Bayesian Sequential Experimental Design for Stochastic Kriging with Jackknife Error Estimates
2019 WINTER SIMULATION CONFERENCE (WSC)(2019)
摘要
We propose a fully sequential experimental design procedure for stochastic kriging (SK) methodology of fitting unknown response surfaces from simulation experiments. The procedure first estimates the current SK model performance by jackknifing the existing data points. Then, an additional SK model is fitted on the jackknife error estimates to capture the landscape of the current SK model performance. Methodologies for balancing exploration and exploitation trade-off in Bayesian optimization are employed to select the next simulation point. Compared to experimental design procedures, our method is robust to the SK model specifications. We design a dynamic allocation algorithm, which we call kriging-based dynamic stochastic kriging (KDSK), and illustrate its performance through two numerical experiments.
更多查看译文
关键词
Bayesian sequential experimental design,jackknife error estimates,fully sequential experimental design procedure,stochastic kriging methodology,unknown response surfaces,simulation experiments,current SK model performance,existing data points,additional SK model,exploitation trade,Bayesian optimization,simulation point,experimental design procedures,model specifications,dynamic stochastic kriging
AI 理解论文
溯源树
样例
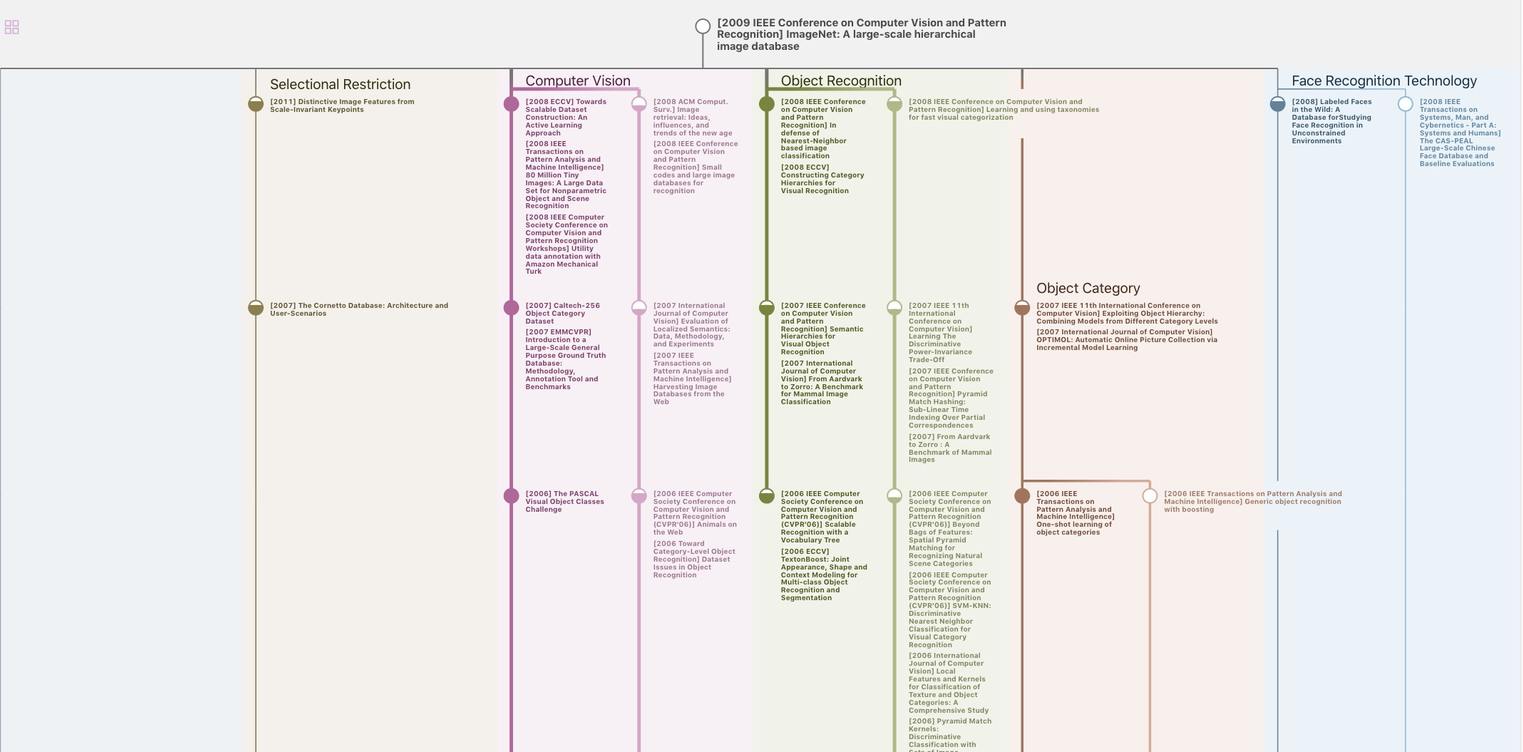
生成溯源树,研究论文发展脉络
Chat Paper
正在生成论文摘要