Learning a Directional Soft Lane Affordance Model for Road Scenes Using Self-Supervision
2020 IEEE INTELLIGENT VEHICLES SYMPOSIUM (IV)(2020)
摘要
Humans navigate complex environments in an organized yet flexible manner, adapting to the context and implicit social rules. Understanding these naturally learned patterns of behavior is essential for applications such as autonomous vehicles. However, algorithmically defining these implicit rules of human behavior remains difficult. This work proposes a novel self-supervised method for training a probabilistic network model to estimate the regions humans are most likely to drive in as well as a multimodal representation of the inferred direction of travel at each point. The model is trained on individual human trajectories conditioned on a representation of the driving environment. The model is shown to successfully generalize to new road scenes, demonstrating potential for real-world application as a prior for socially acceptable driving behavior in challenging or ambiguous scenarios which are poorly handled by explicit traffic rules.
更多查看译文
关键词
autonomous vehicles,human behavior,self-supervised method,probabilistic network model,multimodal representation,driving environment,road scenes,socially acceptable driving behavior,traffic rules,directional soft lane affordance model learning
AI 理解论文
溯源树
样例
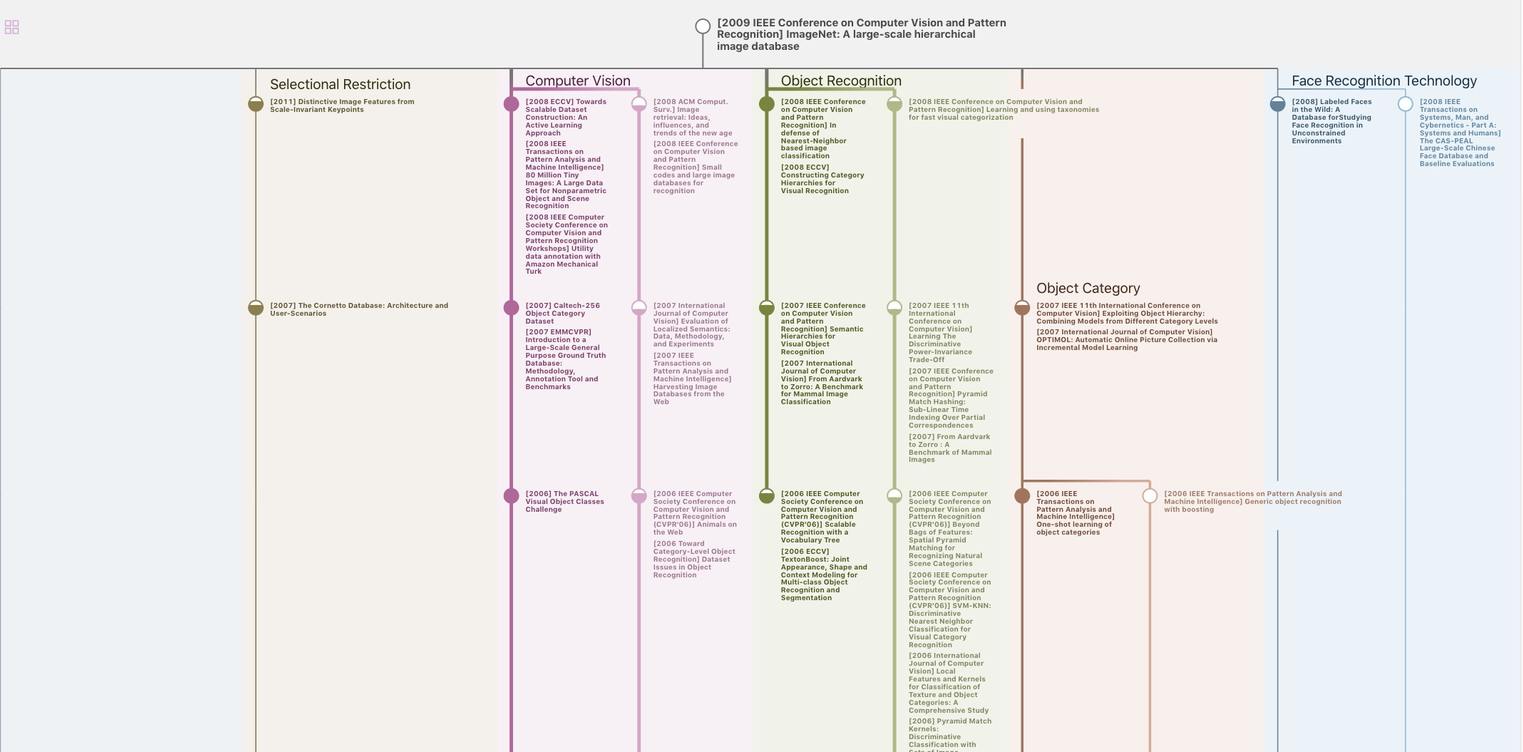
生成溯源树,研究论文发展脉络
Chat Paper
正在生成论文摘要