Multiscale Representation of Observation Error Statistics in Data Assimilation
SENSORS(2020)
摘要
Accounting for realistic observation errors is a known bottleneck in data assimilation, because dealing with error correlations is complex. Following a previous study on this subject, we propose to use multiscale modelling, more precisely wavelet transform, to address this question. This study aims to investigate the problem further by addressing two issues arising in real-life data assimilation: how to deal with partially missing data (e.g., concealed by an obstacle between the sensor and the observed system), and how to solve convergence issues associated with complex observation error covariance matrices? Two adjustments relying on wavelets modelling are proposed to deal with those, and offer significant improvements. The first one consists of adjusting the variance coefficients in the frequency domain to account for masked information. The second one consists of a gradual assimilation of frequencies. Both of these fully rely on the multiscale properties associated with wavelet covariance modelling. Numerical results on twin experiments show that multiscale modelling is a promising tool to account for correlations in observation errors in realistic applications.
更多查看译文
关键词
data assimilation,observation errors,error correlation,multiscale analysis,wavelets,error covariance matrices
AI 理解论文
溯源树
样例
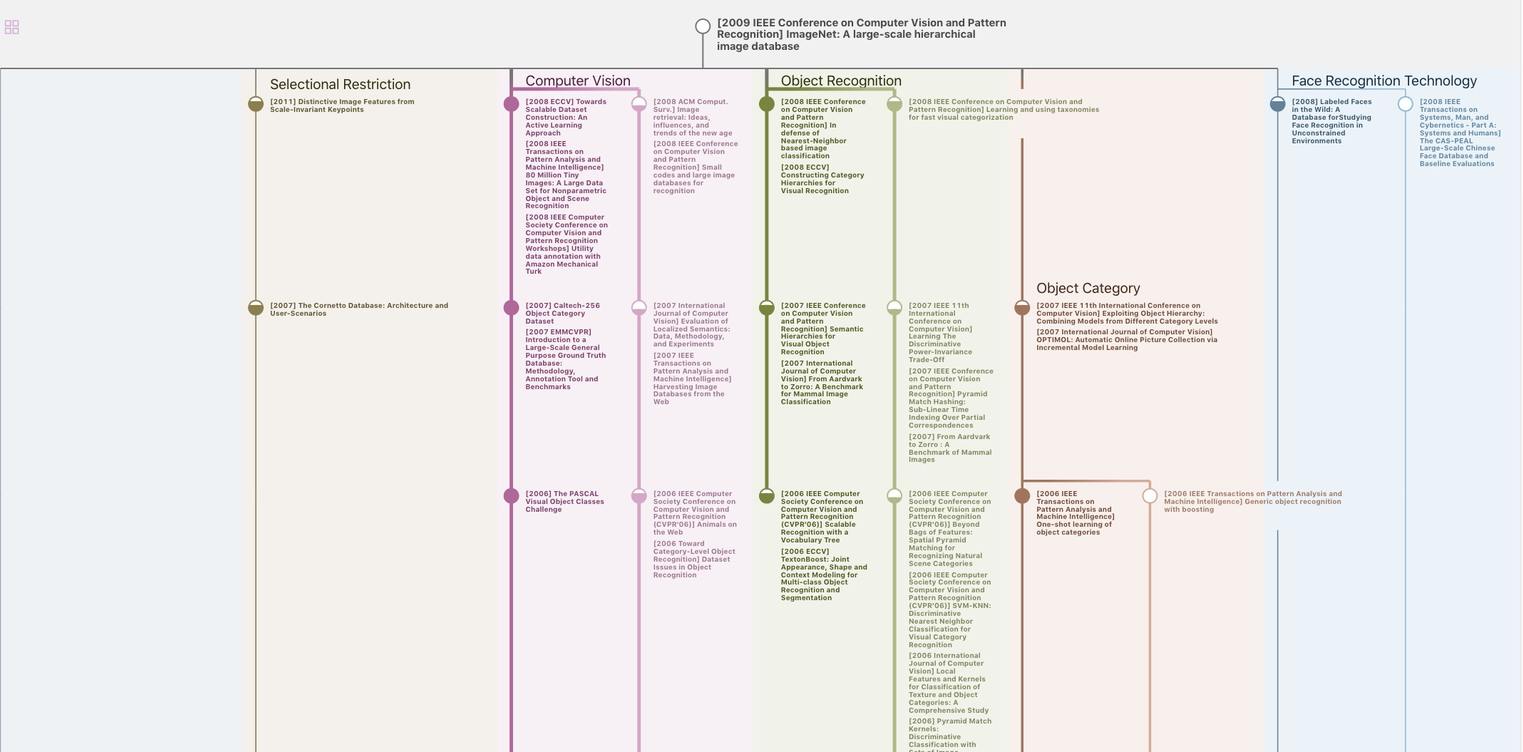
生成溯源树,研究论文发展脉络
Chat Paper
正在生成论文摘要