Dynamic Neural Language Models
NEURAL INFORMATION PROCESSING (ICONIP 2019), PT III(2019)
摘要
Language evolves over time with trends and shifts in technological, political, or cultural contexts. Capturing these variations is important to develop better language models. While recent works tackle temporal drifts by learning diachronic embeddings, we instead propose to integrate a temporal component into a recurrent language model. It takes the form of global latent variables, which are structured in time by a learned non-linear transition function. We perform experiments on three time-annotated corpora. Experimental results on language modeling and classification tasks show that our model performs consistently better than temporal word embedding methods in two temporal evaluation settings: prediction and modeling. Moreover, we empirically show that the system is able to predict informative latent representations in the future.
更多查看译文
关键词
Temporal modelling, Language model, RNN
AI 理解论文
溯源树
样例
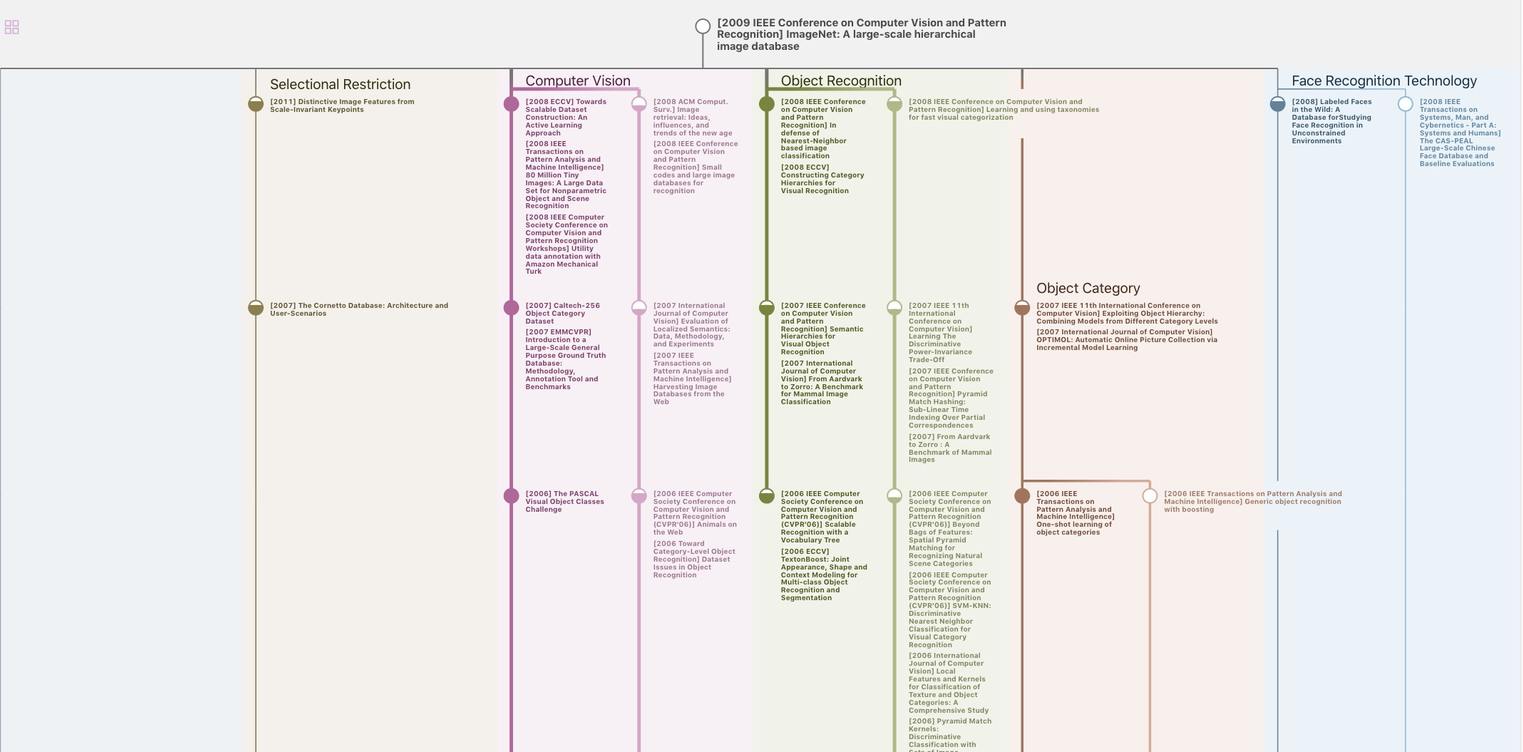
生成溯源树,研究论文发展脉络
Chat Paper
正在生成论文摘要