Online Learning in Contextual Bandits using Gated Linear Networks
NIPS 2020(2020)
摘要
We introduce a new and completely online contextual bandit algorithm called Gated Linear Contextual Bandits (GLCB). This algorithm is based on Gated Linear Networks (GLNs), a recently introduced deep learning architecture with properties well-suited to the online setting. Leveraging data-dependent gating properties of the GLN we are able to estimate prediction uncertainty with effectively zero algorithmic overhead. We empirically evaluate GLCB compared to 9 state-of-the-art algorithms that leverage deep neural networks, on a standard benchmark suite of discrete and continuous contextual bandit problems. GLCB obtains median first-place despite being the only online method, and we further support these results with a theoretical study of its convergence properties.
更多查看译文
关键词
contextual bandits,online learning,gated linear networks
AI 理解论文
溯源树
样例
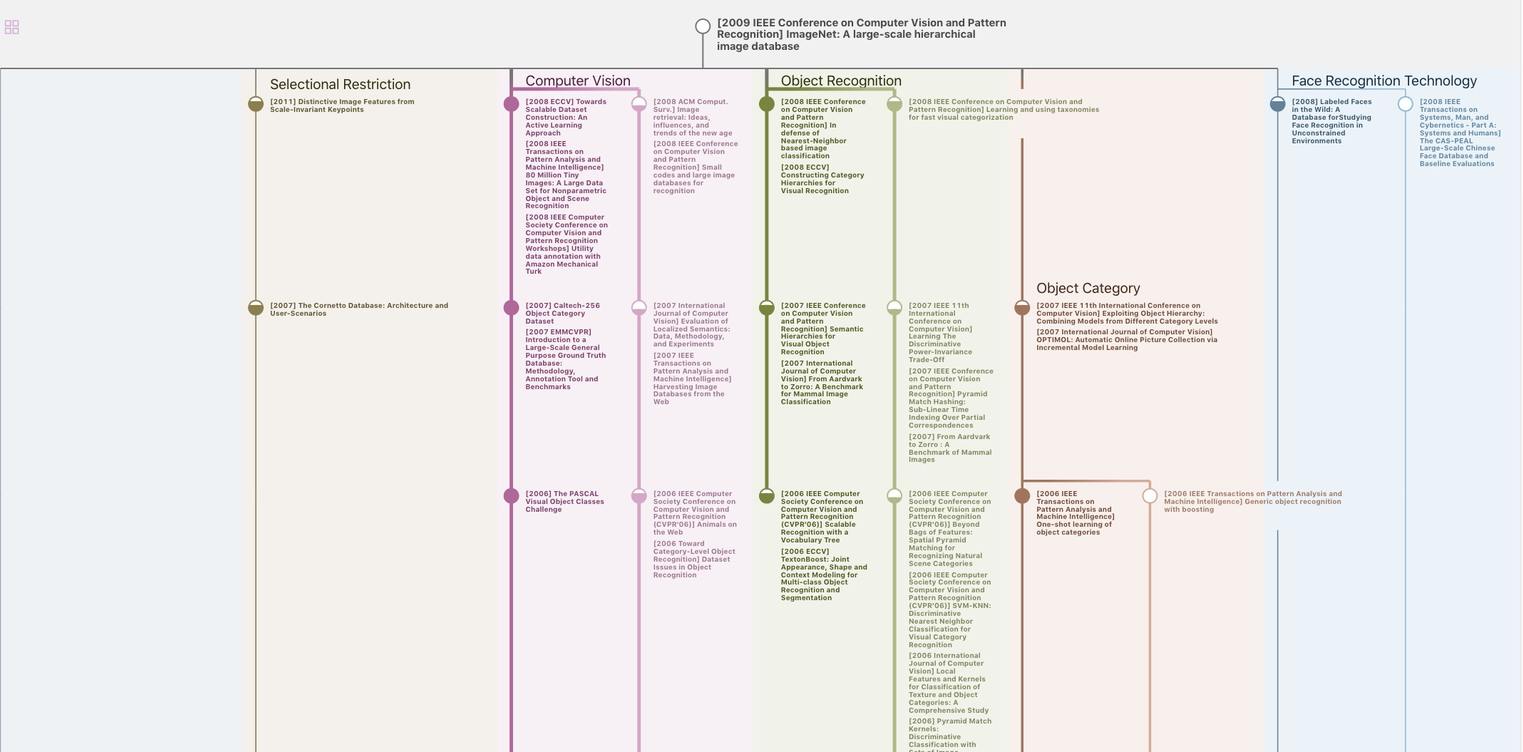
生成溯源树,研究论文发展脉络
Chat Paper
正在生成论文摘要