A Machine-Learning Model For Zonal Ship Flow Prediction Using Ais Data: A Case Study In The South Atlantic States Region
JOURNAL OF MARINE SCIENCE AND ENGINEERING(2019)
摘要
Predicting traffic flow is critical in efficient maritime transportation management, coordination, and planning. Scientists have proposed many prediction methods, most of which are designed for specific locations or for short-term prediction. For the purpose of management, methods that enable long-term prediction for large areas are highly desirable. Therefore, we propose developing a spatiotemporal approach that can describe and predict traffic flows within a region. We designed the model based on a multiple hexagon-based convolutional neural network (mh-CNN) model that takes both the flow dynamics and environmental conditions into account. This model is highly flexible in that it predicts zonal traffic flow within variable time windows. We applied the method to measure and predict the daily and hourly traffic flows in the South Atlantic States region by taking the impacts of extreme weather events into consideration. Results show that our method outperformed other methods in daily prediction during normal days and hourly prediction during hurricane events. Based on the results, we also provide some recommendations regarding the future usage and customization of the model.
更多查看译文
关键词
traffic flow prediction,deep learning,spatiotemporal modeling,extreme weather
AI 理解论文
溯源树
样例
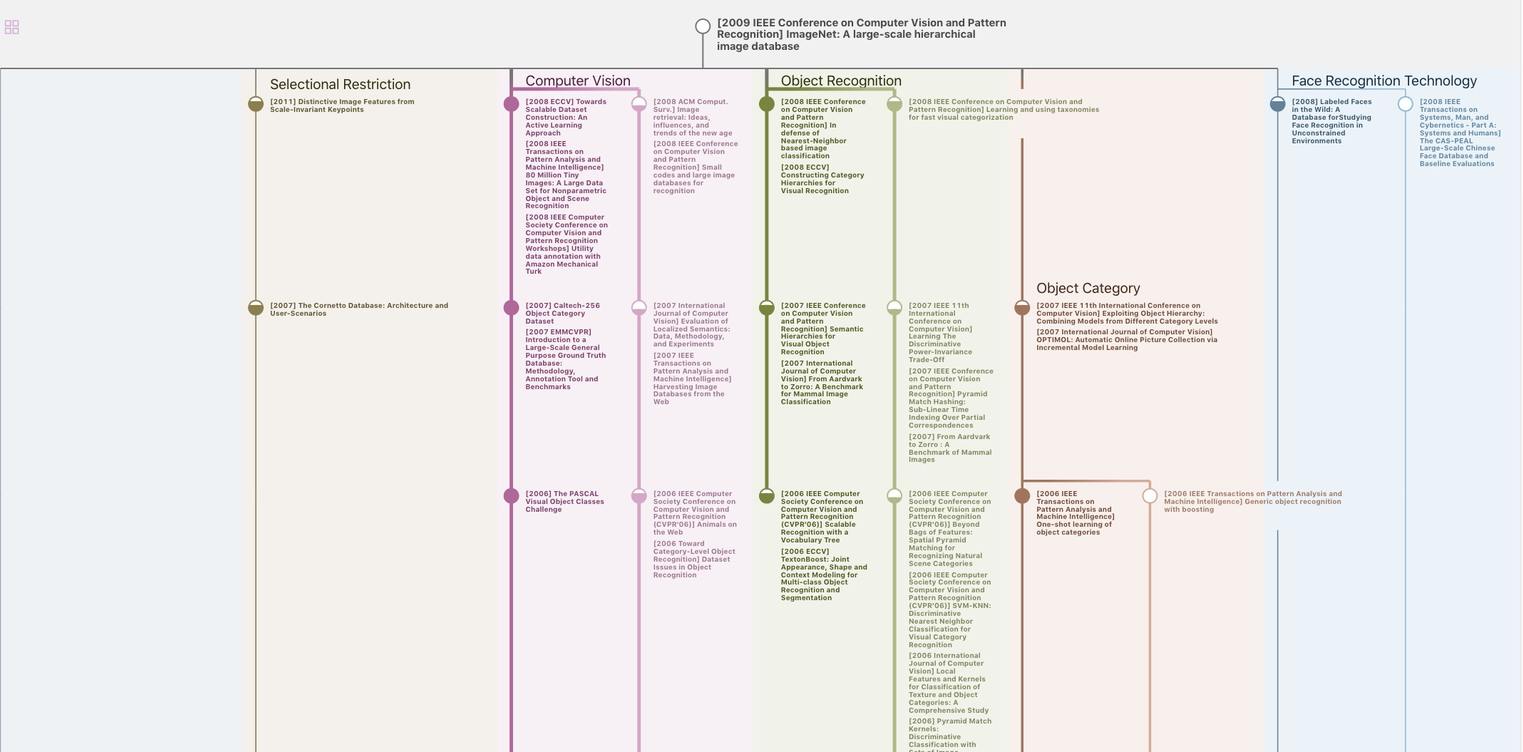
生成溯源树,研究论文发展脉络
Chat Paper
正在生成论文摘要