Reducing The Subject Variability Of Eeg Signals With Adversarial Domain Generalization
NEURAL INFORMATION PROCESSING (ICONIP 2019), PT I(2019)
摘要
A major obstacle in generalizing brain-computer interface (BCI) systems to previously unseen subjects is the subject variability of electroencephalography (EEG) signals. To deal with this problem, the existing methods focus on domain adaptation with subject-specific EEG data, which are expensive and time consuming to collect. In this paper, domain generalization methods are introduced to reduce the influence of subject variability in BCI systems without requiring any information from unseen subjects. We first modify a deep adversarial network for domain generalization and then propose a novel adversarial domain generalization framework, DResNet, in which domain information is utilized to learn two components of weights: unbiased weights that are common across subjects and biased weights that are subject-specific. Experimental results on two public EEG datasets indicate that our proposed methods can achieve a performance comparable to and more stable than that of the state-of-the-art domain adaptation method. In contrast to existing domain adaptation methods, our proposed domain generalization approach does not require any data from test subjects and can simultaneously generalize well to multiple test subjects.
更多查看译文
关键词
Brain-computer interface, EEG subject variability, Domain adaptation, Domain generalization, Domain residual network, Emotion recognition, Vigilance estimation
AI 理解论文
溯源树
样例
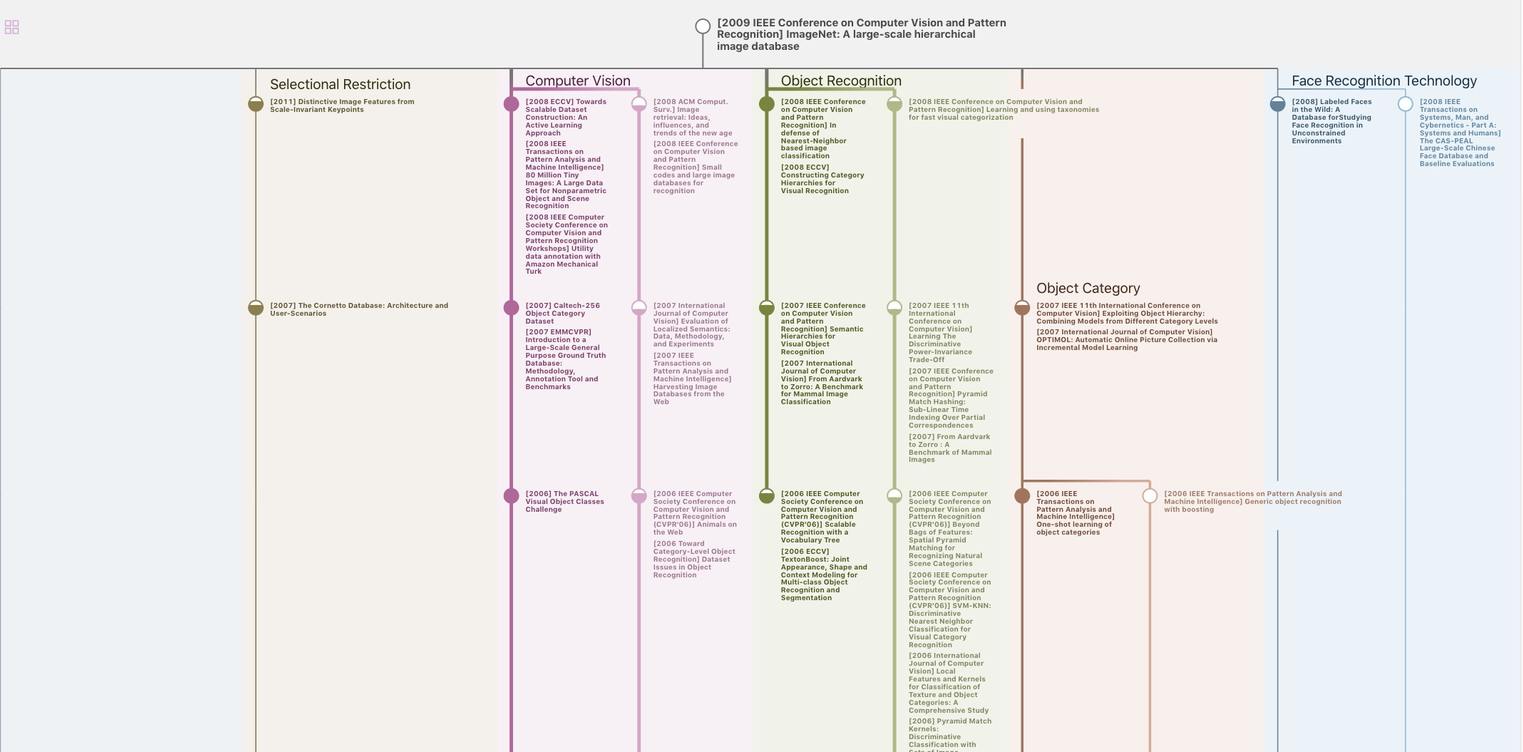
生成溯源树,研究论文发展脉络
Chat Paper
正在生成论文摘要