Improving Grapheme-to-Phoneme Conversion by Investigating Copying Mechanism in Recurrent Architectures
2019 IEEE Automatic Speech Recognition and Understanding Workshop (ASRU)(2019)
摘要
Attention driven encoder-decoder architectures have become highly successful in various sequence-to-sequence learning tasks. We propose copy-augmented Bi-directional Long Short-Term Memory based Encoder-Decoder architecture for the Grapheme-to-Phoneme conversion. In Grapheme-to-Phoneme task, a number of character units in words possess high degree of similarity with some phoneme unit(s). Thus, we make an attempt to capture this characteristic using copy-augmented architecture. Our proposed model automatically learns to generate phoneme sequences during inference by copying source token embeddings to the decoder's output in a controlled manner. To our knowledge, this is the first time the copy-augmentation is being investigated for Grapheme-to-Phoneme conversion task. We validate our experiments over accented and non-accented publicly available CMU-Dict datasets and achieve State-of-The-Art performances in terms of both phoneme and word error rates. Further, we verify the applicability of our proposed approach on Hindi Lexicon and show that our model outperforms all recent State-of-The-Art results.
更多查看译文
关键词
Grapheme-to-Phoneme,Copy augmentation,encoder-decoder,attention
AI 理解论文
溯源树
样例
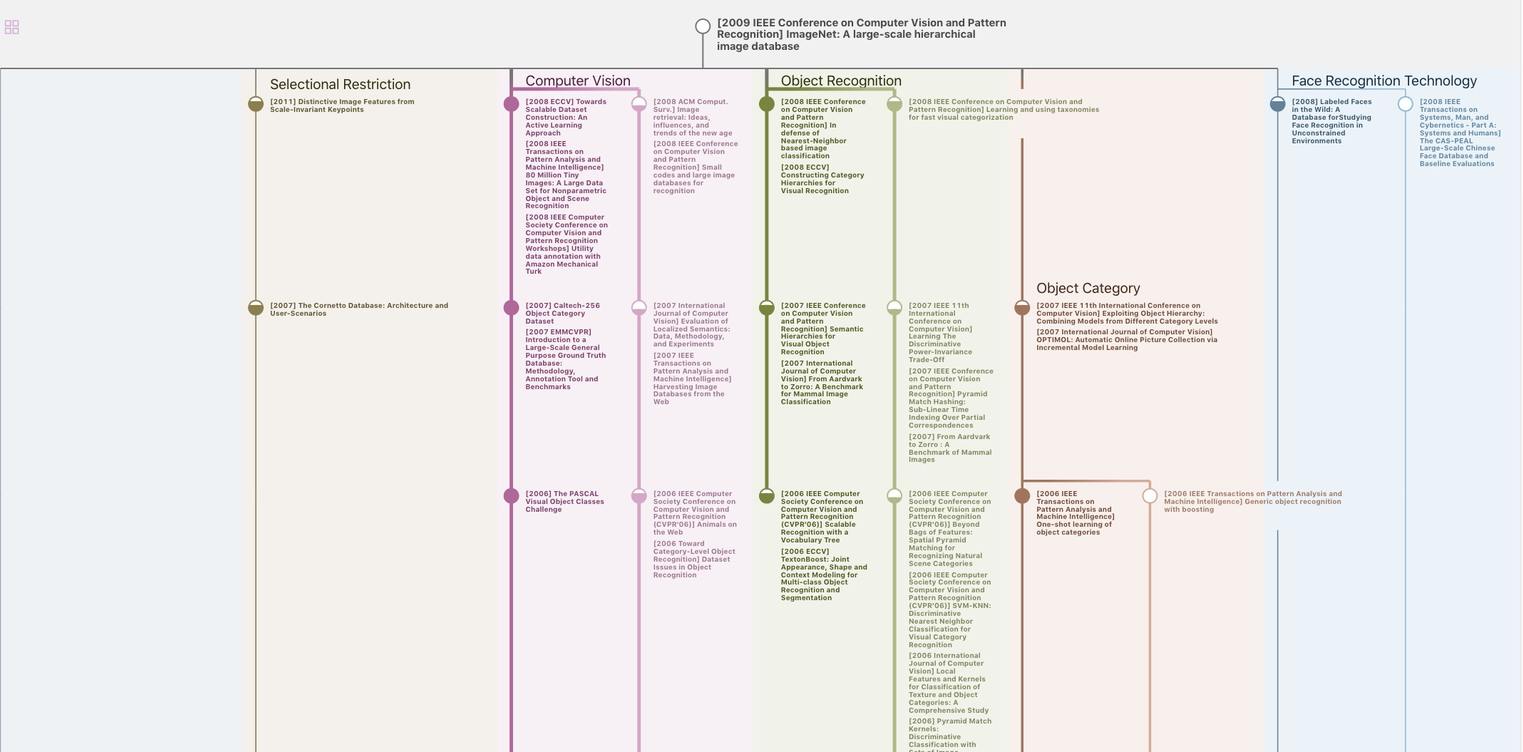
生成溯源树,研究论文发展脉络
Chat Paper
正在生成论文摘要