StructPool: Structured Graph Pooling via Conditional Random Fields
ICLR(2020)
摘要
Learning high-level representations for graphs is of great importance for graph analysis tasks. In addition to graph convolution, graph pooling is an important but less explored research area. In particular, most of existing graph pooling techniques do not consider the graph structural information explicitly. We argue that such information is important and develop a novel graph pooling technique, know as the StructPool, in this work. We consider the graph pooling as a node clustering problem, which requires the learning of a cluster assignment matrix. We propose to formulate it as a structured prediction problem and employ conditional random fields to capture the relationships among assignments of different nodes. We also generalize our method to incorporate graph topological information in designing the Gibbs energy function. Experimental results on multiple datasets demonstrate the effectiveness of our proposed StructPool.
更多查看译文
关键词
Graph Pooling, Representation Learning, Graph Analysis
AI 理解论文
溯源树
样例
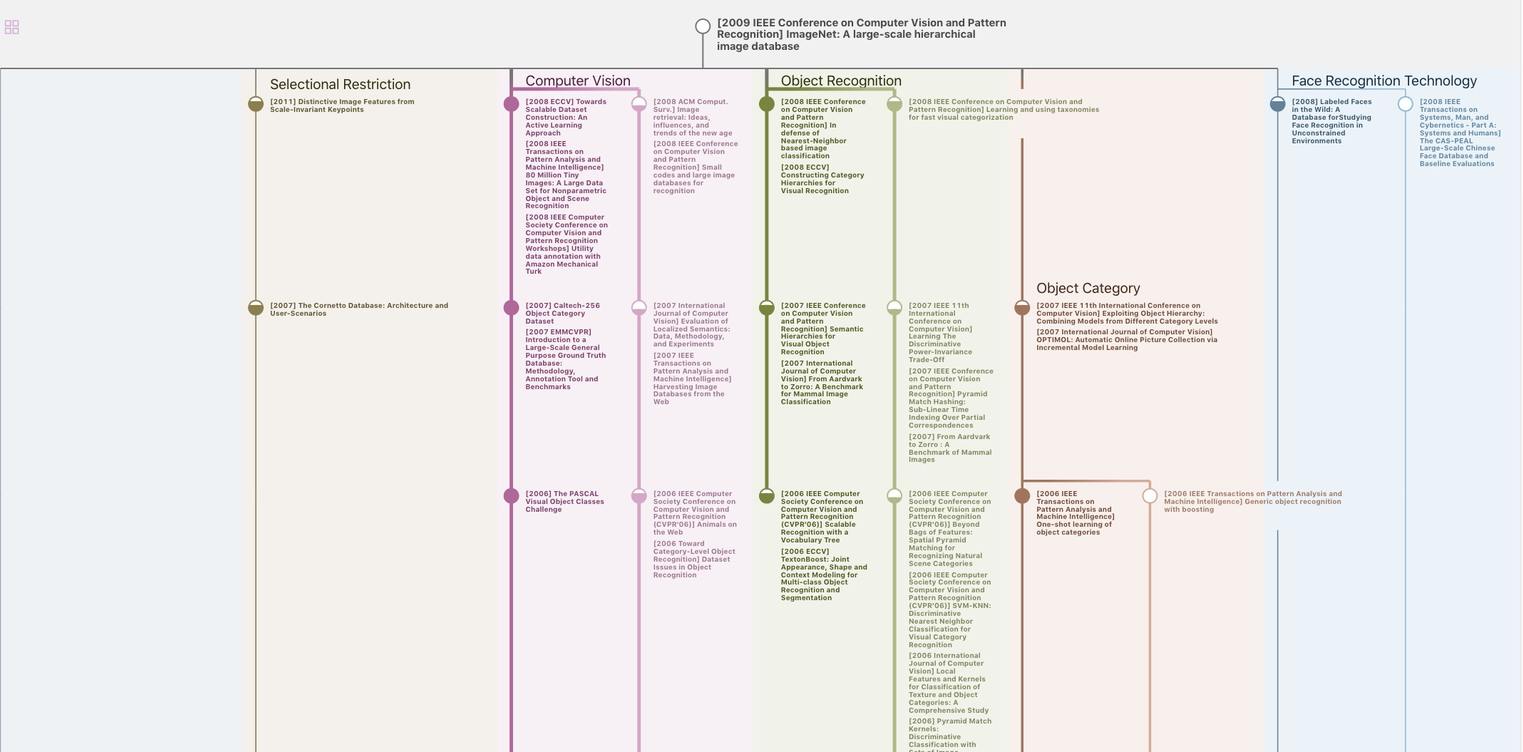
生成溯源树,研究论文发展脉络
Chat Paper
正在生成论文摘要