Learning Network Representation Via Ego-Network-Level Relationship
NEURAL INFORMATION PROCESSING (ICONIP 2019), PT IV(2019)
摘要
Network representation, aiming to map each node of a network into a low-dimensional space, is a fundamental problem in the network analysis. Most existing works focus on the self-level or pairwise-level relationship among nodes to capture network structure. However, it is too simple to characterize the complex dependencies in the network. In this paper, we introduce the theory of the ego network and present an ego-network-level relationship. Then a deep recurrent auto-encoder model is proposed to preserve the complex dependencies in each ego network. In addition, we present two strategies to solve the sparsity problem. Finally, we conduct extensive experiments on three real datasets. The experimental results demonstrate that the proposed model can well preserve network structure and learn a good network representation.
更多查看译文
关键词
Network representation, Ego-network-level relationship, Recurrent auto-encoder
AI 理解论文
溯源树
样例
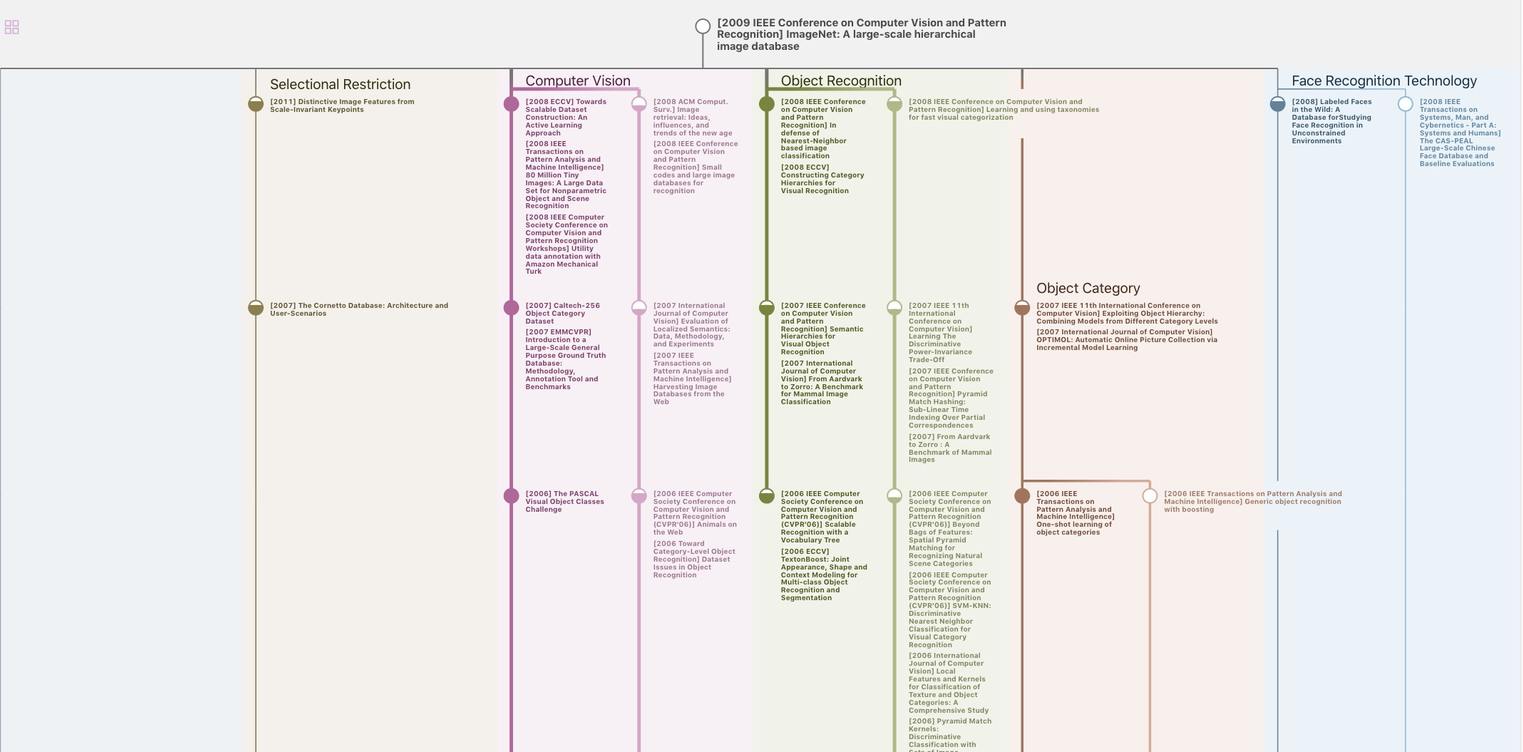
生成溯源树,研究论文发展脉络
Chat Paper
正在生成论文摘要