Ground-To-Aerial Image Geo-Localization Ith A Hard Exemplar Reweighting Triplet Loss
2019 IEEE/CVF INTERNATIONAL CONFERENCE ON COMPUTER VISION (ICCV 2019)(2019)
摘要
The task of ground-to-aerial image geo-localization can be achieved by matching a ground view query image to a reference database of aerial/satellite images. It is highly challenging due to the dramatic viewpoint changes and unknown orientations. In this paper, we propose a novel in-batch reweighting triplet loss to emphasize the positive effect of hard exemplars during end-to-end training. We also integrate an attention mechanism into our model using feature-level contextual information. To analyze the difficulty level of each triplet, we first enforce a modified logistic regression to triplets with a distance rectifying factor. Then, the reference negative distances for corresponding anchors are set, and the relative weights of triplets are computed by comparing their difficulty to the corresponding references. To reduce the influence of extreme hard data and less useful simple exemplars, the final weights are pruned using upper and lower bound constraints. Experiments on two benchmark datasets show that the proposed approach significantly outperforms the state-of-the-art methods.
更多查看译文
关键词
ground-to-aerial image geo-localization,hard exemplar reweighting triplet loss,ground view query image,in-batch reweighting triplet loss,end-to-end training,feature-level contextual information,lower bound constraints,upper bound constraints,modified logistic regression,distance rectifying factor
AI 理解论文
溯源树
样例
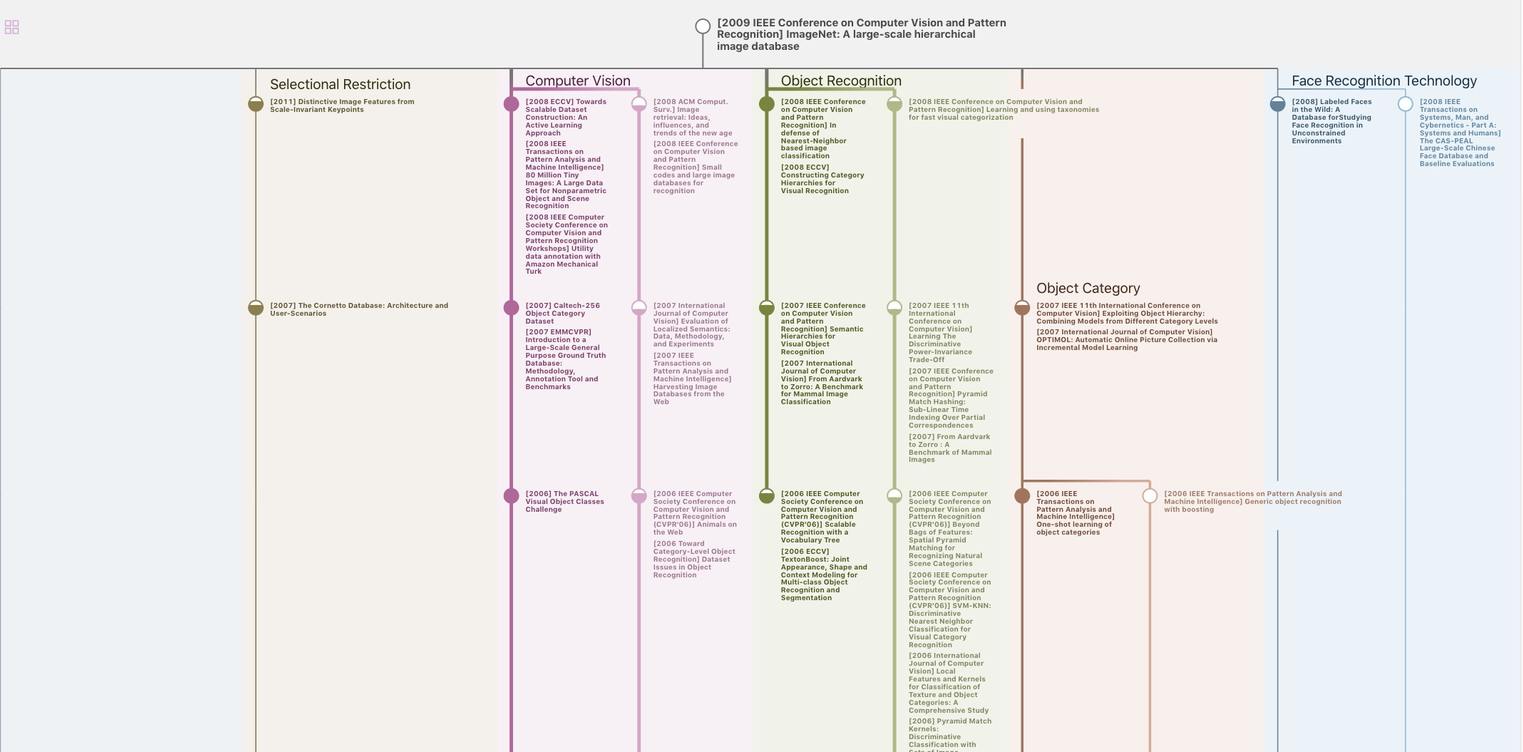
生成溯源树,研究论文发展脉络
Chat Paper
正在生成论文摘要