LEEP: A New Measure to Evaluate Transferability of Learned Representations
international conference on machine learning(2020)
摘要
We introduce a new measure to evaluate the transferability of representations learned by classifiers. Our measure, the Log Expected Empirical Prediction (LEEP), is simple and easy to compute: when given a classifier trained on a source data set, it only requires running the target data set through this classifier once. We analyze the properties of LEEP theoretically and demonstrate its effectiveness empirically. Our analysis shows that LEEP can predict the performance and convergence speed of both transfer and meta-transfer learning methods, even for small or imbalanced data. Moreover, LEEP outperforms recently proposed transferability measures such as negative conditional entropy and H scores. Notably, when transferring from ImageNet to CIFAR100, LEEP can achieve up to 30% improvement compared to the best competing method in terms of the correlations with actual transfer accuracy.
更多查看译文
关键词
transferability,leep
AI 理解论文
溯源树
样例
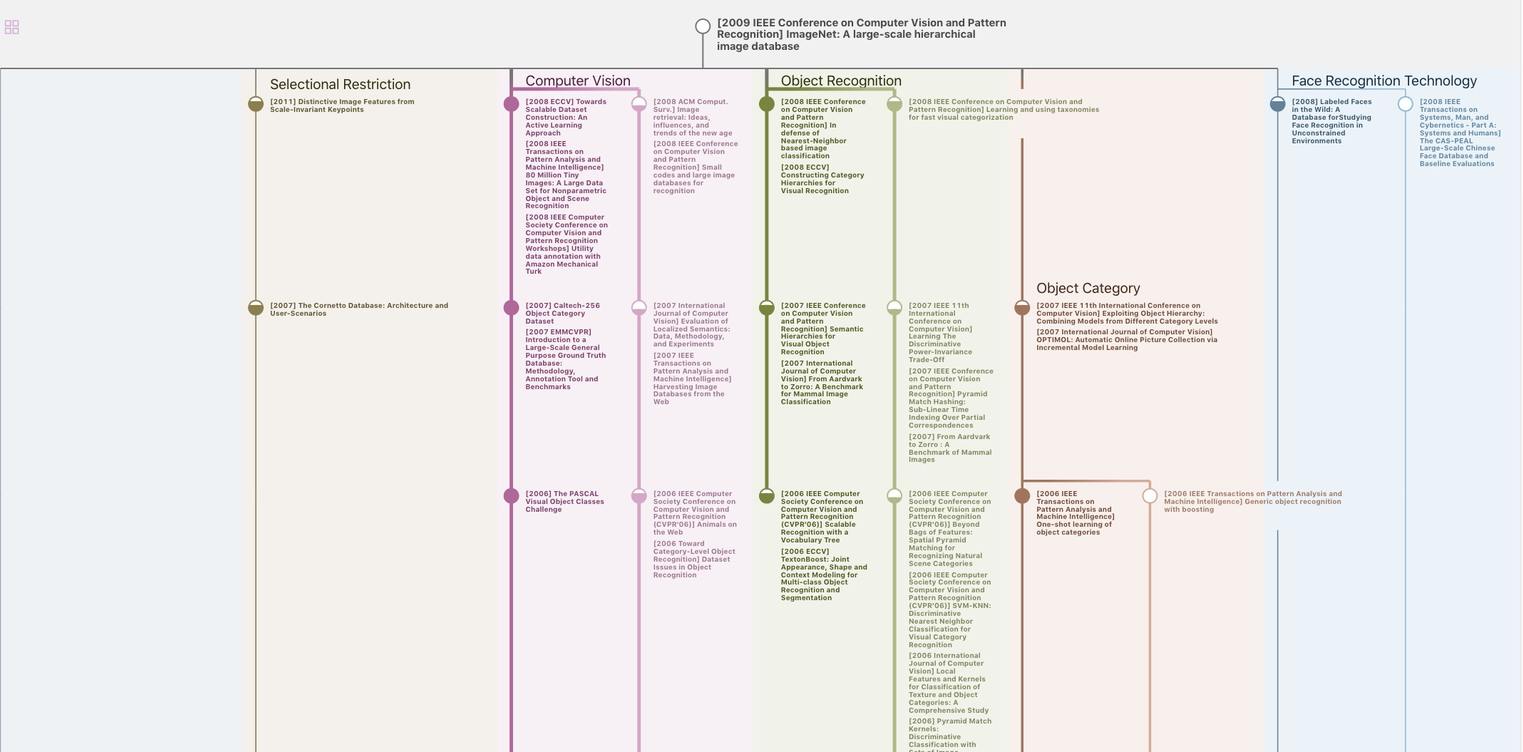
生成溯源树,研究论文发展脉络
Chat Paper
正在生成论文摘要