Segmentation and visualization of left atrium through a unified deep learning framework
International Journal of Computer Assisted Radiology and Surgery(2020)
摘要
Purpose Left atrium segmentation and visualization serve as a fundamental and crucial role in clinical analysis and understanding of atrial fibrillation. However, most of the existing methods are directly transmitting information, which may cause redundant information to be passed to affect segmentation performance. Moreover, they did not further consider atrial visualization after segmentation, which leads to a lack of understanding of the essential atrial anatomy. Methods We propose a novel unified deep learning framework for left atrium segmentation and visualization simultaneously. At first, a novel dual-path module is used to enhance the expressiveness of cardiac image representation. Then a multi-scale context-aware module is designed to effectively handle complex appearance and shape variations of the left atrium and associated pulmonary veins. The generated multi-scale features are feed to gated bidirectional message passing module to remove irrelevant information and extract discriminative features. Finally, the features after message passing are efficiently combined via a deep supervision mechanism to produce the final segmentation result and reconstruct 3D volumes. Results Our approach primarily against the 2018 left atrium segmentation challenge dataset, which consists of 100 3D gadolinium-enhanced magnetic resonance images. Our method achieves an average dice of 0.936 in segmenting the left atrium via fivefold cross-validation, which outperforms state-of-the-art methods. Conclusions The performance demonstrates the effectiveness and advantages of our network for the left atrium segmentation and visualization. Therefore, our proposed network could potentially improve the clinical diagnosis and treatment of atrial fibrillation.
更多查看译文
关键词
Left atrium,Gadolinium-enhanced magnetic resonance image,Segmentation,Visualization
AI 理解论文
溯源树
样例
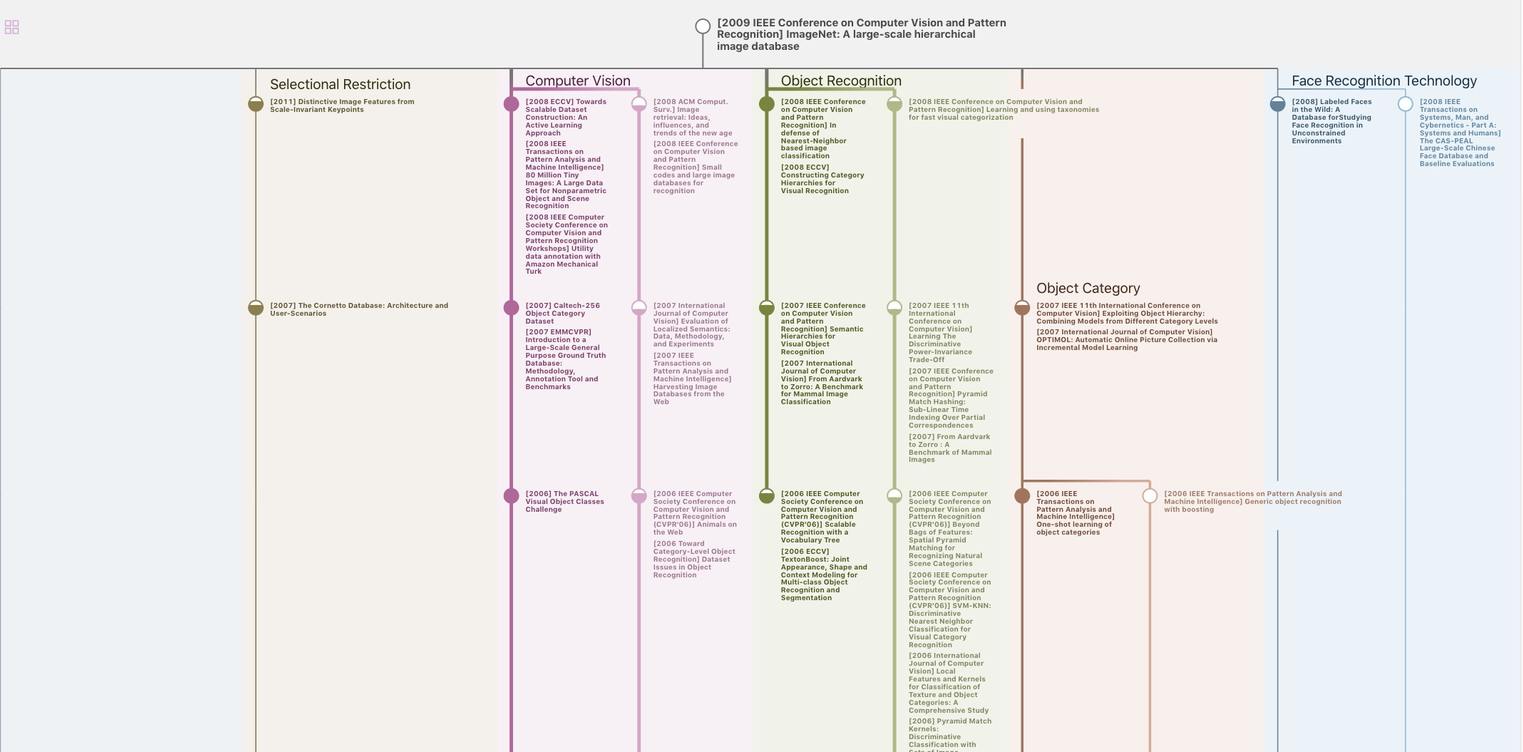
生成溯源树,研究论文发展脉络
Chat Paper
正在生成论文摘要