Revisiting Bilinear Pooling: A Coding Perspective
THIRTY-FOURTH AAAI CONFERENCE ON ARTIFICIAL INTELLIGENCE, THE THIRTY-SECOND INNOVATIVE APPLICATIONS OF ARTIFICIAL INTELLIGENCE CONFERENCE AND THE TENTH AAAI SYMPOSIUM ON EDUCATIONAL ADVANCES IN ARTIFICIAL INTELLIGENCE(2020)
摘要
Bilinear pooling has achieved state-of-the-art performance on fusing features in various machine learning tasks, owning to its ability to capture complex associations between features. Despite the success. bilinear pooling suffers from redundancy and burstiness issues, mainly due to the rank-one property of the resulting representation. In this paper, we prove that bilinear pooling is indeed a similarity-based coding-pooling formulation. This establishment then enables us to devise a new feature fusion algorithm, the factorized bilinear coding (FBC) method, to overcome the drawbacks of the bilinear pooling. We show that FBC can generate compact and discriminative representations with substantially fewer parameters. Experiments on two challenging tasks, namely image classification and visual question answering, demonstrate that our method surpasses the bilinear pooling technique by a large margin.
更多查看译文
AI 理解论文
溯源树
样例
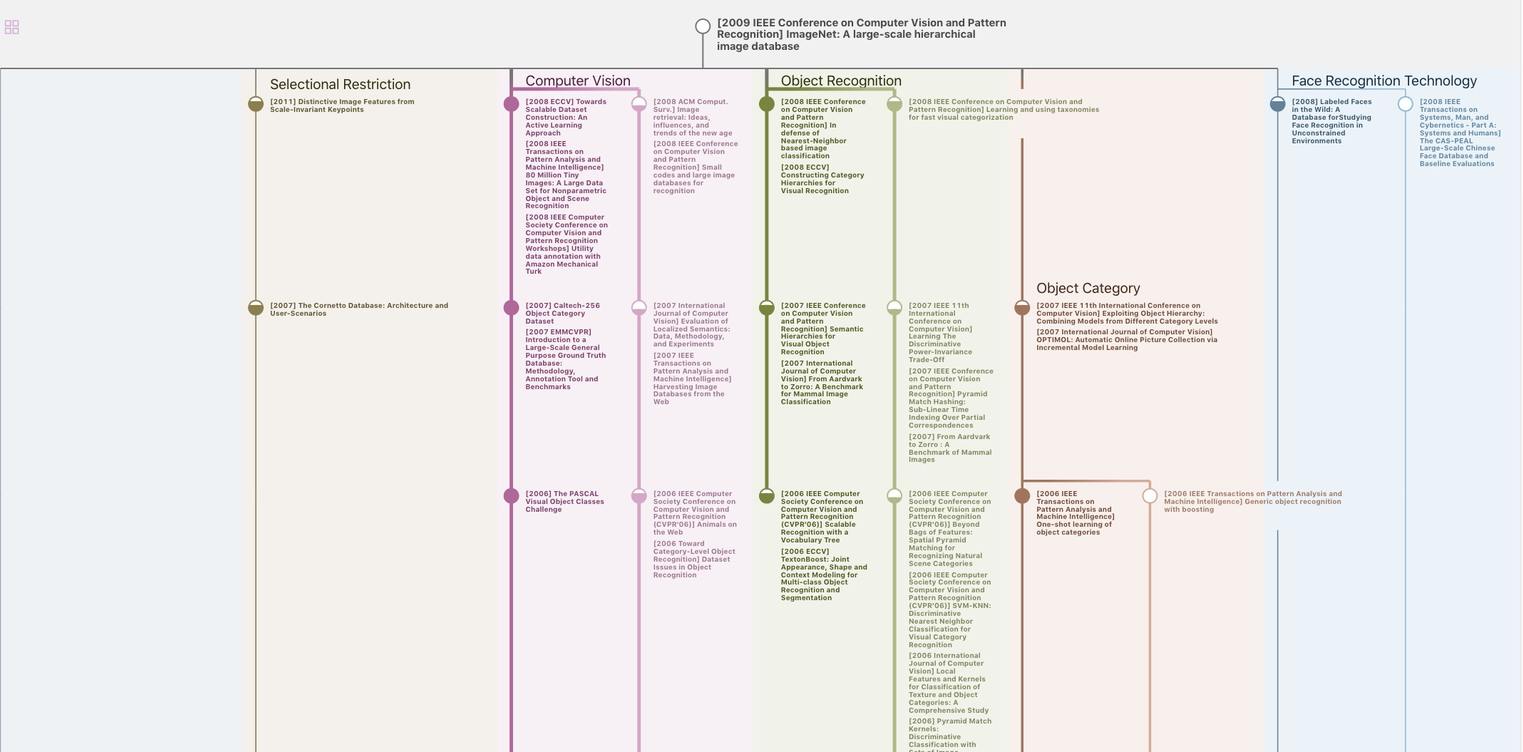
生成溯源树,研究论文发展脉络
Chat Paper
正在生成论文摘要