Deep Neural Network for Accurate and Efficient Atomistic Modeling of Phase Change Memory
IEEE electron device letters(2020)
摘要
This letter presents a general-purpose fully-atomistic method to simulate phase change memory (PCM), by combining density functional theory (DFT) and deep neural network (DNN). Its maximum calculation error of atomic forces is about 10(-1) eV/angstrom, which is 1-2 orders of magnitude more accurate than state-of-art artificial neural network (ANN) in PCM literature (over 10(1) eV/angstrom). Its simulation time, t(s), scales linearlywith the number of atoms n(a) (t(s) proportional to n(a)), which is more efficient than DFT (t(s) proportional to n(a)(3)) widely used to model PCM, leading to approximately 2, 4, 6 orders of magnitude reduction of modeling time when n(a) approximate to 10(1), 10(2), 10(3), for instance. Its efficiency and accuracy may be useful to develop next-generation atomistic modeling tools to enable in-depth optimization of PCM.
更多查看译文
关键词
Phase change memory,artificial neural network,chalcogenide,amorphization,crystallization,nucleation,growth
AI 理解论文
溯源树
样例
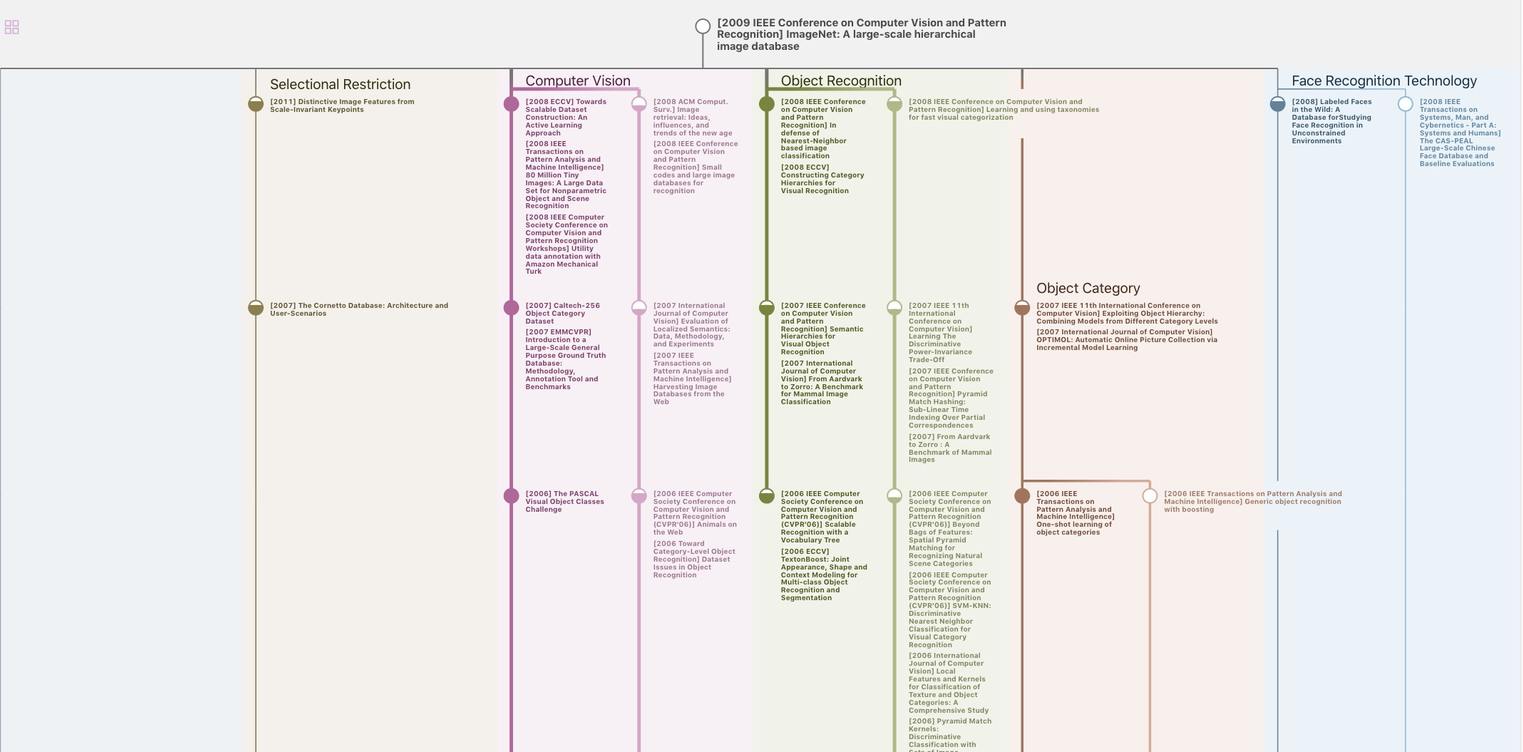
生成溯源树,研究论文发展脉络
Chat Paper
正在生成论文摘要