Sim2Real Transfer for Reinforcement Learning without Dynamics Randomization
IROS(2020)
摘要
We show how to use the Operational Space Control framework (OSC) under joint and Cartesian constraints for reinforcement learning in Cartesian space. Our method is able to learn fast and with adjustable degrees of freedom, while we are able to transfer policies without additional dynamics randomizations on a KUKA LBR iiwa peg-in-hole task. Before learning in simulation starts, we perform a system identification for aligning the simulation environment as far as possible with the dynamics of a real robot. Adding constraints to the OSC controller allows us to learn in a safe way on the real robot or to learn a flexible, goal conditioned policy that can be easily transferred from simulation to the real robot.(1)
更多查看译文
关键词
dynamics randomization,sim2real transfer,reinforcement learning
AI 理解论文
溯源树
样例
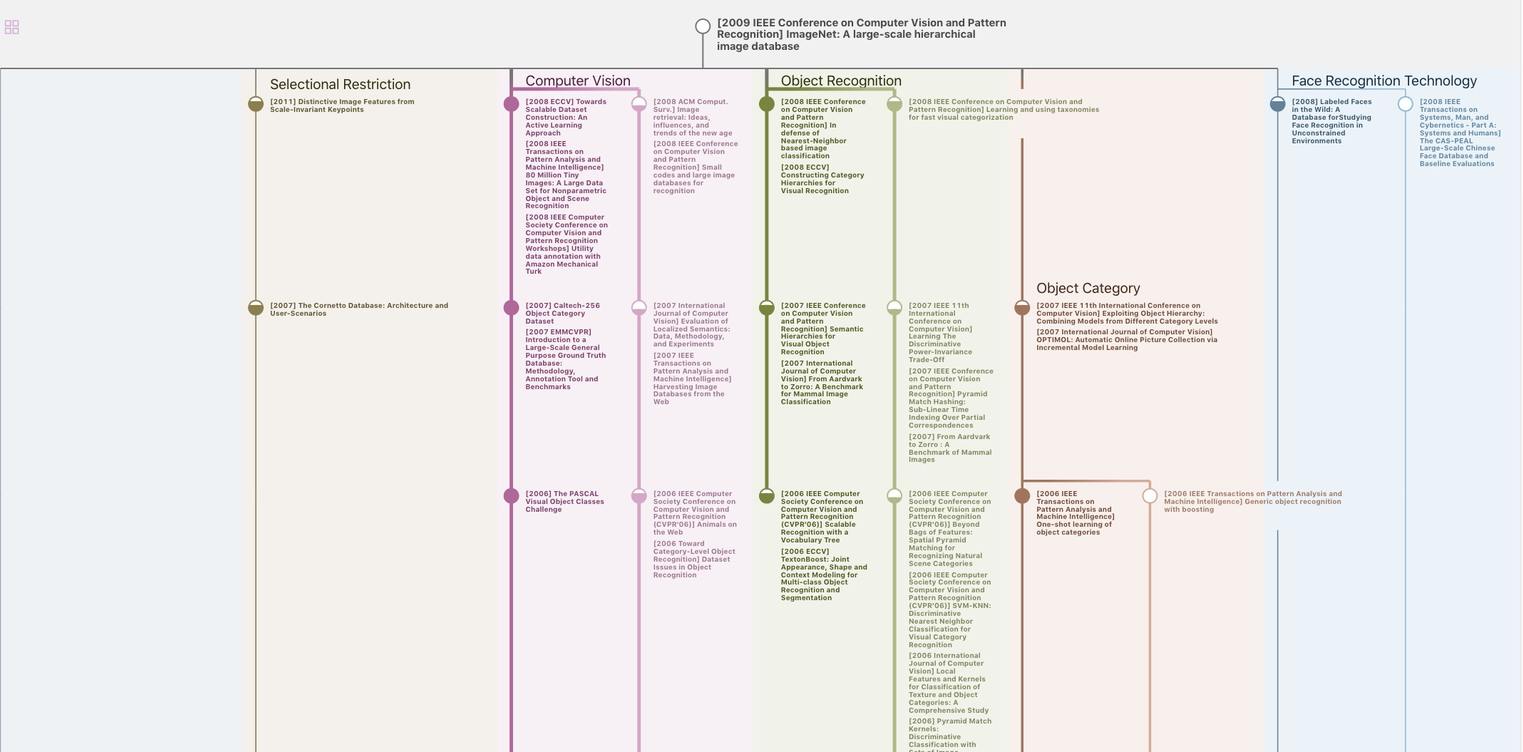
生成溯源树,研究论文发展脉络
Chat Paper
正在生成论文摘要