Efficient Semantic Video Segmentation with Per-Frame Inference
European Conference on Computer Vision(2020)
摘要
For semantic segmentation, most existing real-time deep models trained with each frame independently may produce inconsistent results when tested on a video sequence. A few methods take the correlations in the video sequence into account, e.g., by propagating the results to the neighbouring frames using optical flow, or extracting frame representations using multi-frame information, which may lead to inaccurate results or unbalanced latency. In contrast, here we explicitly consider the temporal consistency among frames as extra constraints during training and process each frame independently in the inference phase. Thus no computation overhead is introduced for inference. Compact models are employed for real-time execution. To narrow the performance gap between compact models and large models, new temporal knowledge distillation methods are designed. Weighing among accuracy, temporal smoothness and efficiency, our proposed method outperforms previous keyframe based methods and corresponding baselines which are trained with each frame independently on benchmark datasets including Cityscapes and Camvid. Code is available at: https://git.io/vidseg.
更多查看译文
关键词
video,per-frame
AI 理解论文
溯源树
样例
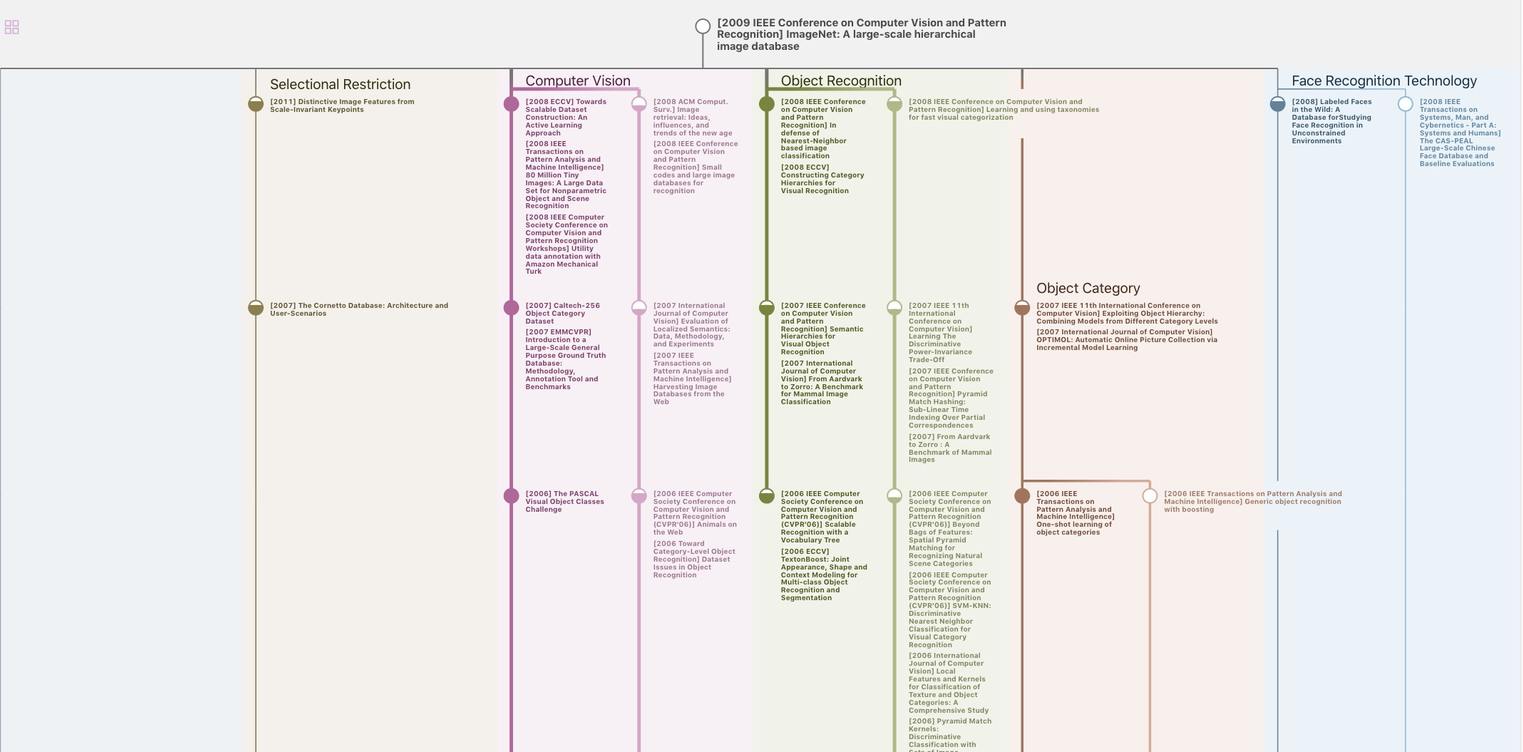
生成溯源树,研究论文发展脉络
Chat Paper
正在生成论文摘要