Generalized Sliced Distances for Probability Distributions
arxiv(2020)
摘要
Probability metrics have become an indispensable part of modern statistics and machine learning, and they play a quintessential role in various applications, including statistical hypothesis testing and generative modeling. However, in a practical setting, the convergence behavior of the algorithms built upon these distances have not been well established, except for a few specific cases. In this paper, we introduce a broad family of probability metrics, coined as Generalized Sliced Probability Metrics (GSPMs), that are deeply rooted in the generalized Radon transform. We first verify that GSPMs are metrics. Then, we identify a subset of GSPMs that are equivalent to maximum mean discrepancy (MMD) with novel positive definite kernels, which come with a unique geometric interpretation. Finally, by exploiting this connection, we consider GSPM-based gradient flows for generative modeling applications and show that under mild assumptions, the gradient flow converges to the global optimum. We illustrate the utility of our approach on both real and synthetic problems.
更多查看译文
关键词
generalized sliced distances,distributions
AI 理解论文
溯源树
样例
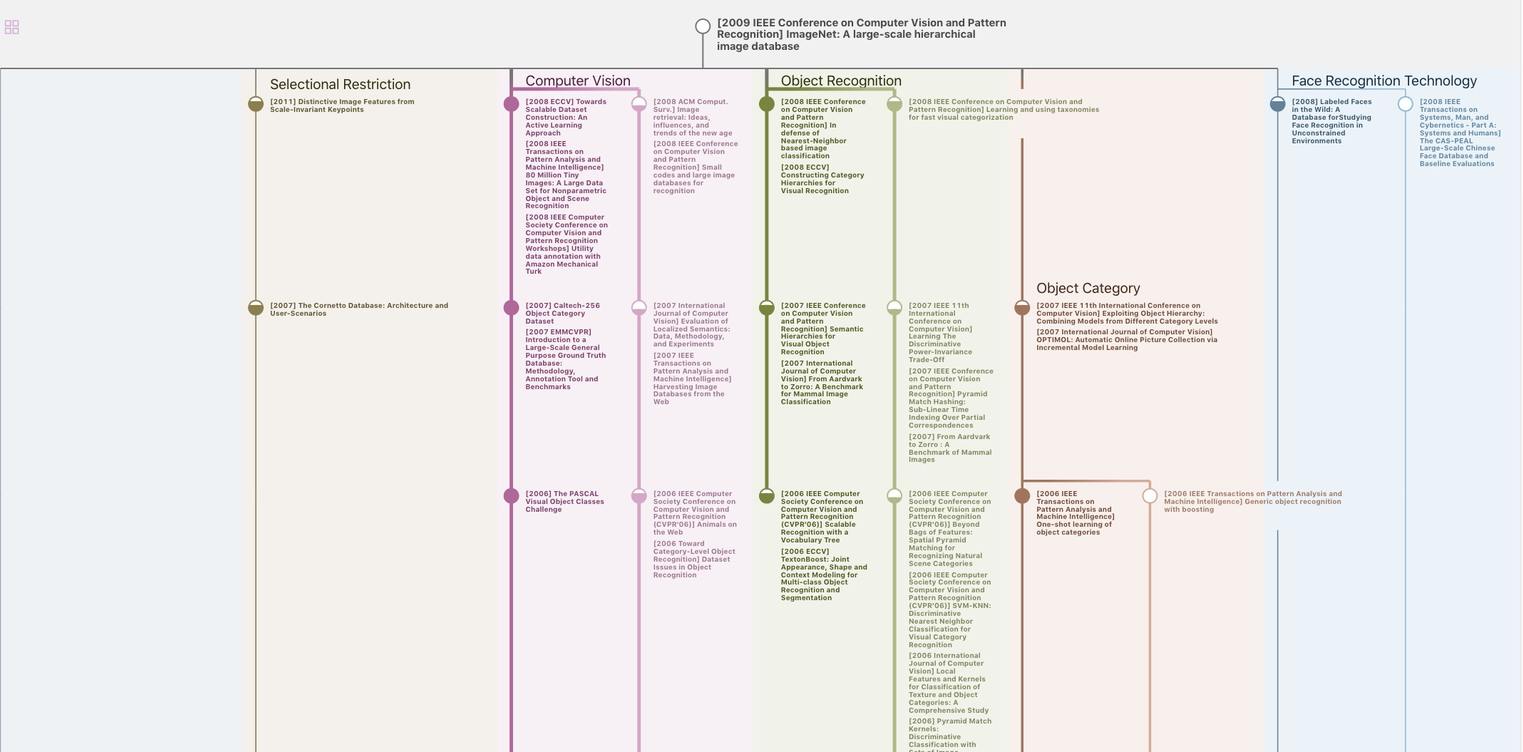
生成溯源树,研究论文发展脉络
Chat Paper
正在生成论文摘要