Model Aggregation Method for Data Parallelism in Distributed Real-Time Machine Learning of Smart Sensing Equipment
IEEE Access(2019)
摘要
In distributed real-time machine learning of smart sensing equipment, training speed and training accuracy are two hard-to-choose trade-off performance measures directly influenced by the design of distributed machine learning algorithms. And it will influence effort of smart sensing equipment directly. We take the model aggregation method of distributed machine learning as a starting point. Due to the loss of accuracy caused by the direct averaging of the parameter average method, we developed the loss function weight reorder stochastic gradient descent method (LR-SGD). LR-SGD uses the loss function value to determine the weight of the work nodes when aggregating the model parameters, and it improves the performance of the parameter average method for nonconvex problems. As shown in the experiment results, our algorithm can improve the training accuracy by a maximum of approximately 0.57% for the Bulk Synchronous Parallel (BSP) model and approximately 6.30% for the Stale Synchronous Parallel (SSP) model.
更多查看译文
关键词
Distributed machine learning,stochastic gradient descent,model aggregation method,smart sensing equipment
AI 理解论文
溯源树
样例
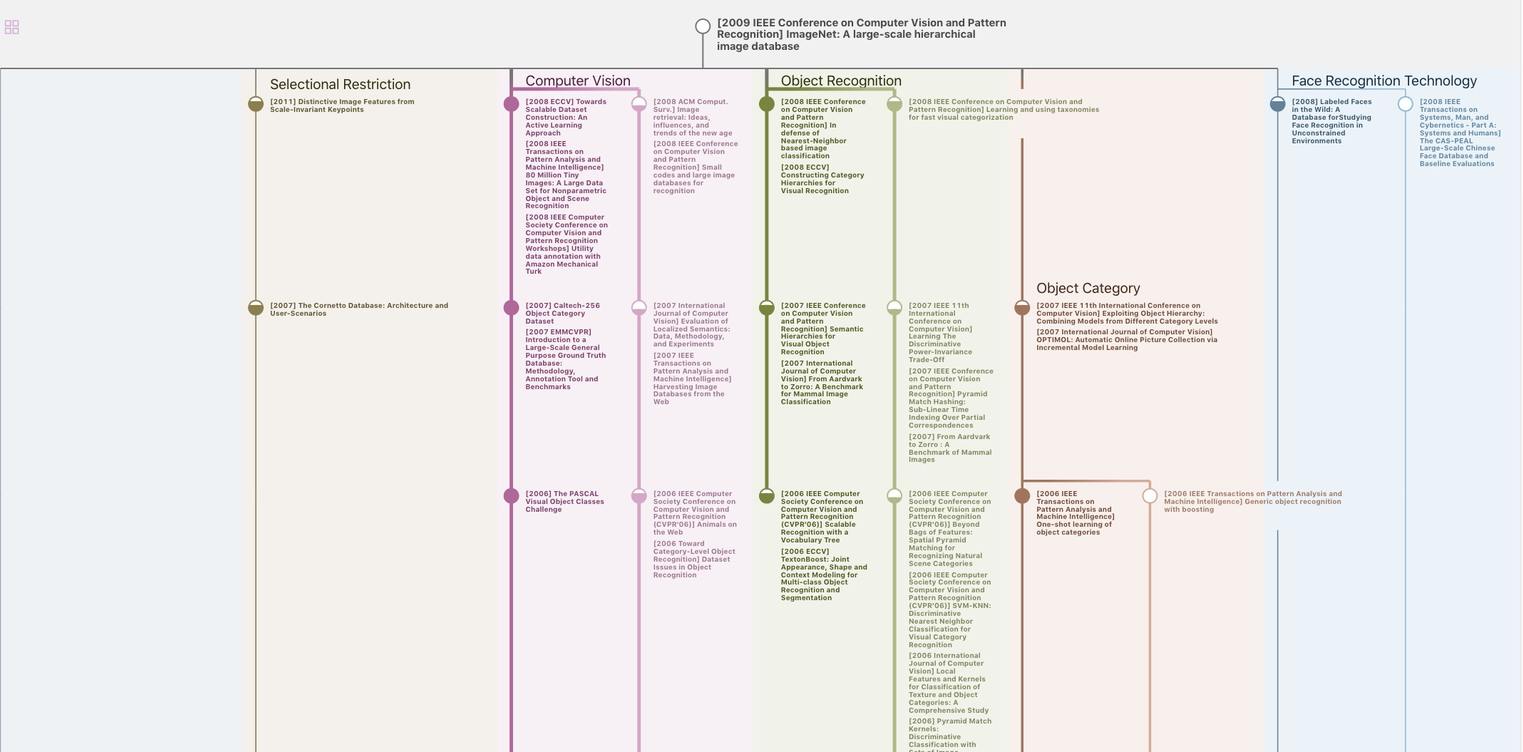
生成溯源树,研究论文发展脉络
Chat Paper
正在生成论文摘要