Least Squares Twin Support Vector Machine Based on Manifold-based Within-class Scatter
2019 IEEE SYMPOSIUM SERIES ON COMPUTATIONAL INTELLIGENCE (IEEE SSCI 2019)(2019)
摘要
Although twin support vector machine (TWSVM) is widely used in practice, it only takes the empirical risk minimization principle into consideration while neglects the data distribution information, which seriously limits the classification performance. In view of this, robust minimum class variance twin support vector machine (RMCV-TWSVM) is proposed. Compared with TWSVM, RMCV-TWSVM has better generalization ability because it takes both the empirical risk minimization principle and data distribution characteristics into consideration. While TWSVM and RMCV-TWSVM always neglect the local characteristics of each class sample. Based on the above analysis, this paper presents least squares twin support vector machine based on manifold-based within-class scatter (MWCS-LSTSVM), which not only focuses on the empirical risk minimization principle and data distribution characteristics, but also preserves the local manifold structure of each class sample. At the same time, the solutions of MWCS-LSTSVM comes directly from solving a pair of systems of linear equations rather than solving two quadratic programming problems. Thus, MWCS-LSTSVM has low time complexity. The experimental results show the significant advantages of proposed approach in both classification accuracy and computational time.
更多查看译文
关键词
generalization, least squares twin support vector machine, manifidd-based within-class scatter
AI 理解论文
溯源树
样例
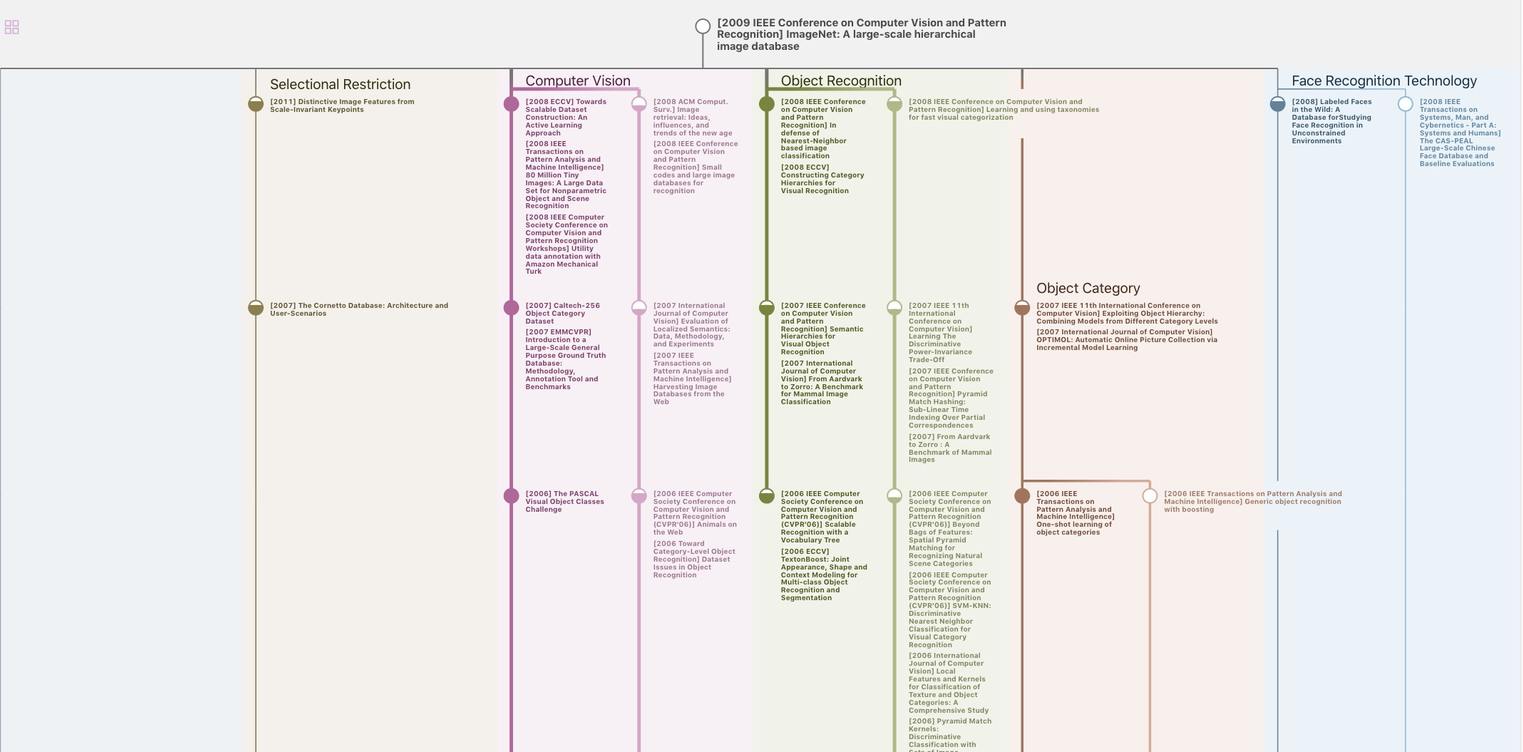
生成溯源树,研究论文发展脉络
Chat Paper
正在生成论文摘要