Multi-modal sequence model with gated fully convolutional blocks for micro-video venue classification
Multimedia Tools and Applications(2019)
摘要
With the large amount of micro-videos available in social network applications, micro-video venue category provides extremely valuable venue information that assists location-oriented applications, personalized services, etc. In this paper, we formulate micro-video venue classification as a multi-modal sequential modeling problem. Unlike existing approaches that use long short-term memory (LSTM) models to capture temporal patterns for micro-video, we propose multi-modality sequence model with gated fully convolutional blocks. Specifically, we firstly adopt three parallel gated fully convolutional blocks to extract spatiotemporal features from visual, acoustic and textual modalities of micro-videos. Then, an additional gated fully convolutional block is used to fuse such three modalities of spatiotemporal features. Finally, corresponding prototype is simultaneously learned to improve the robustness against softmax classification function. Extensive experimental results on a real-world benchmark dataset demonstrate the effectiveness of our model in terms of both Micro-F and Macro-F scores.
更多查看译文
关键词
Micro-video venue classification,Gated fully convolutional block,Multi-modal sequence model,Prototype learning
AI 理解论文
溯源树
样例
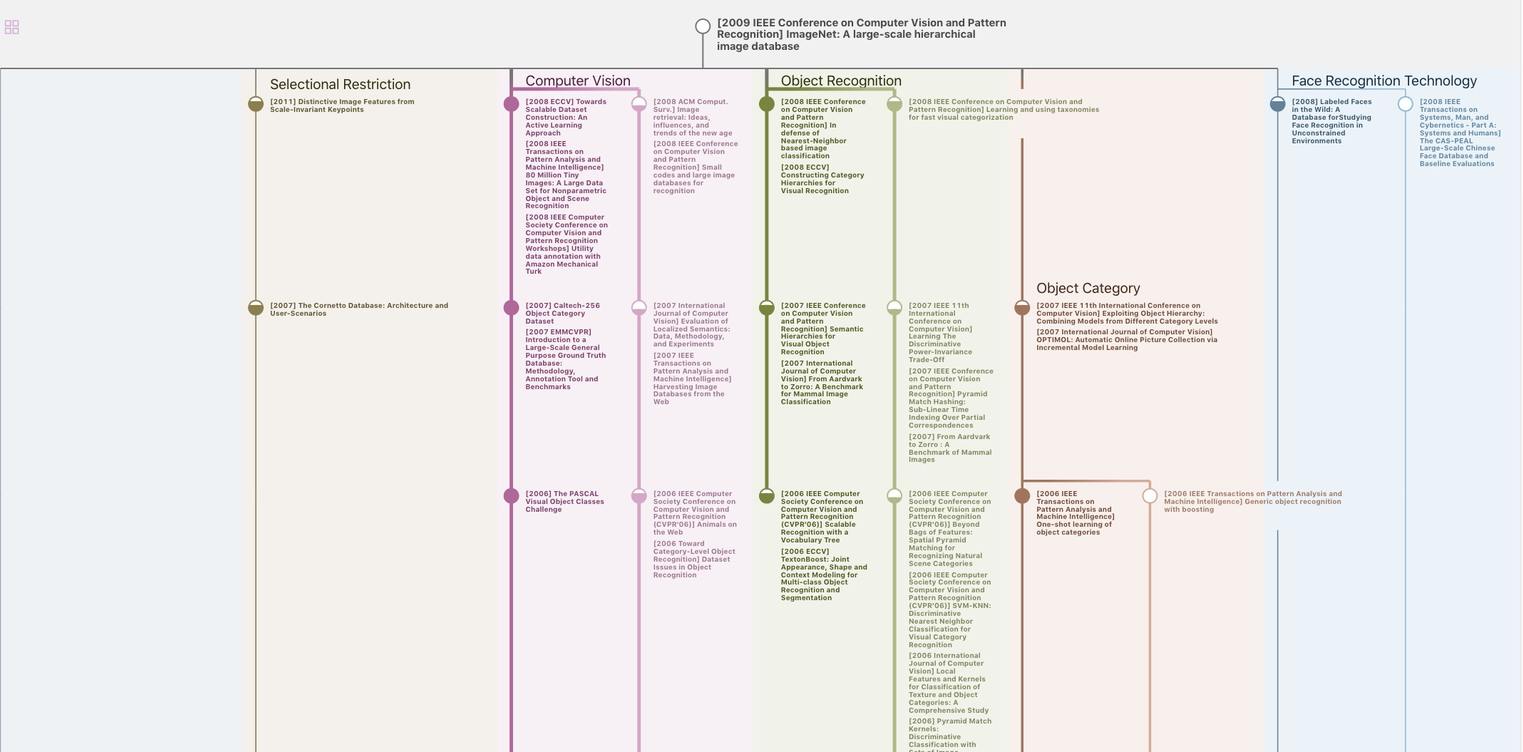
生成溯源树,研究论文发展脉络
Chat Paper
正在生成论文摘要