Neural network based analysis of random telegraph noise in resistive random access memories
SEMICONDUCTOR SCIENCE AND TECHNOLOGY(2020)
摘要
The characterization of random telegraph noise (RTN) signals in resistive random access memories (RRAM) is a challenge. The inherent stochastic operation of these devices, much different to what is seen in other electron devices such as MOSFETs, diodes, etc, makes this issue more complicated from the mathematical viewpoint. Nevertheless, the accurate modeling of these type of signals is essential for their use in digital and analog applications. RTN signals are revealed to be linked to the emission and capture of electrons by traps close to the conductive filament that can influence resistive switching operation in RRAMs. RTN features depend on the number of active traps, on the interaction between these traps at different times, on the occurrence of anomalous effects, etc Using a new representation technique, the locally weighted time lag plot (LWTLP), a highly efficient method in terms of computation, data from current-time (I-t) traces can be represented with a pattern that allows the analysis of important RTN signal features. In addition, self-organizing maps, a neural network devoted to clustering, can be employed to perform an automatic classification of the RTN traces that have similar LWTLP patterns. This pattern analysis allows a better understanding of RTN signals and the physics underlying them. The new technique presented can be performed in a reasonable computing time and it is particularly adequate for long (I-t) traces. We introduce here this technique and the most important results that can be drawn when applied to long RTN traces experimentally obtained in RRAMs.
更多查看译文
关键词
resistive memories,RRAM,RTN,LWTLP,neural network,self organizing maps,random telegraph noise
AI 理解论文
溯源树
样例
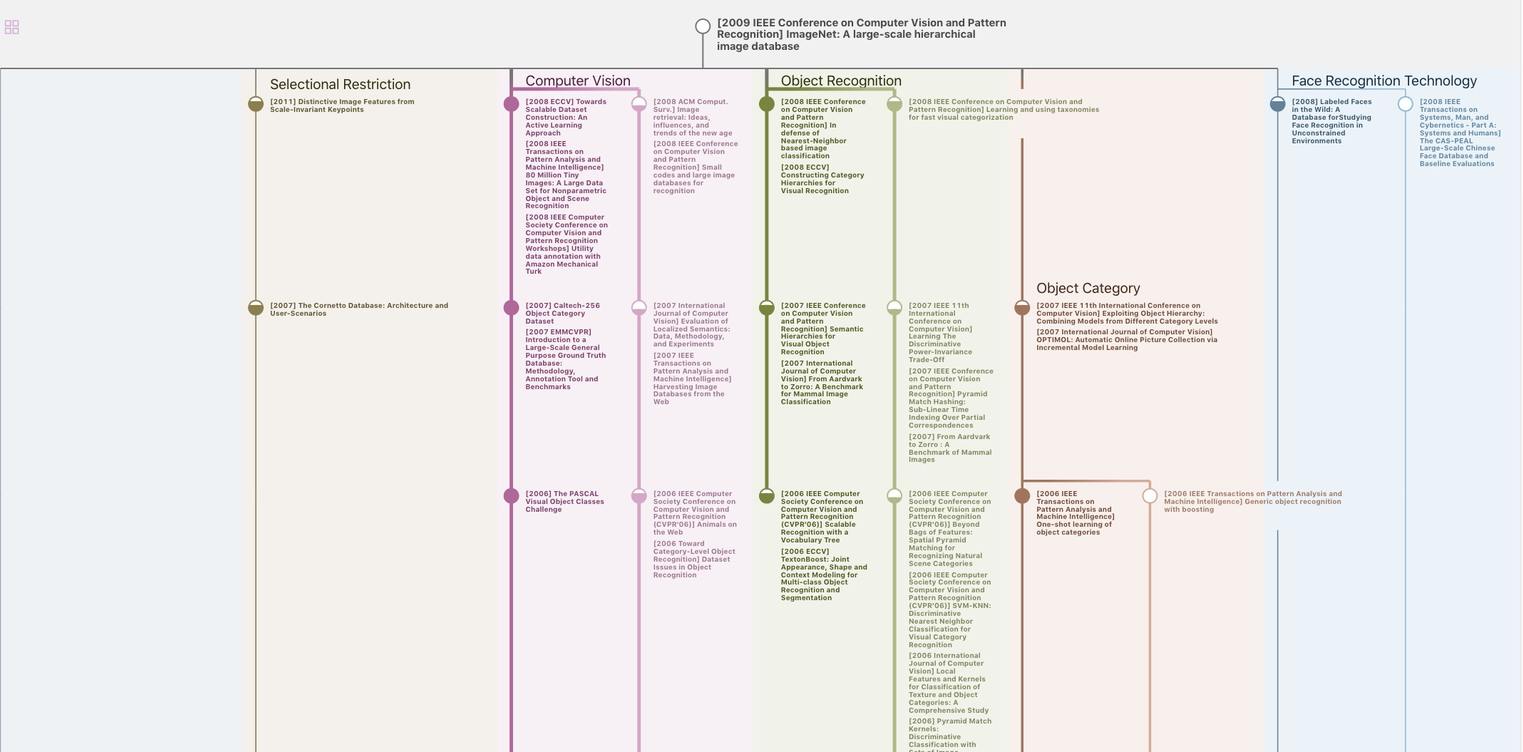
生成溯源树,研究论文发展脉络
Chat Paper
正在生成论文摘要