Enhancing network intrusion detection classifiers using supervised adversarial training
The Journal of Supercomputing(2019)
摘要
The performance of classifiers has a direct impact on the effectiveness of intrusion detection system. Thus, most researchers aim to improve the detection performance of classifiers. However, classifiers can only get limited useful information from the limited number of labeled training samples, which usually affects the generalization of classifiers. In order to enhance the network intrusion detection classifiers, we resort to adversarial training, and a novel supervised learning framework using generative adversarial network for improving the performance of the classifier is proposed in this paper. The generative model in our framework is utilized to continuously generate other complementary labeled samples for adversarial training and assist the classifier for classification, while the classifier in our framework is used to identify different categories. Meanwhile, the loss function is deduced again, and several empirical training strategies are proposed to improve the stabilization of the supervised learning framework. Experimental results prove that the classifier via adversarial training improves the performance indicators of intrusion detection. The proposed framework provides a feasible method to enhance the performance and generalization of the classifier.
更多查看译文
关键词
Intrusion detection,Generative adversarial networks,Artificial neural network,Adversarial training,Machine learning
AI 理解论文
溯源树
样例
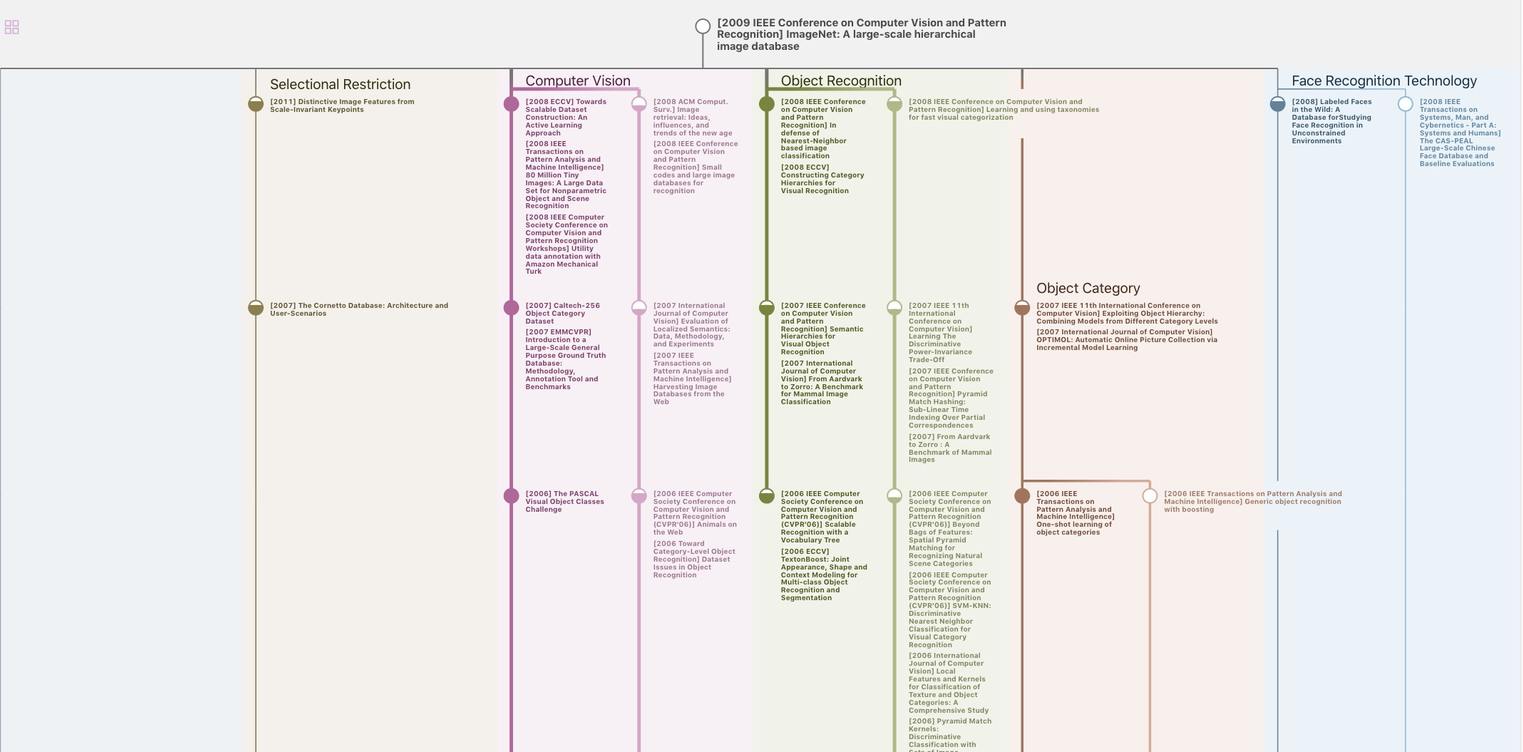
生成溯源树,研究论文发展脉络
Chat Paper
正在生成论文摘要