Improving The Efficiency Of Encoder-Decoder Architecture For Pixel-Level Crack Detection
IEEE ACCESS(2019)
摘要
Cracks are one of the most common categories of pavement distress that may potentially threaten road and highway safety. Thus, a reliable and efficient pixel-level method of crack detection is necessary for real-time measurement of the crack. However, many existing encoder-decoder architectures for crack detection are time-consuming because the part of decoder module always has lots of convolutional layers and feature channels that lead to performance that highly relies on computing resources, which is a handicap in scenarios with limited resources. In this study, we propose a simple and effective method to boost the algorithmic efficiency based on encoder-decoder architecture for crack detection. We develop a switch module, called SWM, to predict whether the image is positive or negative and then skip the decoder module to save computation time when it is negative. This method uses the encoder module as the fixed feature extractor and only needs to place a light-weight classifier head on the end of the encoder module to output the final class probability. We choose the classical UNet and DeepCrack as examples of the encoder-decoder architectures to show how SWM is integrated into the architectures to reduce computation complexity. Evaluations on the public CrackTree206 and AIMCrack datasets demonstrate that our method can significantly boost the efficiency of the encoder-decoder architectures in all tasks, while without affecting the performance. The SWM can also be easily embedded into other encoder-decoder architectures for further improvement. The source code is available at https://github.com/hanshenchen/crack-detection.
更多查看译文
关键词
Computer vision, deep learning, encoder-decoder architecture, crack detection
AI 理解论文
溯源树
样例
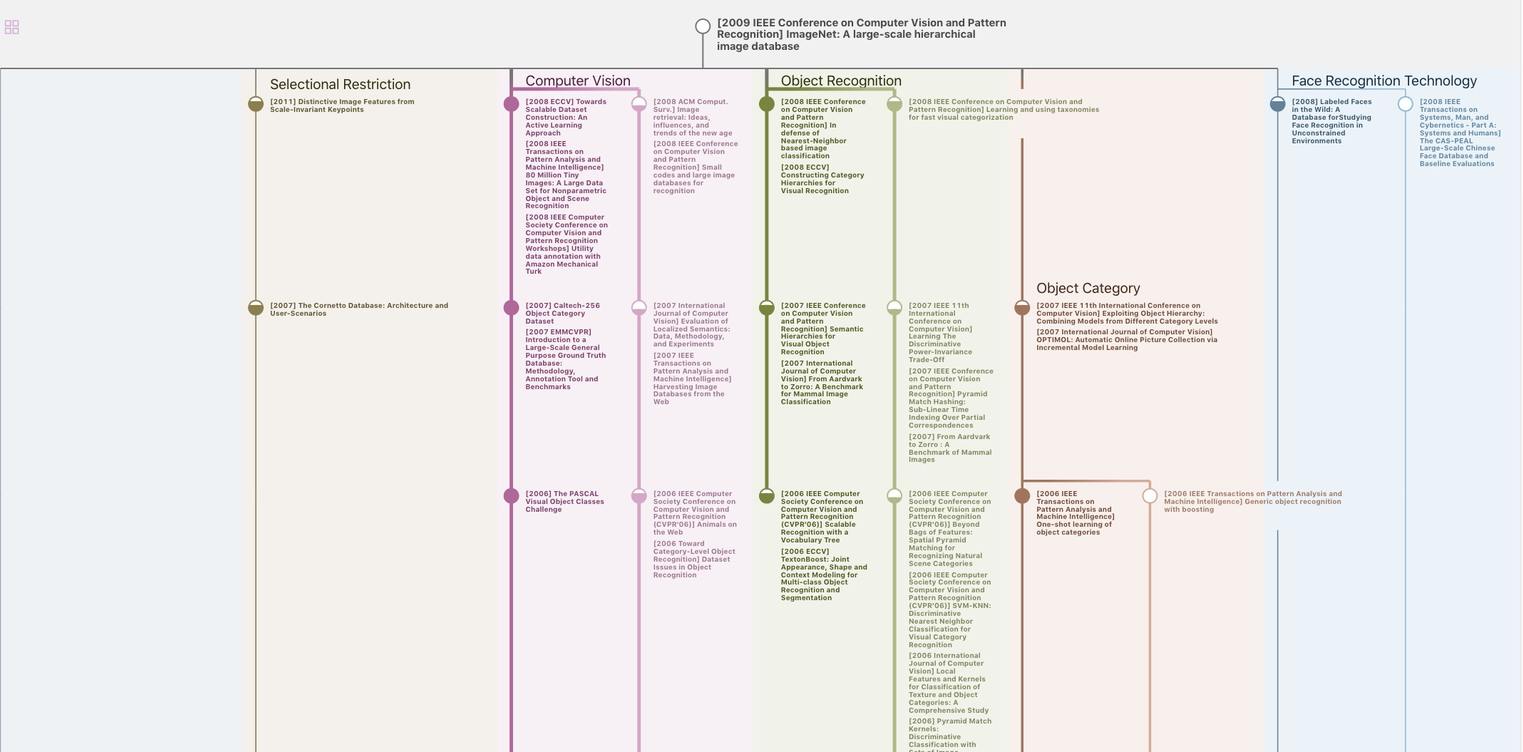
生成溯源树,研究论文发展脉络
Chat Paper
正在生成论文摘要