Optimal Computing Budget Allocation For Binary Classification With Noisy Labels And Its Applications On Simulation Analytics
2019 WINTER SIMULATION CONFERENCE (WSC)(2019)
摘要
In this study, we consider the budget allocation problem for binary classification with noisy labels. The classification accuracy can be improved by reducing the label noises which can be achieved by observing multiple independent observations of the labels. Hence, an efficient budget allocation strategy is needed to reduce the label noise and meanwhile guarantees a promising classification accuracy. Two problem settings are investigated in this work. One assumes that we do not know the underlying classification structures and labels can only be determined by comparing the sample average of its Bernoulli success probability with a given threshold. The other case assumes that data points with different labels can be separated by a hyperplane. For both cases, the closed-form optimal budget allocation strategies are developed. A simulation analytics example is used to demonstrate how the budget is allocated to different scenarios to further improve the learning of optimal decision functions.
更多查看译文
关键词
optimal computing budget allocation,binary classification,label noise,classification structures,closed-form optimal budget allocation strategies,budget allocation strategy
AI 理解论文
溯源树
样例
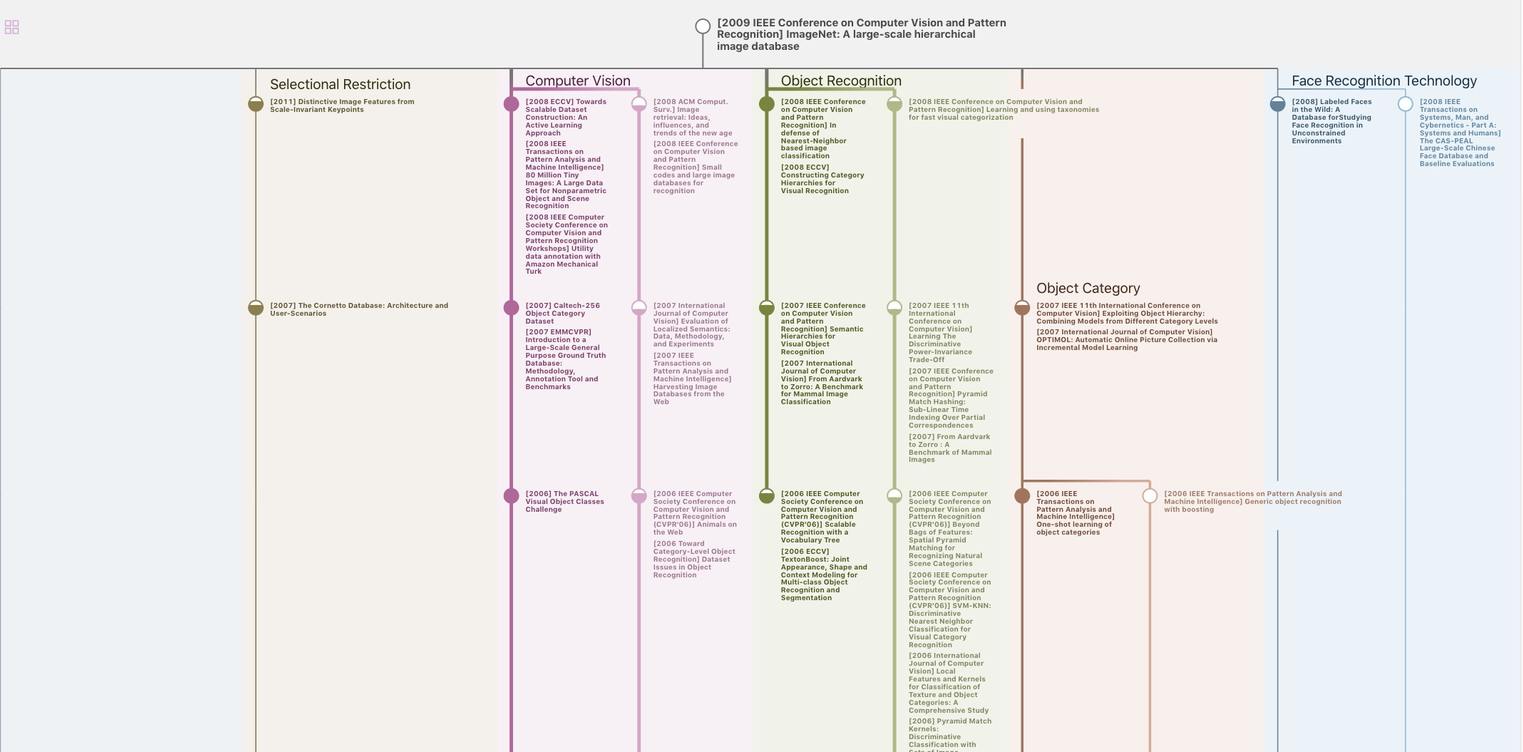
生成溯源树,研究论文发展脉络
Chat Paper
正在生成论文摘要