Gabor-Cnn For Object Detection Based On Small Samples
DEFENCE TECHNOLOGY(2020)
摘要
Object detection models based on convolutional neural networks (CNN) have achieved state-of-the-art performance by heavily rely on large-scale training samples. They are insufficient when used in specific applications, such as the detection of military objects, as in these instances, a large number of samples is hard to obtain. In order to solve this problem, this paper proposes the use of Gabor-CNN for object detection based on a small number of samples. First of all, a feature extraction convolution kernel library composed of multi-shape Gabor and color Gabor is constructed, and the optimal Gabor convolution kernel group is obtained by means of training and screening, which is convolved with the input image to obtain feature information of objects with strong auxiliary function. Then, the k-means clustering algorithm is adopted to construct several different sizes of anchor boxes, which improves the quality of the regional proposals. We call this regional proposal process the Gabor-assisted Region Proposal Network (Gabor-assisted RPN). Finally, the Deeply-Utilized Feature Pyramid Network (DU-FPN) method is proposed to strengthen the feature expression of objects in the image. A bottom-up and a top-down feature pyramid is constructed in ResNet-50 and feature information of objects is deeply utilized through the transverse connection and integration of features at various scales. Experimental results show that the method proposed in this paper achieves better results than the state-of-art contrast models on data sets with small samples in terms of accuracy and recall rate, and thus has a strong application prospect. Copyright (C) 2020 China Ordnance Society. Publishing Services by Elsevier B.V. on behalf of KeAi Communications Co. Ltd.
更多查看译文
关键词
Deep learning, Convolutional neural network, Small samples, Gabor convolution kernel, Feature pyramid
AI 理解论文
溯源树
样例
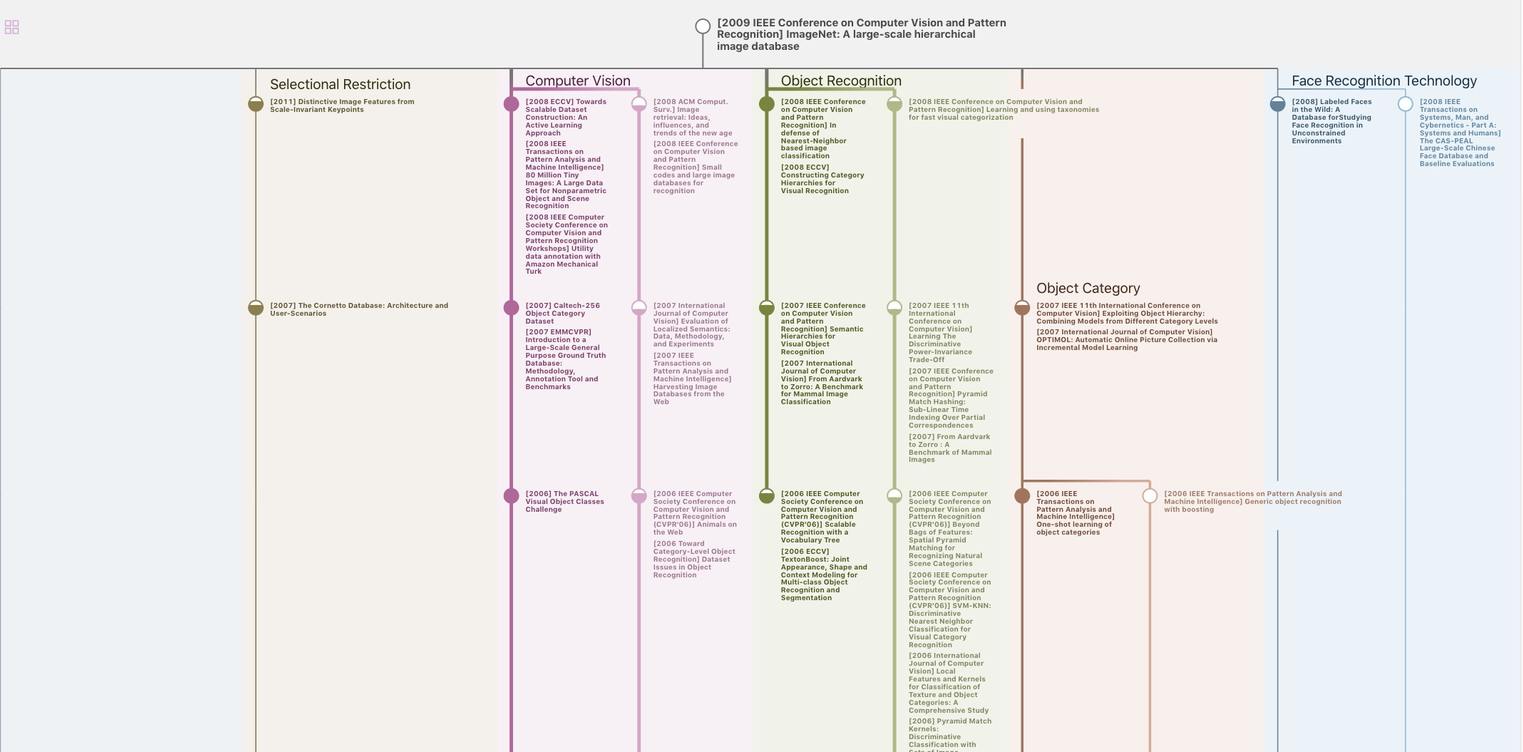
生成溯源树,研究论文发展脉络
Chat Paper
正在生成论文摘要