SCALE-Net: Scalable Vehicle Trajectory Prediction Network under Random Number of Interacting Vehicles via Edge-enhanced Graph Convolutional Neural Network
2020 IEEE/RSJ International Conference on Intelligent Robots and Systems (IROS)(2020)
摘要
Predicting the future trajectory of surrounding vehicles in a randomly varying traffic level is one of the most challenging problems in developing an autonomous vehicle. Since there is no pre-defined number of interacting vehicles participated in, the prediction network has to be scalable with respect to the number of vehicles in order to guarantee consistent performance in terms of both accuracy and computational load. In this paper, the first fully scalable trajectory prediction network, SCALE-Net, is proposed that can ensure both high prediction performance while keeping the computational load low regardless of the number of surrounding vehicles. The SCALE-Net employs the Edge-enhanced Graph Convolutional Neural Network (EGCN) for the inter-vehicular interaction embedding network. Since the proposed EGCN is inherently scalable with respect to the graph node (an agent in this study), the model can be operated independently from the total number of vehicles considered. We evaluated the scalability of the SCALE-Net on the publically available NGSIM datasets by comparing variations on computation time and prediction accuracy per single driving scene with respect to the varying vehicle number. The experimental test shows that both computation time and prediction performance of the SCALE-Net consistently outperform those of previous models regardless of the level of traffic complexities.
更多查看译文
关键词
autonomous vehicle,pre-defined number,interacting vehicles,fully scalable trajectory prediction network,SCALE-Net,high prediction performance,computational load low,edge-enhanced graph convolutional neural network,inter-vehicular interaction embedding network,computation time,prediction accuracy,varying vehicle number,scalable vehicle trajectory prediction network,random number,future trajectory,randomly varying traffic level
AI 理解论文
溯源树
样例
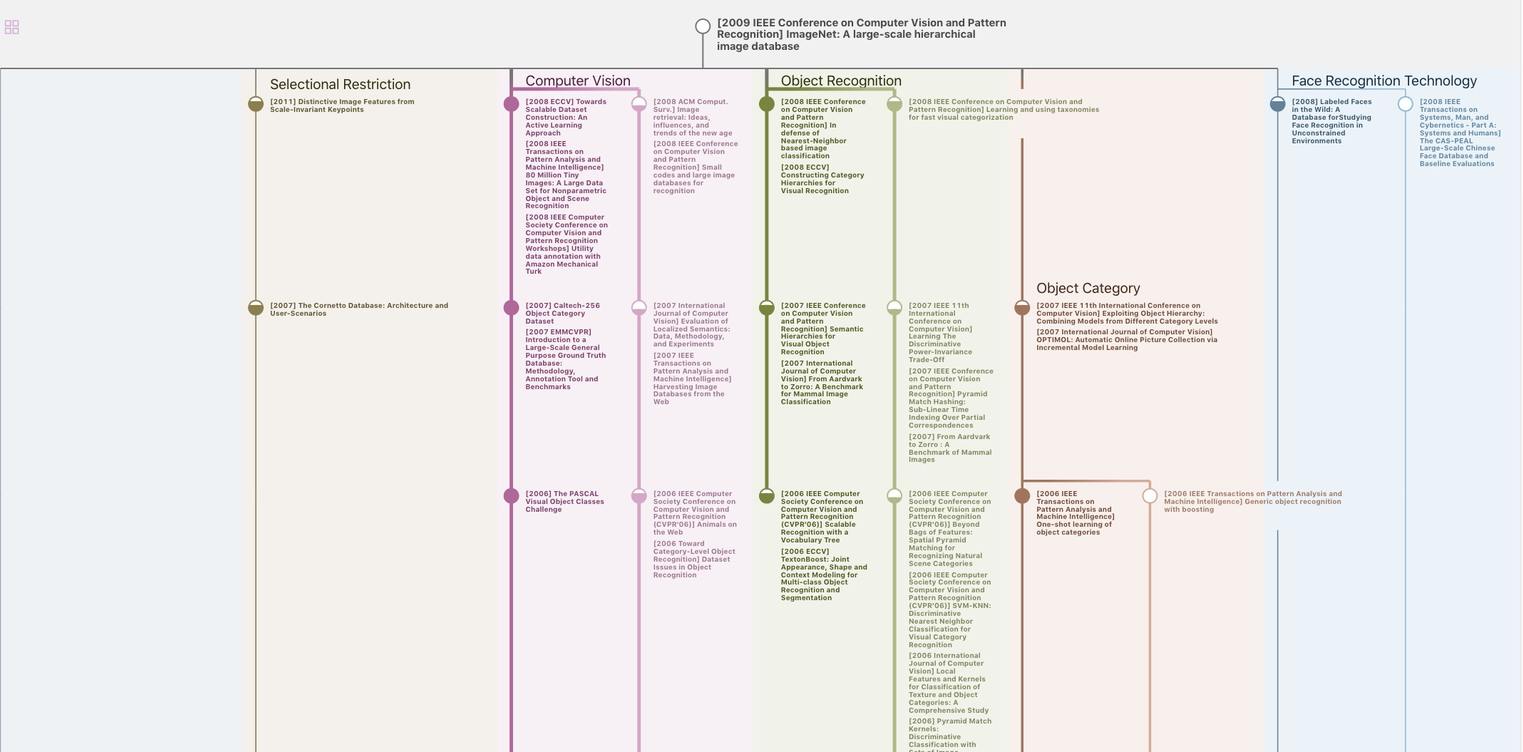
生成溯源树,研究论文发展脉络
Chat Paper
正在生成论文摘要