Multi-source Domain Adaptation in the Deep Learning Era: A Systematic Survey
arxiv(2020)
摘要
In many practical applications, it is often difficult and expensive to obtain enough large-scale labeled data to train deep neural networks to their full capability. Therefore, transferring the learned knowledge from a separate, labeled source domain to an unlabeled or sparsely labeled target domain becomes an appealing alternative. However, direct transfer often results in significant performance decay due to domain shift. Domain adaptation (DA) addresses this problem by minimizing the impact of domain shift between the source and target domains. Multi-source domain adaptation (MDA) is a powerful extension in which the labeled data may be collected from multiple sources with different distributions. Due to the success of DA methods and the prevalence of multi-source data, MDA has attracted increasing attention in both academia and industry. In this survey, we define various MDA strategies and summarize available datasets for evaluation. We also compare modern MDA methods in the deep learning era, including latent space transformation and intermediate domain generation. Finally, we discuss future research directions for MDA.
更多查看译文
关键词
adaptation,deep learning era,multi-source
AI 理解论文
溯源树
样例
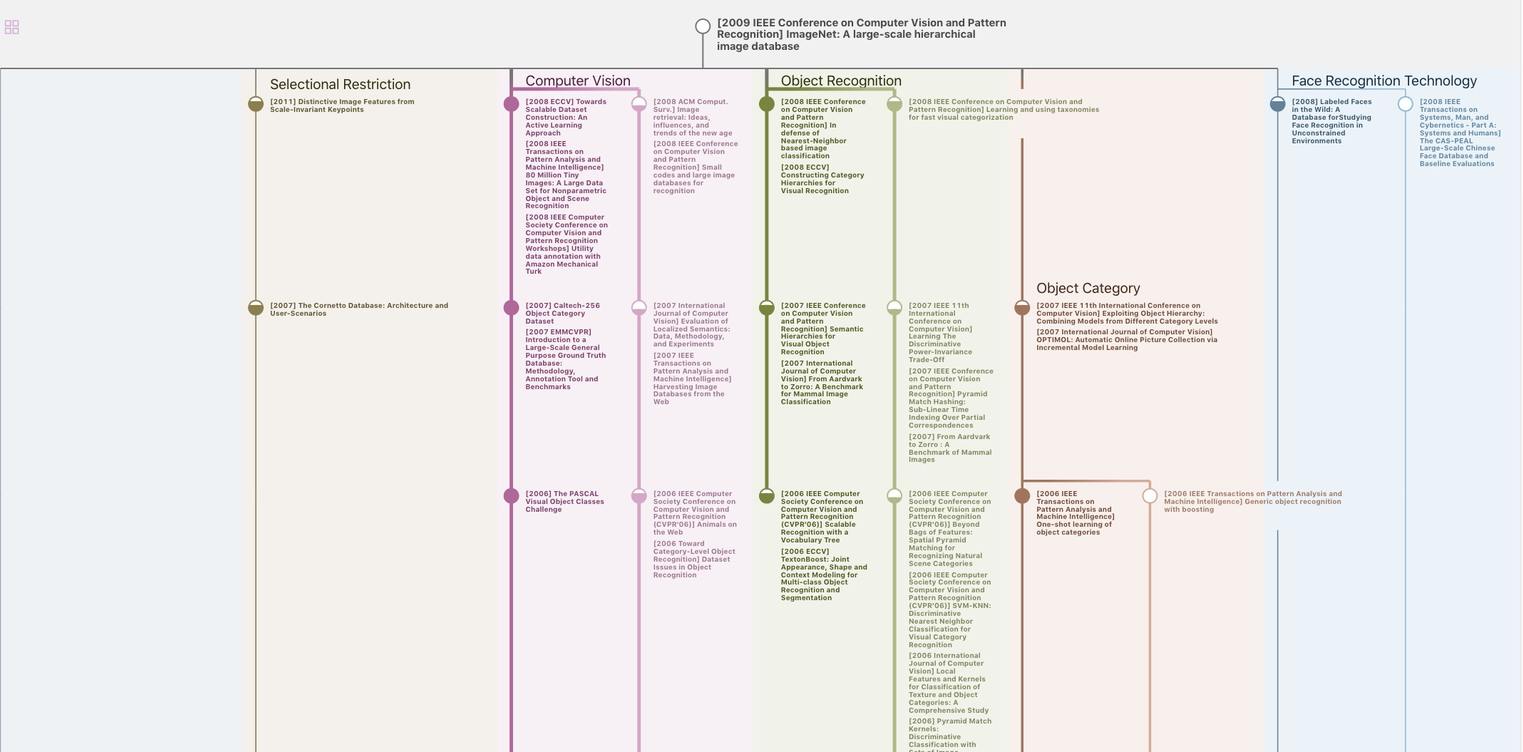
生成溯源树,研究论文发展脉络
Chat Paper
正在生成论文摘要