Multimodal Spatiotemporal Networks for Sign Language Recognition
IEEE access(2019)
摘要
Different from other human behaviors, sign language has the characteristics of limited local motion of upper limb and meticulous hand action. Some sign language gestures are ambiguous in RGB video due to the influence of lighting and background color, which affects the recognition accuracy. We propose a multimodal deep learning architecture for sign language recognition which effectively combines RGB-D input and two-stream spatiotemporal networks. Depth videos, as an effective compensation of RGB input, can supply additional distance information about the signer's hands. A novel sampling method called ARSS (Aligned Random Sampling in Segments) is put forward to select and align optimal RGB-D video frames, which improves the capacity utilization of multimodal data and reduces the redundancy. We get the hand ROI by joints information of RGB data for local focus in spatial stream. D-shift Net is proposed as depth motion feature extraction in temporal stream, which fully utilizes three dimensional motion information of the sign language. Both streams are fused by convolutional fusion layer to get complementary features. Our approach explored the multimodal information and enhanced the recognition precision. It obtains the state-the-of-art performance on the datasets of CSL (96.7%) and IsoGD (63.78%).
更多查看译文
关键词
Sign language recognition,two-stream network,motion features,multimodal data
AI 理解论文
溯源树
样例
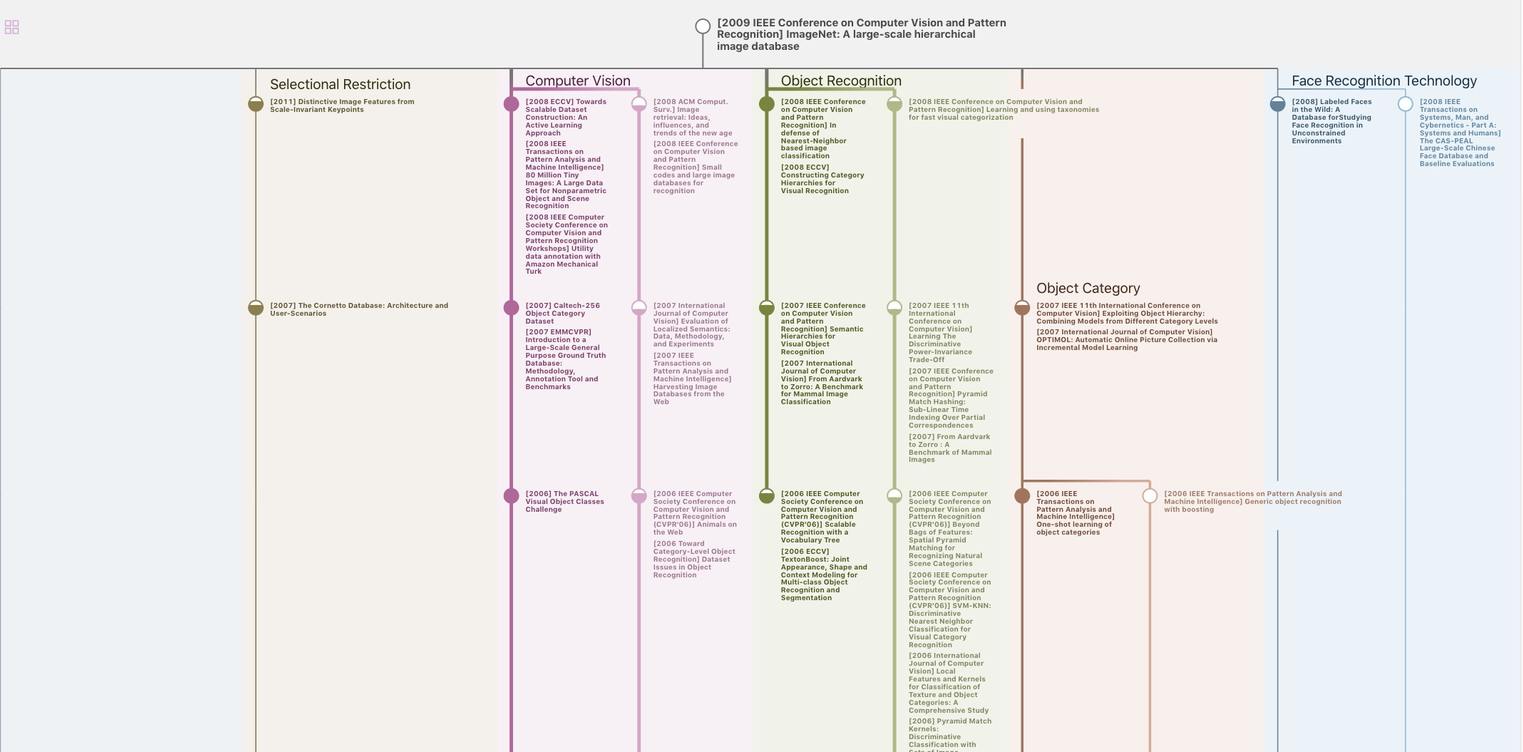
生成溯源树,研究论文发展脉络
Chat Paper
正在生成论文摘要