文发展脉络
Chat Paper
正在生成论文摘要