DeSIRe: Deep Signer-Invariant Representations for Sign Language Recognition
IEEE Transactions on Systems, Man, and Cybernetics: Systems(2021)
摘要
As a key technology to help bridging the gap between deaf and hearing people, sign language recognition (SLR) has become one of the most active research topics in the human-computer interaction field. Although several SLR methodologies have been proposed, the development of a real-world SLR system is still a very challenging task. One of the main challenges is related to the large intersigner variability that exists in the manual signing process of sign languages. To address this problem, we propose a novel end-to-end deep neural network that explicitly models highly discriminative signer-independent latent representations from the input data. The key idea of our model is to learn a distribution over latent representations, conditionally independent of signer identity. Accordingly, the learned latent representations will preserve as much information as possible about the signs, and discard signer-specific traits that are irrelevant for recognition. By imposing such regularization in the representation space, the result is a truly signer-independent model which is robust to different and new test signers. The experimental results demonstrate the effectiveness of the proposed model in several SLR databases.
更多查看译文
关键词
Deep neural networks,generative models,representation learning,signer-independent sign language recognition,variational autoencoders (VAEs)
AI 理解论文
溯源树
样例
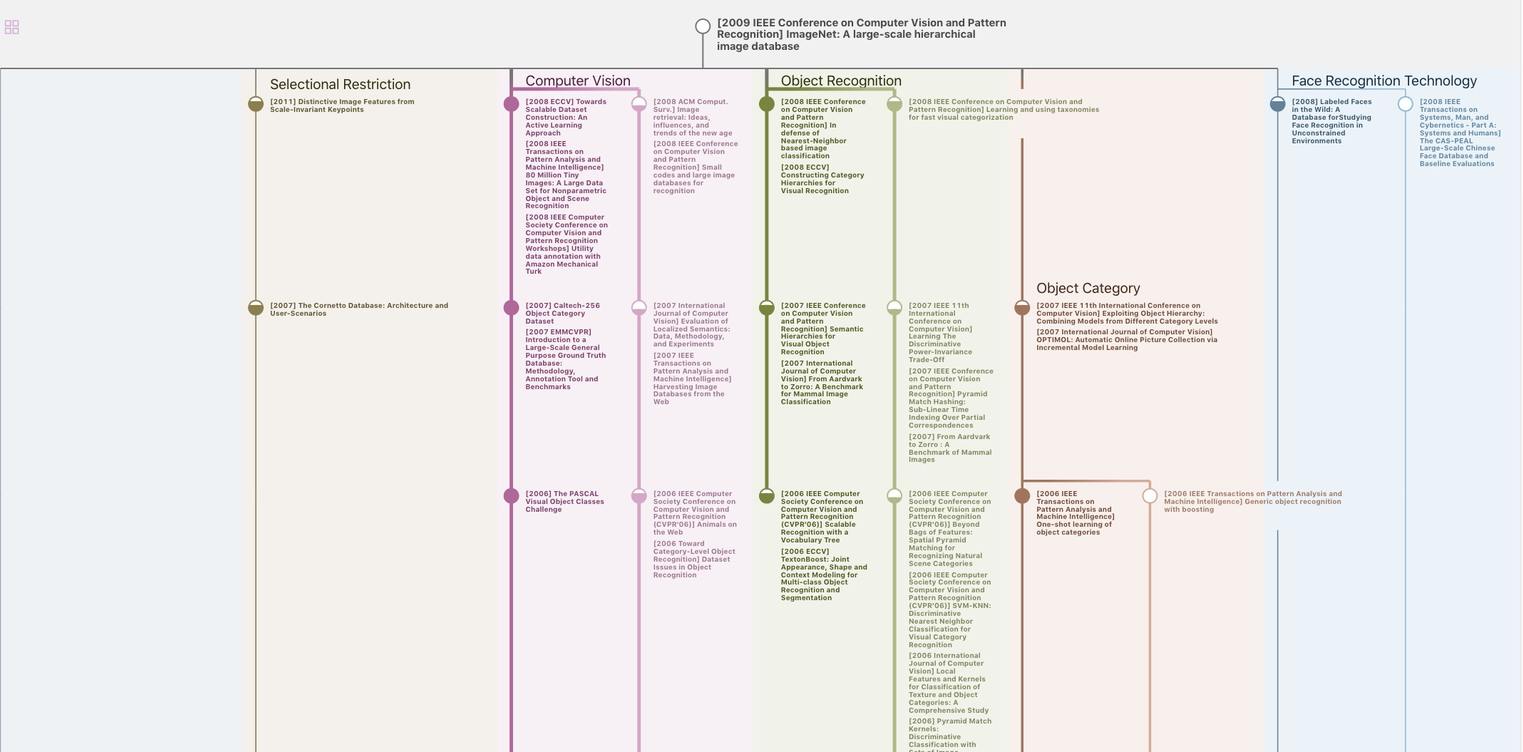
生成溯源树,研究论文发展脉络
Chat Paper
正在生成论文摘要