A Robust Embedding For Attributed Networks With Outliers
NEURAL INFORMATION PROCESSING (ICONIP 2019), PT IV(2019)
Abstract
Network embedding, as a promising tool, aims to learn low-dimensional embeddings for nodes in a network. Most existing methods work well when the topological structure is closely correlated to node attributes. However, real-world networks often contain outliers that have abnormal attributes. These attributes are quite different from the properties of their neighboring nodes, and they are not consistent with network structure. Thus, outliers exert negative impacts on the learned embeddings. Several methods only obtain unsatisfied results as they don't consider the effects of outliers. Hence, how to eliminate outlier impacts is essential for network embedding. In this paper, we propose a novel method called REANO for learning a Roust Embedding for an Attributed Network with Outliers. An overview of REANO combines residual analysis with attributed network embedding. In detail, residual analysis smooths out the negative impacts from outliers. Meanwhile, network embedding aggregates node attributes with network structure by using deep neural networks. By developing a joint optimization framework, REANO effectively alleviates outlier effects on the learned embeddings, and improve the robustness of node embeddings. Experiments on real-world datasets and manually planted outliers show that REANO learns more robust embeddings of nodes than the state-of-the-art algorithms.
MoreTranslated text
Key words
Network outliers, Network embedding, Attributed networks
AI Read Science
Must-Reading Tree
Example
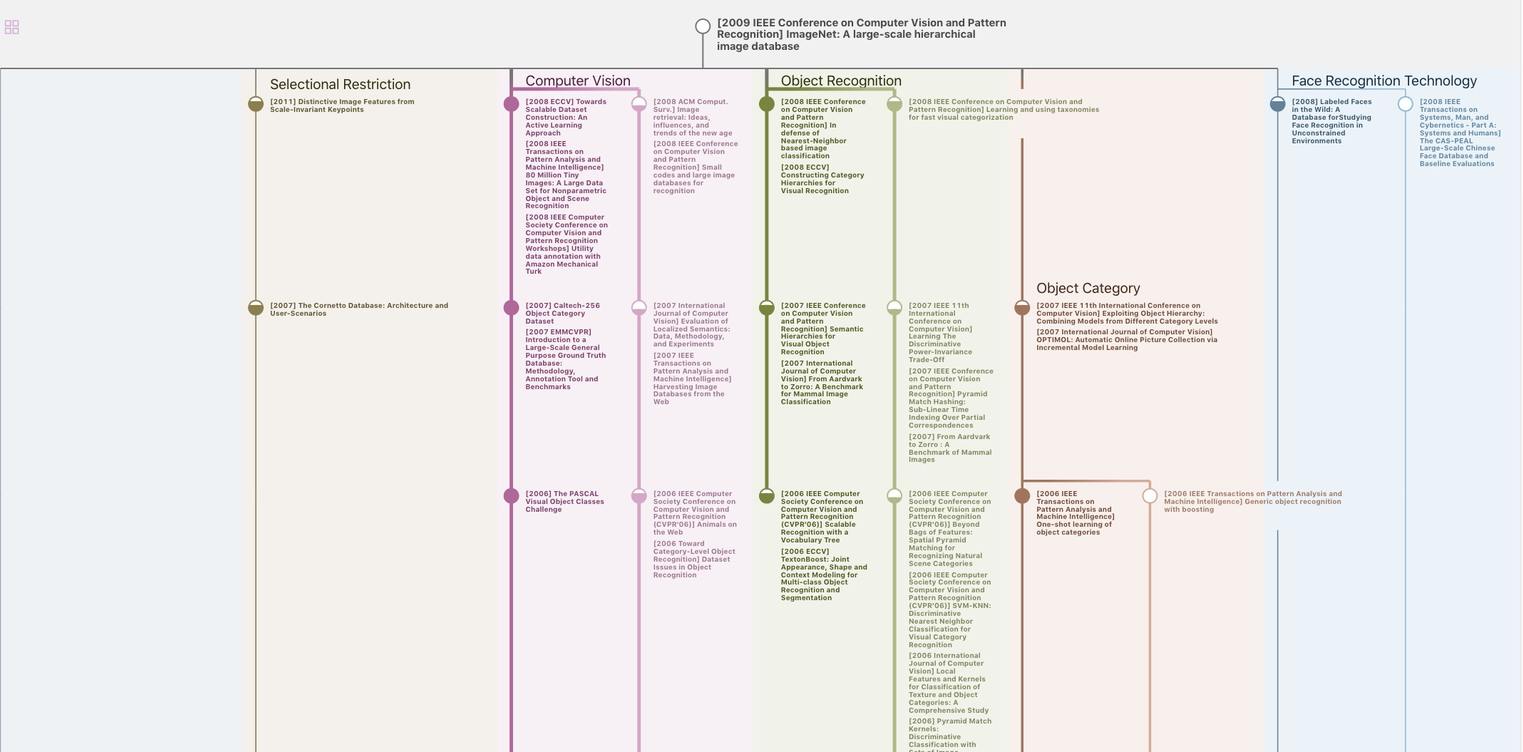
Generate MRT to find the research sequence of this paper
Chat Paper
Summary is being generated by the instructions you defined