Evolving Ensembles Of Routing Policies Using Genetic Programming For Uncertain Capacitated Arc Routing Problem
2019 IEEE SYMPOSIUM SERIES ON COMPUTATIONAL INTELLIGENCE (IEEE SSCI 2019)(2019)
摘要
The Uncertain Capacitated Arc Routing Problem (UCARP) has a wide range of real-world applications. Genetic Programming Hyper-heuristic (GPHH) approaches have shown success in solving UCARP to evolve routing policies that generate routes in real time. However, existing GPHH approaches still have a drawback. Despite the effectiveness in many benchmarks, the single routing policy evolved by GPHH is too complex to interpret. On the other hand, the users need to be able to understand the evolved routing policies to feel confident to use them. In this paper, we aim to employ three ensemble methods, BaggingGP, BoostingGP and Cooperative Co-evolution GP (CCGP) to evolve a group of interpretable routing policies. The ensemble can be used to compare with single complex routing policy from GPHH. Experiment studies show that CCGP significantly outperformed BaggingGP and BoostingGP, and can generate much smaller and simpler routing policies to form ensembles with comparable test performance as the routing policy evolved by SimpleGP. This demonstrates the potential of improving the interpretability issue of GPHH using ensemble methods.
更多查看译文
关键词
UCARP, GPHH, ensemble learning, routing policy
AI 理解论文
溯源树
样例
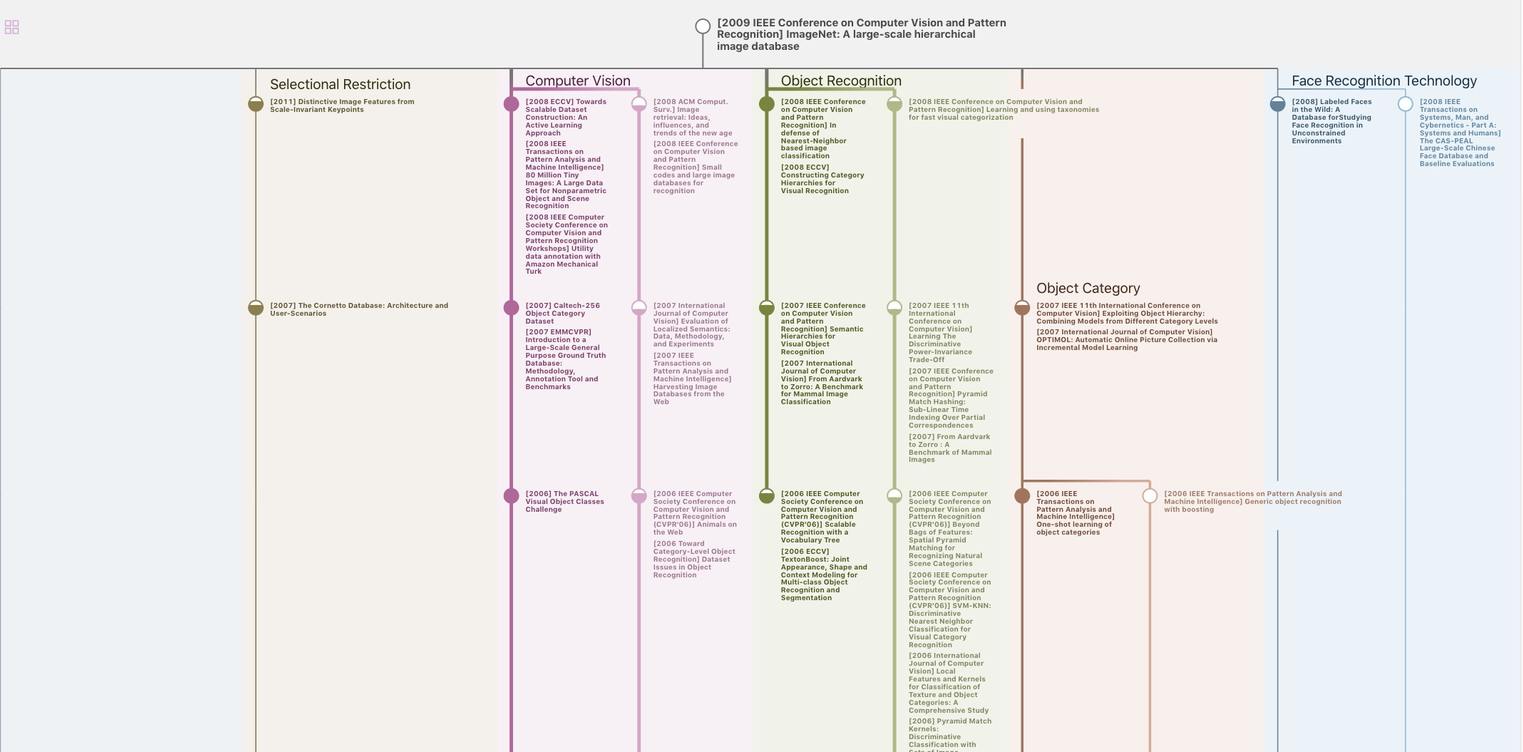
生成溯源树,研究论文发展脉络
Chat Paper
正在生成论文摘要