An Explicit and Scene-Adapted Definition of Convex Self-Similarity Prior With Application to Unsupervised Sentinel-2 Super-Resolution
IEEE Transactions on Geoscience and Remote Sensing(2020)
摘要
Sentinel-2 satellite, launched by the European Space Agency, plays a critical role in various Earth observation missions. However, the spatial resolutions of Sentinel-2 images are different across its spectral bands, including four bands with a resolution of 10 m, six bands with a resolution of 20 m, and three bands with a resolution of 60 m. To facilitate the effectiveness of analyzing these images, super-resolving of the low-/medium-resolution bands to a higher resolution is desired. As in any image restoration inverse problems, we exploit image self-similarity, a commonly observed property in natural images, which underlies the state-of-the-art techniques, e.g., in image denoising. However, the design of self-similarity priors in nondiagonal inverse problems is challenging; often, a denoiser based on self-similarity is plugged into the iterations of an algorithm, without a guarantee of convergence in general. In this article, for the first time, we introduce a convex and scene-adapted regularizer built explicitly on a self-similarity graph directly learned from the Sentinel-2 images. We then develop a fast algorithm, termed Sentinel-2 super-resolution via scene-adapted self-similarity (SSSS). We experimentally show the superiority of SSSS over four commonly observed scenes, indicating the potential usage of our convex self-similarity regularization in other imaging inverse problems.
更多查看译文
关键词
Convex optimization,scene-adapted self-similarity,self-similarity,Sentinel-2 satellite,super-resolution
AI 理解论文
溯源树
样例
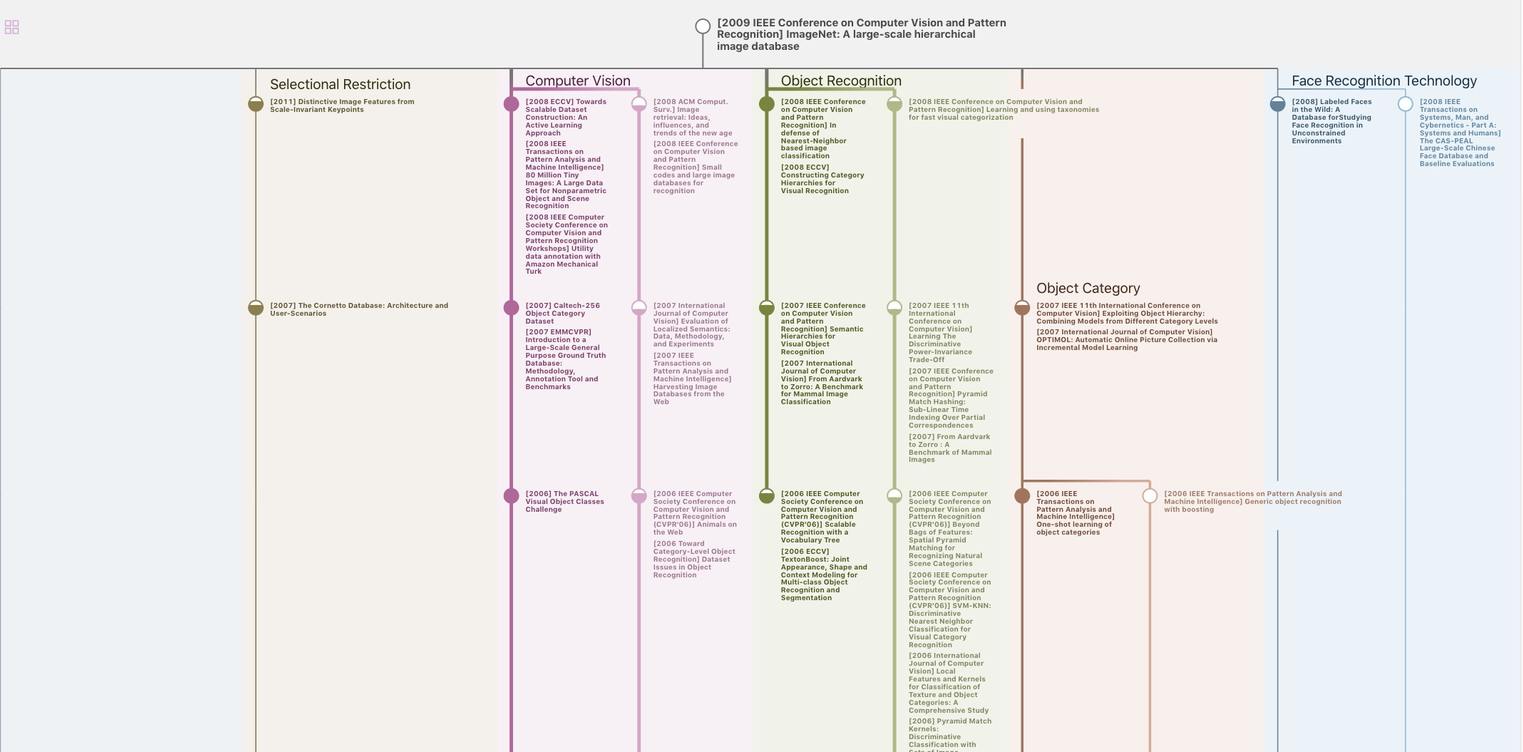
生成溯源树,研究论文发展脉络
Chat Paper
正在生成论文摘要