Automatic Kidney CT Segmentation and Optimization Based on Self-Learning
2019 WRC Symposium on Advanced Robotics and Automation (WRC SARA)(2019)
摘要
Medical image processing plays an increasingly important role in clinical diagnosis and treatment. Using the results of kidney CT image segmentation for three-dimensional reconstruction is an intuitive and accurate method for diagnosis. However, the performance of traditional image segmentation algorithms is unsatisfactory due to the large difference between the kidneys of patients and the noise of CT images, and the surface of the model reconstructed will be rough and deformed when the results are directly used for three-dimensional reconstruction. To improve this situation, we propose a segmentation, reconstruction and optimization system(SROS), which combines the auto segmentation of kidney CT sequence images and the optimization of three-dimensional reconstruction. According to the characteristics of U-Net, we improved it to be suitable for accomplishing our task. Firstly, we initialize the size and gray value range of CT image sequence. Secondly, we use sequence images and label images to train the network model. Thirdly, we use the trained network model to segment the sequence images. Finally, we use the model to optimize the reconstruction. The experimental results show that SROS has a good performance in terms of 3D reconstruction accuracy, smoothness and robustness.
更多查看译文
关键词
medical image processing,deep learning
AI 理解论文
溯源树
样例
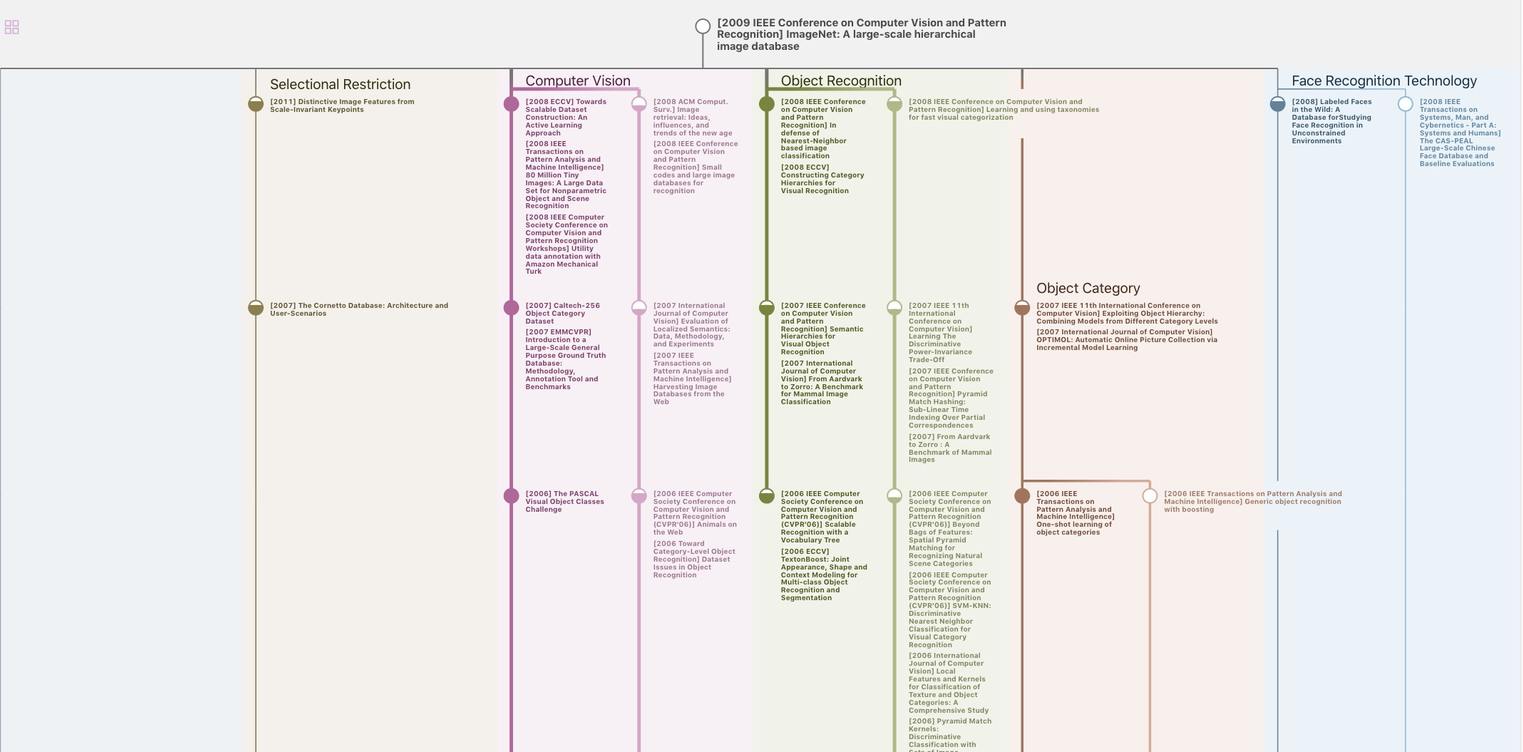
生成溯源树,研究论文发展脉络
Chat Paper
正在生成论文摘要