Three Approaches for Personalization with Applications to Federated Learning
arxiv(2020)
摘要
The standard objective in machine learning is to train a single model for all users. However, in many learning scenarios, such as cloud computing and federated learning, it is possible to learn one personalized model per user. In this work, we present a systematic learning-theoretic study of personalization. We propose and analyze three approaches: user clustering, data interpolation, and model interpolation. For all three approaches, we provide learning-theoretic guarantees and efficient algorithms for which we also demonstrate the performance empirically. All of our algorithms are model agnostic and work for any hypothesis class.
更多查看译文
关键词
federated learning,personalization,applications
AI 理解论文
溯源树
样例
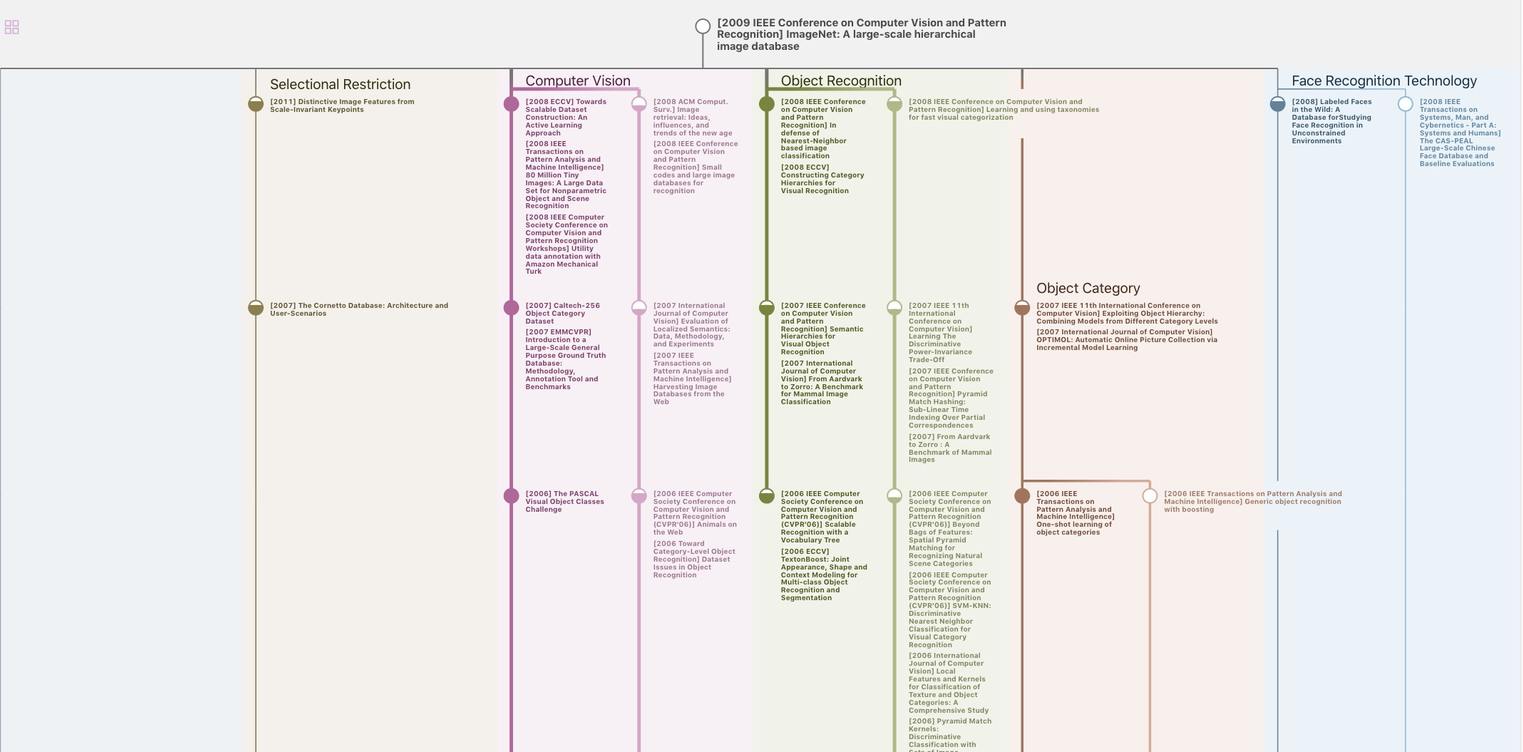
生成溯源树,研究论文发展脉络
Chat Paper
正在生成论文摘要